Statistical Analysis of Tapered Grain Solid Rocket Motor Performance
AIAA SCITECH 2023 Forum(2023)
摘要
This paper investigates applying statistical learning techniques to tapered grain solid rocket motors. Tapered grain geometries offer an alternative to complex cross sections to control the thrust profile of the motor and are common in defense and space launch applications. Lagrangian flow assumptions were used to develop a tool that can robustly model the internal ballistics for tapered grain motors with fidelity suitable for conceptual design applications. Using a lumped parameter burn rate model, the burning area can be modeled to produce thrust vs. time curves with high computational efficiency. Once validated, the solid rocket motor internal ballistics tool was used to generate large databases practical for statistical learning applications. In this paper, we use statistical learning techniques for predicting thrust profiles based on the provided geometries. Tapered right circularly perforated star grain families are included in the paper. This paper includes a discussion of the physics equations governing the internal ballistics tool, the validation cases for the tapered solid rocket motor tool, the statistical learning approaches, and the accompanying results.
更多查看译文
关键词
motor,rocket,performance,grain
AI 理解论文
溯源树
样例
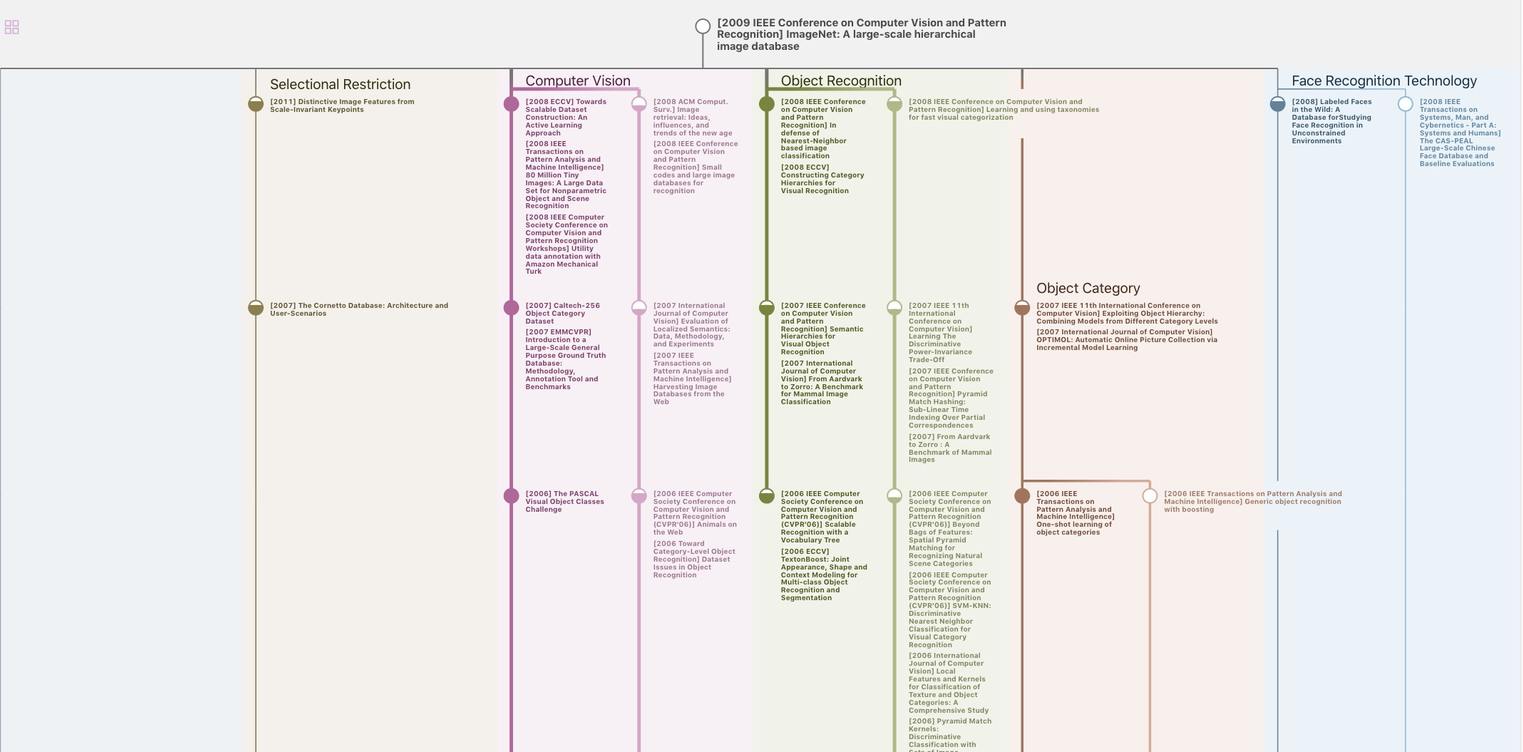
生成溯源树,研究论文发展脉络
Chat Paper
正在生成论文摘要