Abundance Matrix Correlation Analysis Network Based on Hierarchical Multihead Self-Cross-Hybrid Attention for Hyperspectral Change Detection
IEEE Transactions on Geoscience and Remote Sensing(2023)
摘要
Hyperspectral image (HSI) change detection is a technique for detecting the changes between the multitemporal HSIs of the same scene. Many existing change detection methods have achieved good results, but there still exist problems as follows: 1) mixed pixels exist in HSI due to the low spatial resolution of hyperspectral sensor and other external interference and 2) many existing deep learning-based networks cannot make full use of the correlation difference information between the bitemporal images. These problems are not conducive to further improving the accuracy of change detection. In this article, we propose an abundance matrix correlation analysis network based on hierarchical multihead self-cross-hybrid attention (AMCAN-HMSchA) for HSI change detection, which hierarchically highlights the correlation difference information at the subpixel level to detect the subtle changes. The endmember sharing-based abundance matrix learning module (AMLM) maps the changed information between bitemporal HSIs to the corresponding abundance matrices. The hierarchical MSchA extracts the enhanced difference features by constantly comparing the self-correlation with cross correlation between the abundance matrices of the HSIs. Then, the difference features are concatenated and fed into the fully connected layers to obtain the change map. Experiments on three widely used datasets show that the proposed method has superior performance compared with other state-of-the-art methods.
更多查看译文
关键词
Abundance matrix,change detection,correlation difference information,hyperspectral image (HSI)
AI 理解论文
溯源树
样例
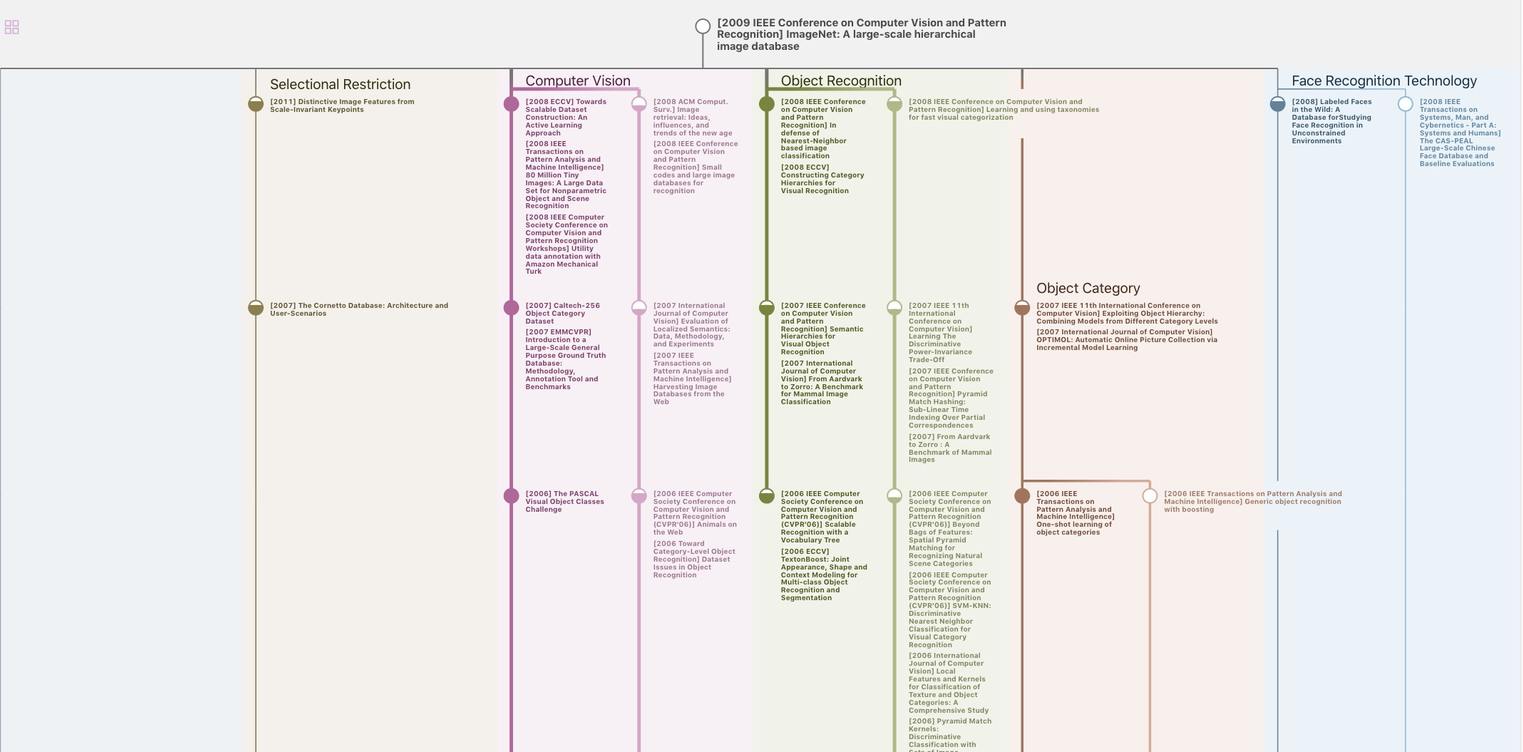
生成溯源树,研究论文发展脉络
Chat Paper
正在生成论文摘要