Query by Example in Remote Sensing Image Archive Using Enhanced Deep Support Vector Data Description
IEEE Journal of Selected Topics in Applied Earth Observations and Remote Sensing(2023)
Abstract
This article studies remote sensing image retrieval using kernel-based support vector data description (SVDD). We exploit deep SVDD, which is a well-known method for one-class classification to recover the most relevant samples from the archive. To this end, a deep neural network (DNN) is jointly trained to map the data into a hypersphere of minimum volume in the latent space. It is expected that similar samples to the query are compressed inside of the hypersphere. The closest embedding to the center of the hypersphere is related to the most relevant sample to query. We enhance deep SVDD by injecting the statistical information of data to the DNN by means of additional terms in the cost function. The first enhancement method takes advantage of covariance regularization of batches of the training set to penalize unnecessary redundancy and minimize the correlation between the different dimensions of the embedding. The second method involves unlocking the hypersphere's predefined center while preventing network divergence during training. Therefore, two parameters are designed to control the importance of the drifting of the center and the importance of a fixed predefined center (convergence), respectively. This has been implemented by considering the average of batches of embedding in each iteration as the updated center. This pushes irrelevant samples away from query samples, making data clustering easier for the DNN. The performance of the proposed methods is evaluated on benchmark datasets.
MoreTranslated text
Key words
Deep neural network (DNN),deep support vector data description (SVDD),EuroSAT,one-class classification,query by example in earth observation,remote sensing (RS) image retrieval
AI Read Science
Must-Reading Tree
Example
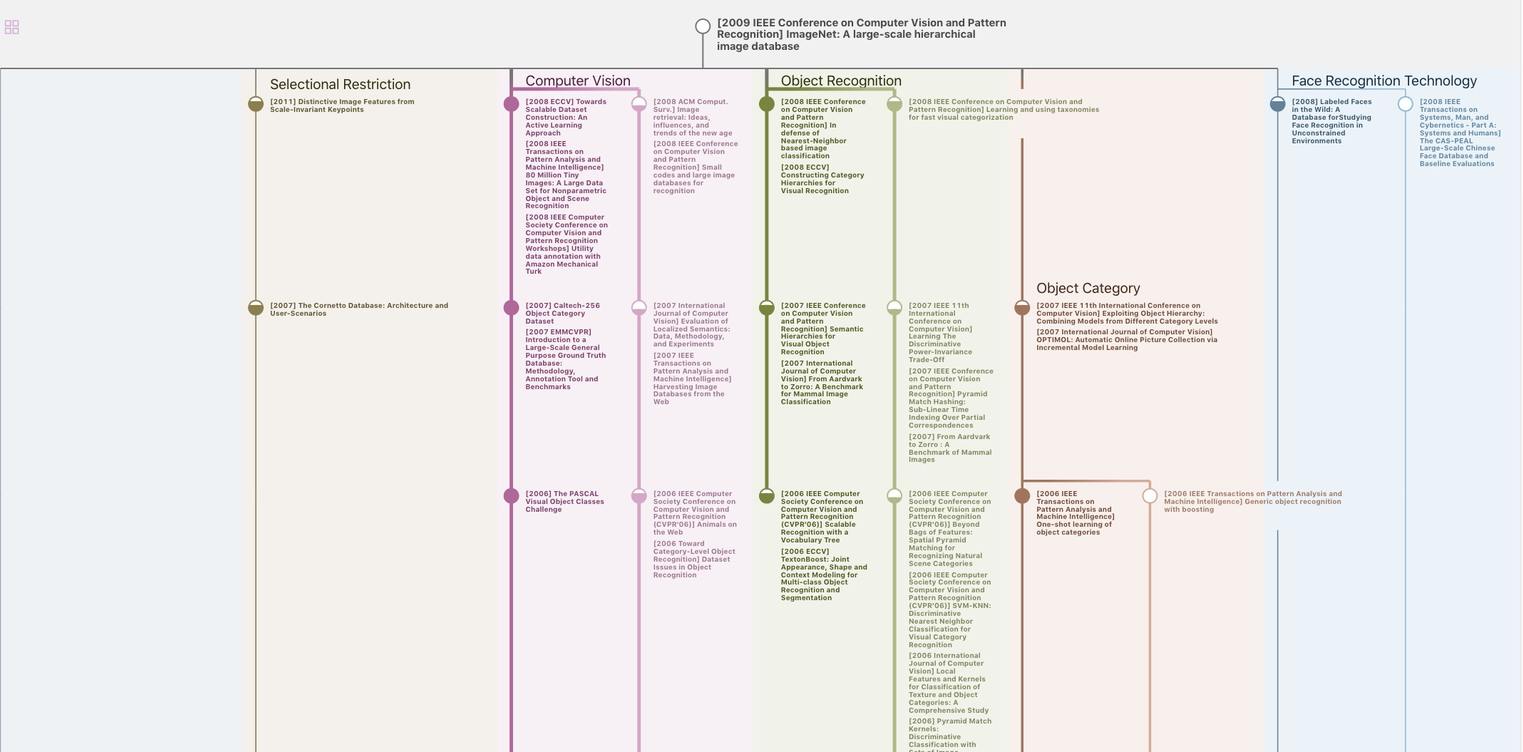
Generate MRT to find the research sequence of this paper
Chat Paper
Summary is being generated by the instructions you defined