Estimating System State from the Actions of a Reinforcement Learning Agent
AIAA SCITECH 2023 Forum(2023)
摘要
Interaction between robotic agents typically requires some information about the other systems' state be available to each agent. Whether through direct communication among cooperating agents or the use of tracking systems for uncooperative agents, this information is critical to enable one agent to respond to another. When agents are not cooperating, or when environmental or operational considerations prevent communication, this interaction requires that agents maximize the use of sparse channels of information. One such channel is the actions selected by an agent. When these actions are selected by a stochastic policy function this can allow an estimation problem to be formulated that attempts to determine the state distribution of an agent given observations of its actions. This paper examines this estimation problem, formulates estimators which take advantage of an a priori known policy function, and examines the performance of the estimator in two example applications. The estimator performance is analyzed to identify some considerations in agent and estimator design to enable estimating state from the agent actions.
更多查看译文
关键词
estimating,reinforcement,agent,system,learning
AI 理解论文
溯源树
样例
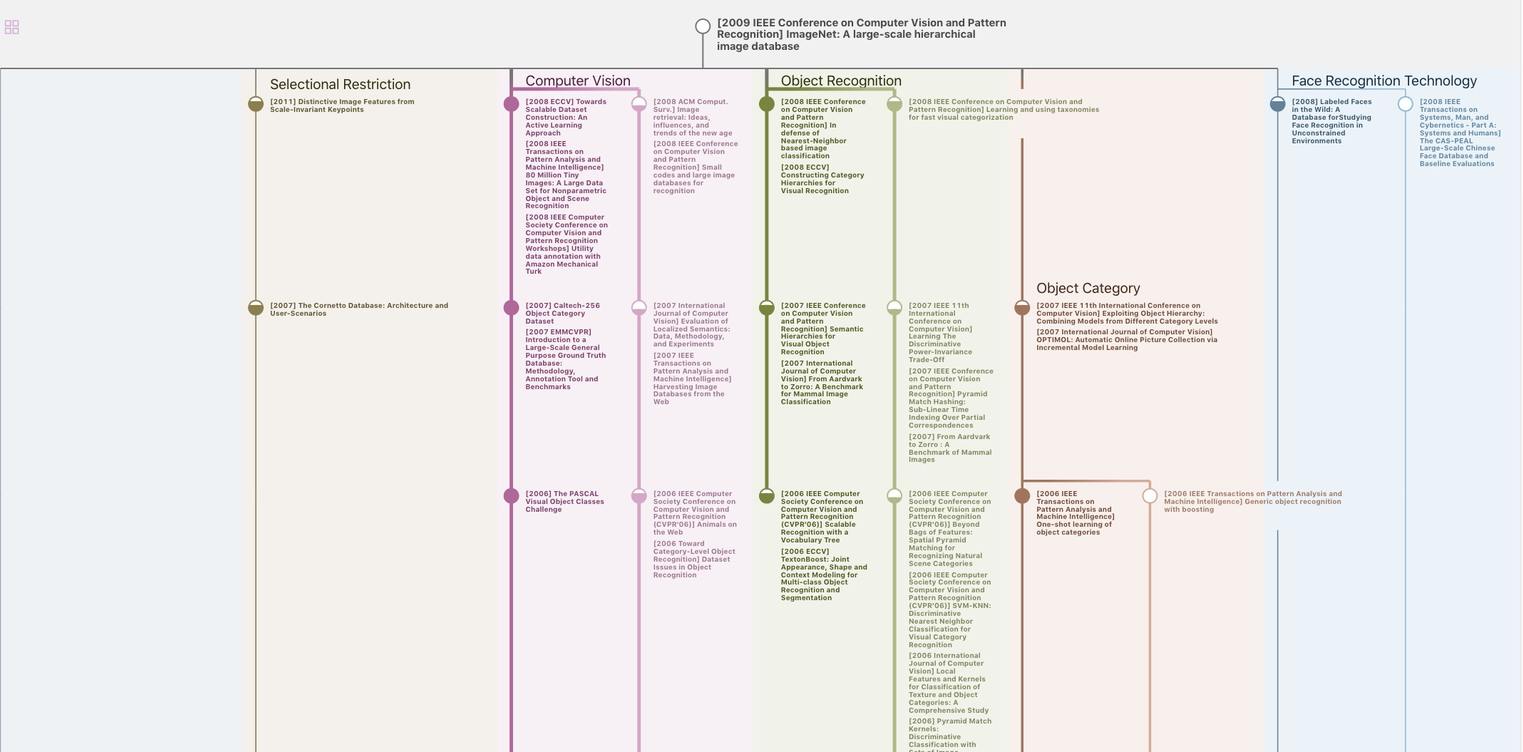
生成溯源树,研究论文发展脉络
Chat Paper
正在生成论文摘要