Quantized Neural Network Synthesis for Direct Logic Circuit Implementation
IEEE Transactions on Computer-Aided Design of Integrated Circuits and Systems(2023)
摘要
Hardware acceleration enables neural network (NN) inferencing on edge devices and for high throughput applications. Most approaches use neural processing elements for computation while storing weights in memory blocks. To avoid costly memory access, recent efforts seek direct logic implementation with weights hardwired into the circuit. However, special training strategies are often needed, and they could not maintain accuracy. In contrast, we take a trained and quantized NN as input and synthesize it by Booth encoding and logic sharing, resulting in a hardware accelerator without degrading accuracy. Experiments demonstrate that our method outperforms existing work in area reduction and/or throughput and power efficiency.
更多查看译文
关键词
AI accelerators,circuit synthesis,field programmable gate arrays,neural network (NN) hardware
AI 理解论文
溯源树
样例
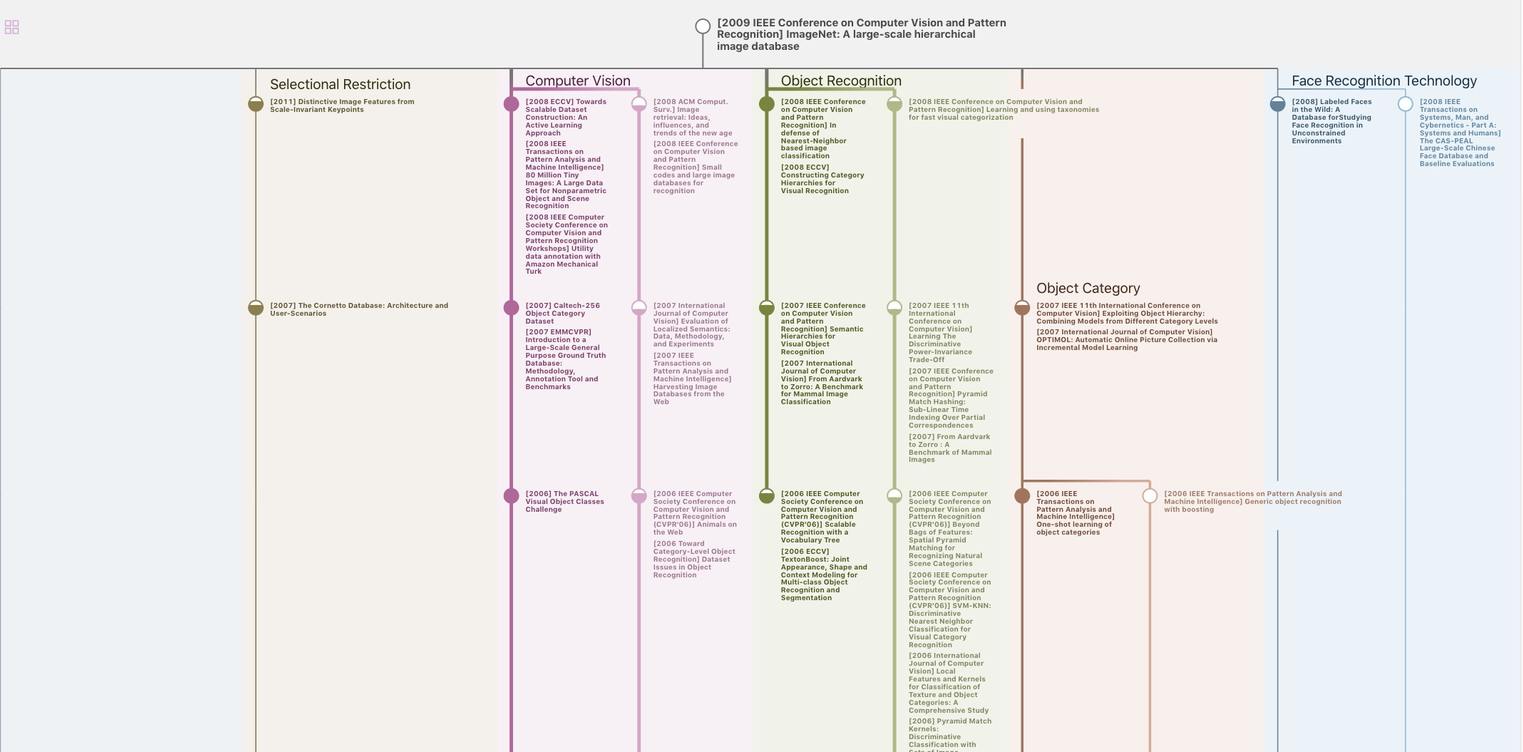
生成溯源树,研究论文发展脉络
Chat Paper
正在生成论文摘要