Detecting pediatric wrist fractures using deep-learning-based object detection
Pediatric radiology(2023)
摘要
Background Missed fractures are the leading cause of diagnostic error in the emergency department, and fractures of pediatric bones, particularly subtle wrist fractures, can be misidentified because of their varying characteristics and responses to injury. Objective This study evaluated the utility of an object detection deep learning framework for classifying pediatric wrist fractures as positive or negative for fracture, including subtle buckle fractures of the distal radius, and evaluated the performance of this algorithm as augmentation to trainee radiograph interpretation. Materials and methods We obtained 395 posteroanterior wrist radiographs from unique pediatric patients (65% positive for fracture, 30% positive for distal radial buckle fracture) and divided them into train ( n = 229), tune ( n = 41) and test ( n = 125) sets. We trained a Faster R-CNN (region-based convolutional neural network) deep learning object-detection model. Two pediatric and two radiology residents evaluated radiographs initially without the artificial intelligence (AI) assistance, and then subsequently with access to the bounding box generated by the Faster R-CNN model. Results The Faster R-CNN model demonstrated an area under the curve (AUC) of 0.92 (95% confidence interval [CI] 0.87–0.97), accuracy of 88% ( n = 110/125; 95% CI 81–93%), sensitivity of 88% ( n = 70/80; 95% CI 78–94%) and specificity of 89% ( n = 40/45, 95% CI 76–96%) in identifying any fracture and identified 90% of buckle fractures ( n = 35/39, 95% CI 76–97%). Access to Faster R-CNN model predictions significantly improved average resident accuracy from 80 to 93% in detecting any fracture ( P < 0.001) and from 69 to 92% in detecting buckle fracture ( P < 0.001). After accessing AI predictions, residents significantly outperformed AI in cases of disagreement (73% resident correct vs. 27% AI, P = 0.002). Conclusion An object-detection-based deep learning approach trained with only a few hundred examples identified radiographs containing pediatric wrist fractures with high accuracy. Access to model predictions significantly improved resident accuracy in diagnosing these fractures.
更多查看译文
关键词
Artificial intelligence,Bone,Buckle fracture,Children,Convolutional neural network,Deep learning,Radiography,Wrist
AI 理解论文
溯源树
样例
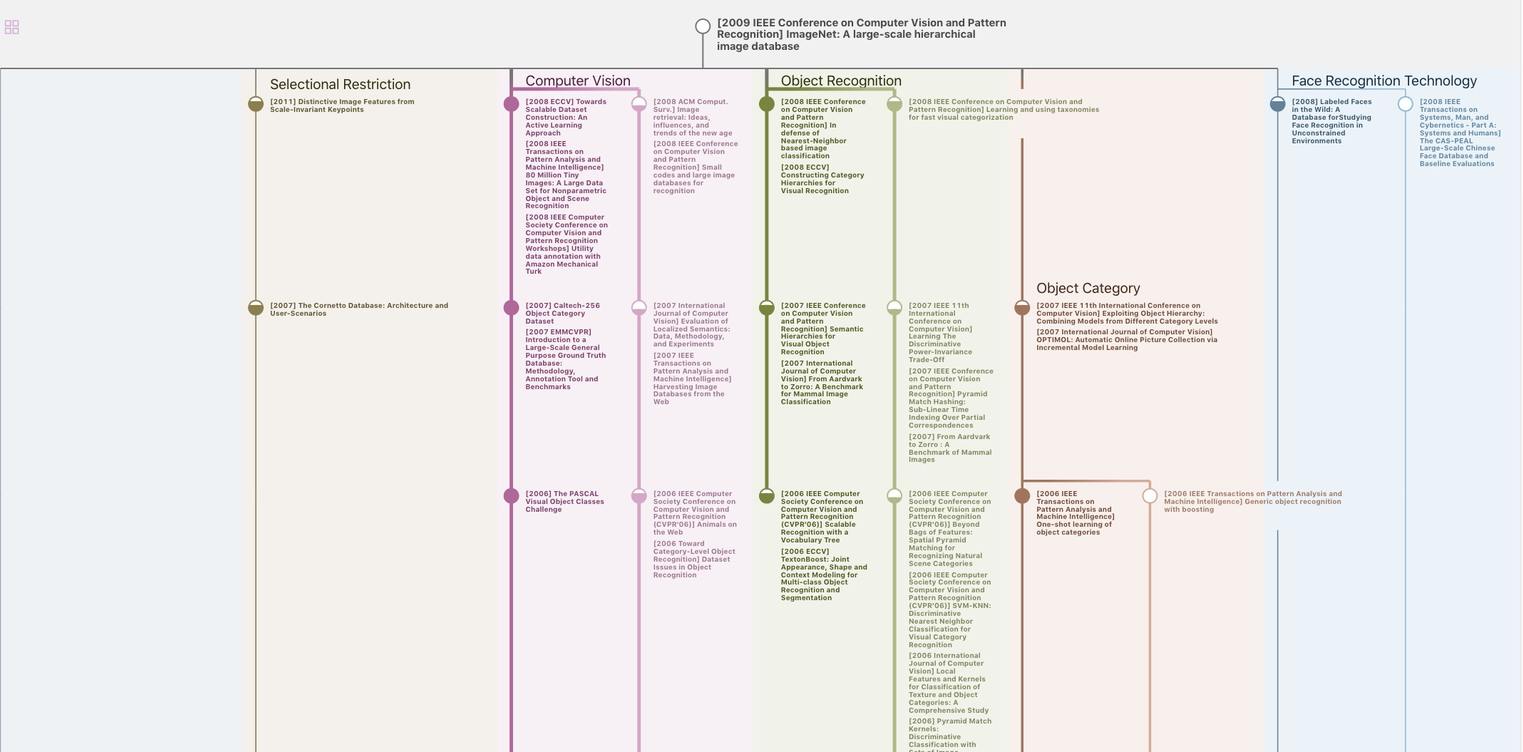
生成溯源树,研究论文发展脉络
Chat Paper
正在生成论文摘要