Deep Multi-Look Sequence Processing for Synthetic Aperture Sonar Image Segmentation
IEEE Transactions on Geoscience and Remote Sensing(2023)
摘要
Deep learning has enabled significant improvements in semantic image segmentation, especially in underwater imaging domains such as side scan sonar (SSS). In this work, we apply deep learning to synthetic aperture sonar (SAS) imagery, which has an advantage over traditional SSS in which SAS produces coherent high- and constant-resolution imagery. Despite the successes of deep learning, one drawback is the need for abundant labeled training data to enable success. Such abundant labeled data are not always available as in the case of SAS where collections are expensive and obtaining quality ground-truth labels may require diver intervention. To overcome these challenges, we propose a domain-specific deep learning network architecture utilizing a unique property to complex-valued SAS imagery: the ability to resolve angle-of-arrival (AoA) of acoustic returns through
$k$
-space processing. By sweeping through consecutive incrementally advanced AoA bandpass filters (a process sometimes referred to as multi-look processing), this technique generates a sequence of images emphasizing angle-dependent seafloor scattering and motion from biologics along the seafloor or in the water column. Our proposal, which we call multi-look sequence processing network (MLSP-Net), is a domain-enriched deep neural network architecture that models the multi-look image sequence using a recurrent neural network (RNN) to extract robust features suitable for semantic segmentation of the seafloor without the need for abundant training data. Unlike previous segmentation works in SAS, our model ingests a complex-valued SAS image and affords the ability to learn the AoA filters in
$k$
-space as part of the training procedure. We show the results on a challenging real-world SAS database, and despite the lack of abundant training data, our proposed method shows superior results over state-of-the-art techniques.
更多查看译文
关键词
Deep learning,image segmentation,recurrent neural networks (RNNs),synthetic aperture sonar (SAS)
AI 理解论文
溯源树
样例
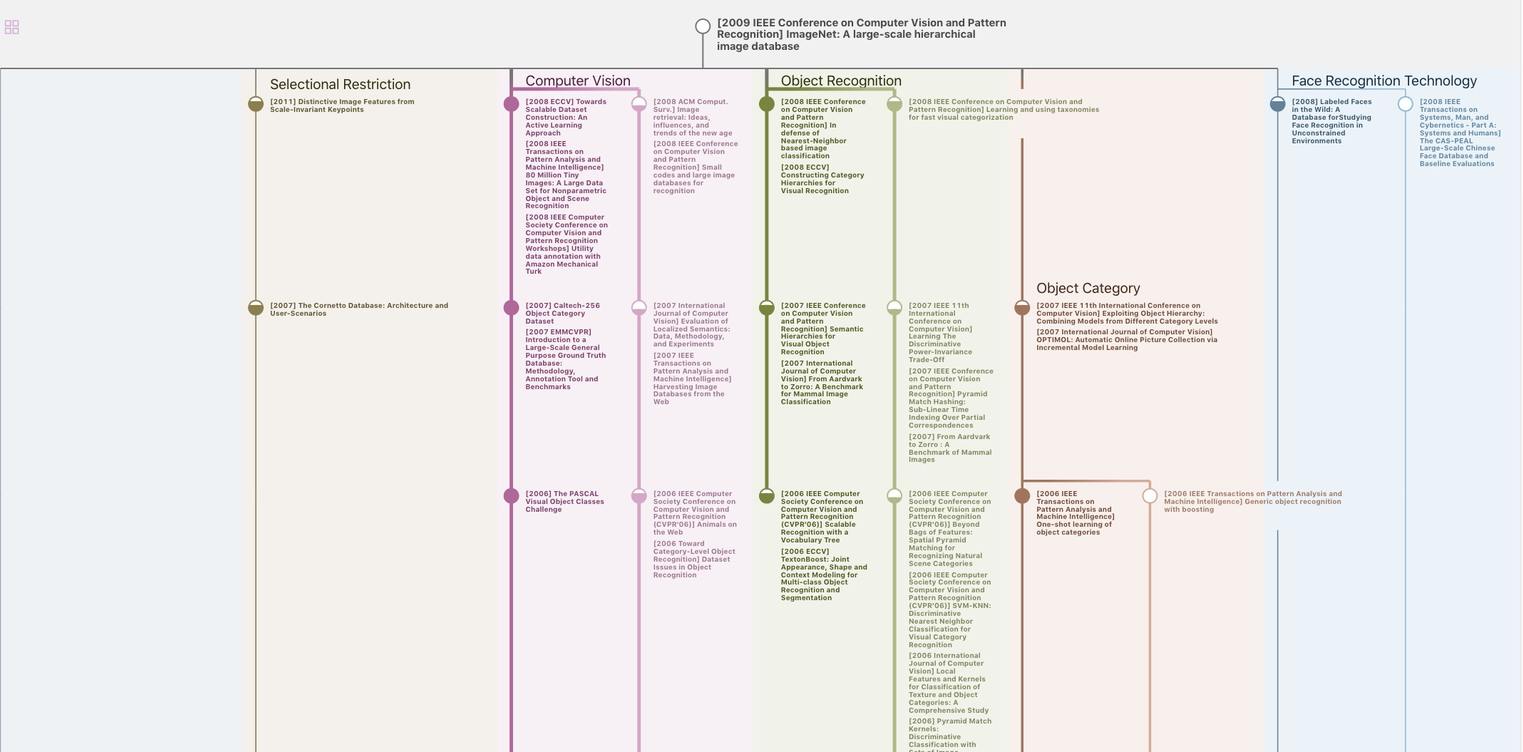
生成溯源树,研究论文发展脉络
Chat Paper
正在生成论文摘要