A Distributed Machine Learning-Based Approach for IRS-Enhanced Cell-Free MIMO Networks
arxiv(2023)
摘要
In cell-free multiple input multiple output (MIMO) networks, multiple base stations (BSs) collaborate to achieve high spectral efficiency. Nevertheless, high penetration loss due to large blockages in harsh propagation environments is often an issue that severely degrades communication performance. Considering that intelligent reflecting surface (IRS) is capable of constructing digitally controllable reflection links in a low-cost manner, we investigate an IRS-enhanced downlink cell-free MIMO network in this paper. We aim to maximize the sum rate of all the users by jointly optimizing the transmit beamforming at the BSs and the reflection coefficients at the IRS. To address the optimization problem, we propose a fully distributed machine learning algorithm. Different from the conventional iterative optimization algorithms that require a central processing at the central processing unit (CPU) and large amount of channel state information and signaling exchange between the BSs and the CPU, in the proposed algorithm, each BS can locally design its beamforming vectors. Meanwhile, the IRS reflection coefficients are determined by one of the BSs. Simulation results show that the deployment of IRS can significantly boost the sum user rate and that the proposed algorithm can achieve a high sum user rate with a low computational complexity.
更多查看译文
关键词
Intelligent reflecting surface,cell-free MIMO,beamforming,distributed machine learning
AI 理解论文
溯源树
样例
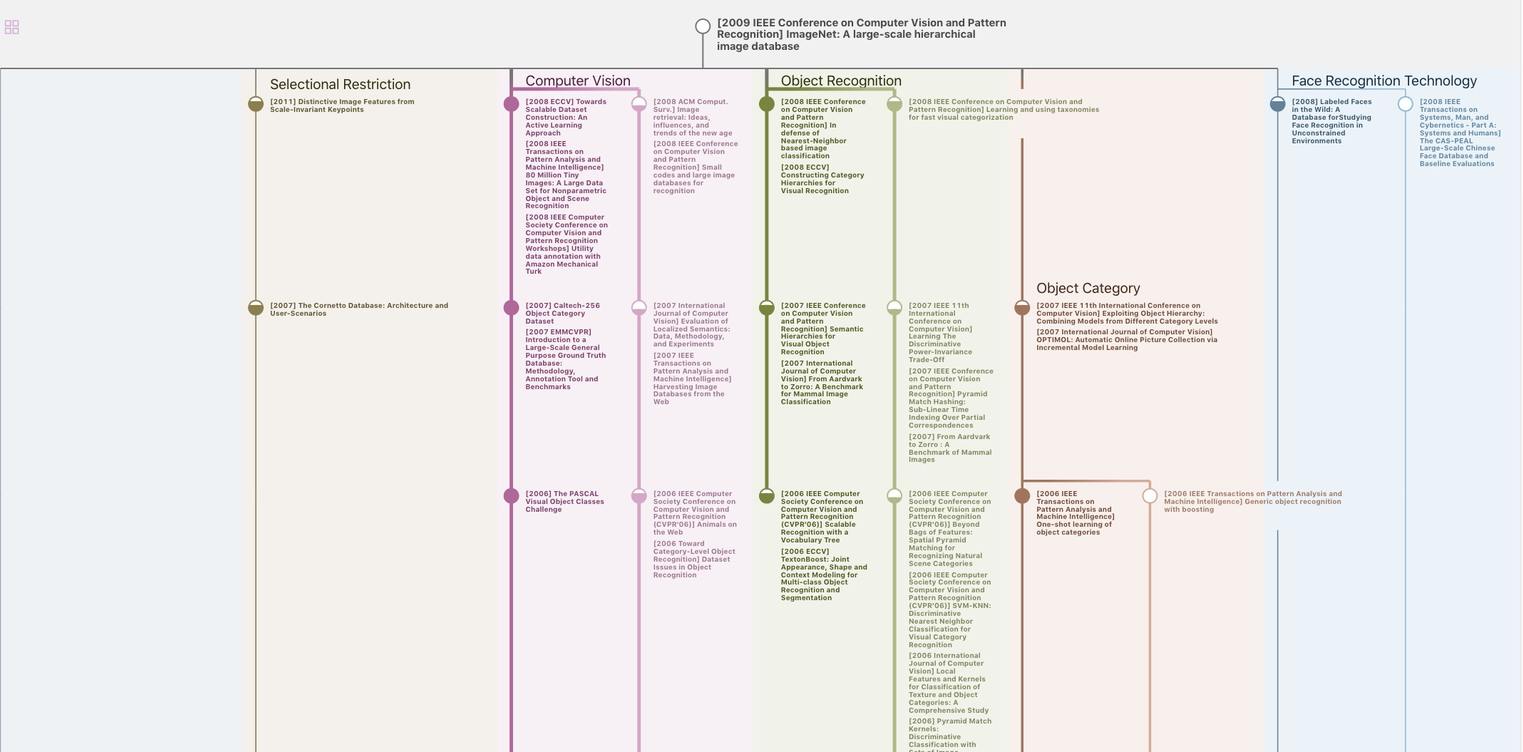
生成溯源树,研究论文发展脉络
Chat Paper
正在生成论文摘要