Incorporating BERT With Probability-Aware Gate for Spoken Language Understanding
IEEE/ACM Transactions on Audio, Speech, and Language Processing(2023)
Abstract
Spoken language understanding (SLU) is an essential part of a task-oriented dialogue system, which mainly includes intent detection and slot filling. Some existing approaches obtain enhanced semantic representation by establishing the correlation between two tasks. However, those methods show little improvement when applied to BERT, since BERT has learned rich semantic features. In this paper, we propose a BERT-based model with the probability-aware gate mechanism, called PAGM (Probability Aware Gated Model). PAGM aims to learn the correlation between intent and slot from the perspective of probability distribution, which explicitly utilizes intent information to guide slot filling. Besides, in order to efficiently incorporate BERT with the probability-aware gate, we design the stacked fine-tuning strategy. This approach introduces a mid-stage before target model training, which enables BERT to get better initialization for final training. Experiments show that PAGM achieves significant improvement on two benchmark datasets, and outperforms the previous state-of-the-art results.
MoreTranslated text
Key words
Training,Correlation,Bit error rate,Semantics,Natural languages,Logic gates,Filling,Natural language processing,spoken language understanding,intent detection,slot filling
AI Read Science
Must-Reading Tree
Example
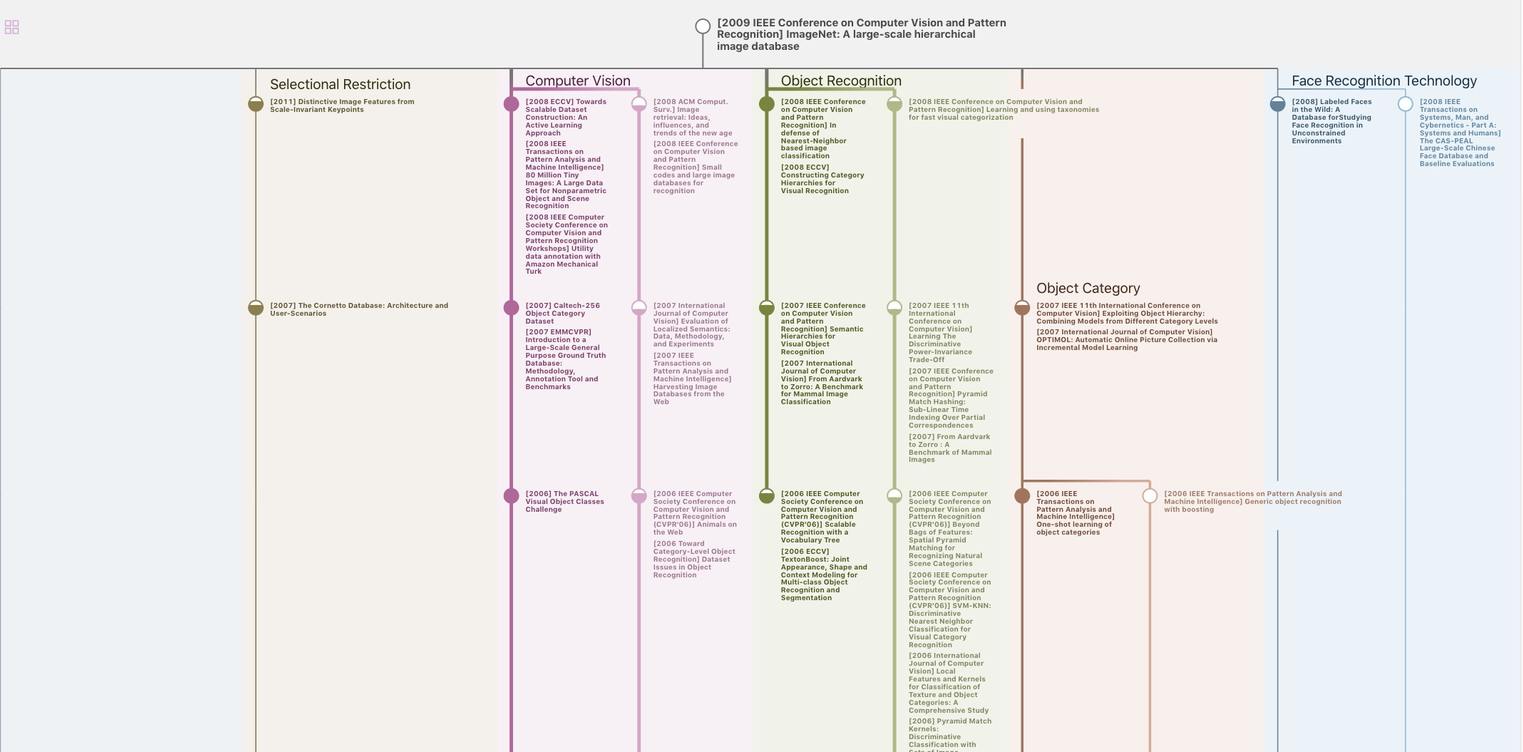
Generate MRT to find the research sequence of this paper
Chat Paper
Summary is being generated by the instructions you defined