Predicting Multi-Period Corporate Default Based on Bayesian Estimation of Forward Intensity - Evidence from China.
Systems(2023)
摘要
We employed a forward intensity approach to predict the multi-period defaults of Chinese-listed firms during the period 2001-2019 on a monthly basis. We introduced the firm's default heterogeneity into the model, and each firm's actual past default situation was considered for Bayesian estimation. Maximum pseudo-likelihood estimation was conducted on 3513 firms to calculate the parameters of the Bayesian model to adjust the default intensity of all 4216 firms. Finally, we re-calculated the default probabilities and compared them with the original default probabilities of the out-of-sample 703 firms for all prediction horizons. We found that the Bayesian model, considering the firm's default heterogeneity, improved the prediction accuracy ratio of the out-of-sample firm's default probabilities both for short and long horizons. As compared with the original model, the prediction accuracy ratio of the out-of-sample's default probabilities, which were computed by our model, increased by almost 15% for horizons from 1 month to 6 months. When the horizon was extended from 1 year to 3 years, the prediction accuracy ratio increased by more than 10%. We found that the Bayesian model improved the predictive performance of the forward intensity model, which is helpful to improve the credit risk measurement system of Chinese-listed firms.
更多查看译文
关键词
default,Bayesian estimation,heterogeneity,forward intensity,credit risk measurement system
AI 理解论文
溯源树
样例
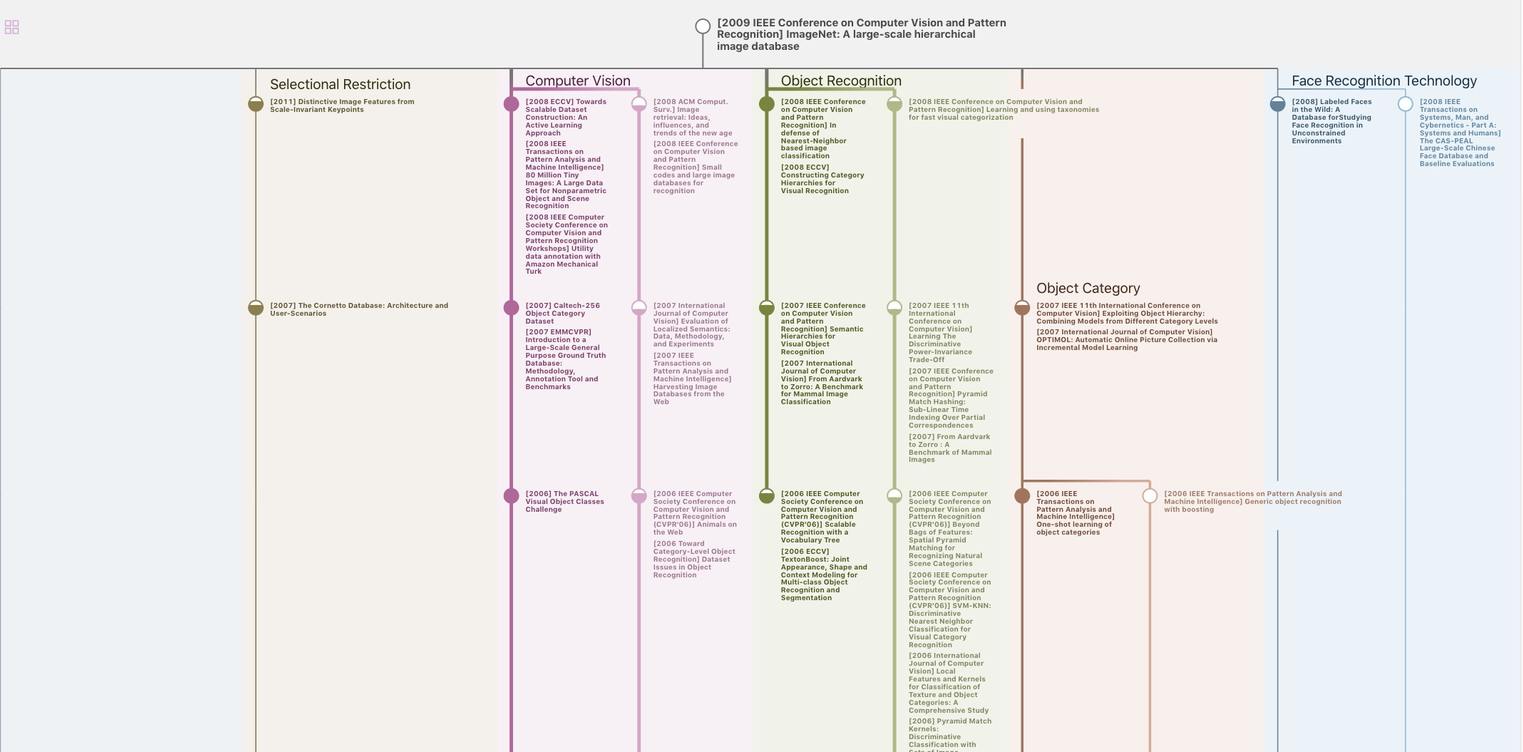
生成溯源树,研究论文发展脉络
Chat Paper
正在生成论文摘要