Strong inductive biases provably prevent harmless interpolation
ICLR 2023(2023)
摘要
Classical wisdom suggests that estimators should avoid fitting noise to achieve good generalization. In contrast, modern overparameterized models can yield small test error despite interpolating noise -- a phenomenon often called "benign overfitting" or "harmless interpolation". This paper argues that the degree to which interpolation is harmless hinges upon the strength of an estimator's inductive bias, i.e., how heavily the estimator favors solutions with a certain structure: while strong inductive biases prevent harmless interpolation, weak inductive biases can even require fitting noise to generalize well. Our main theoretical result establishes tight non-asymptotic bounds for high-dimensional kernel regression that reflect this phenomenon for convolutional kernels, where the filter size regulates the strength of the inductive bias. We further provide empirical evidence of the same behavior for deep neural networks with varying filter sizes and rotational invariance.
更多查看译文
关键词
high-dimensional statistics,non-parametric regression,deep learning theory,generalization bounds,benign overfitting
AI 理解论文
溯源树
样例
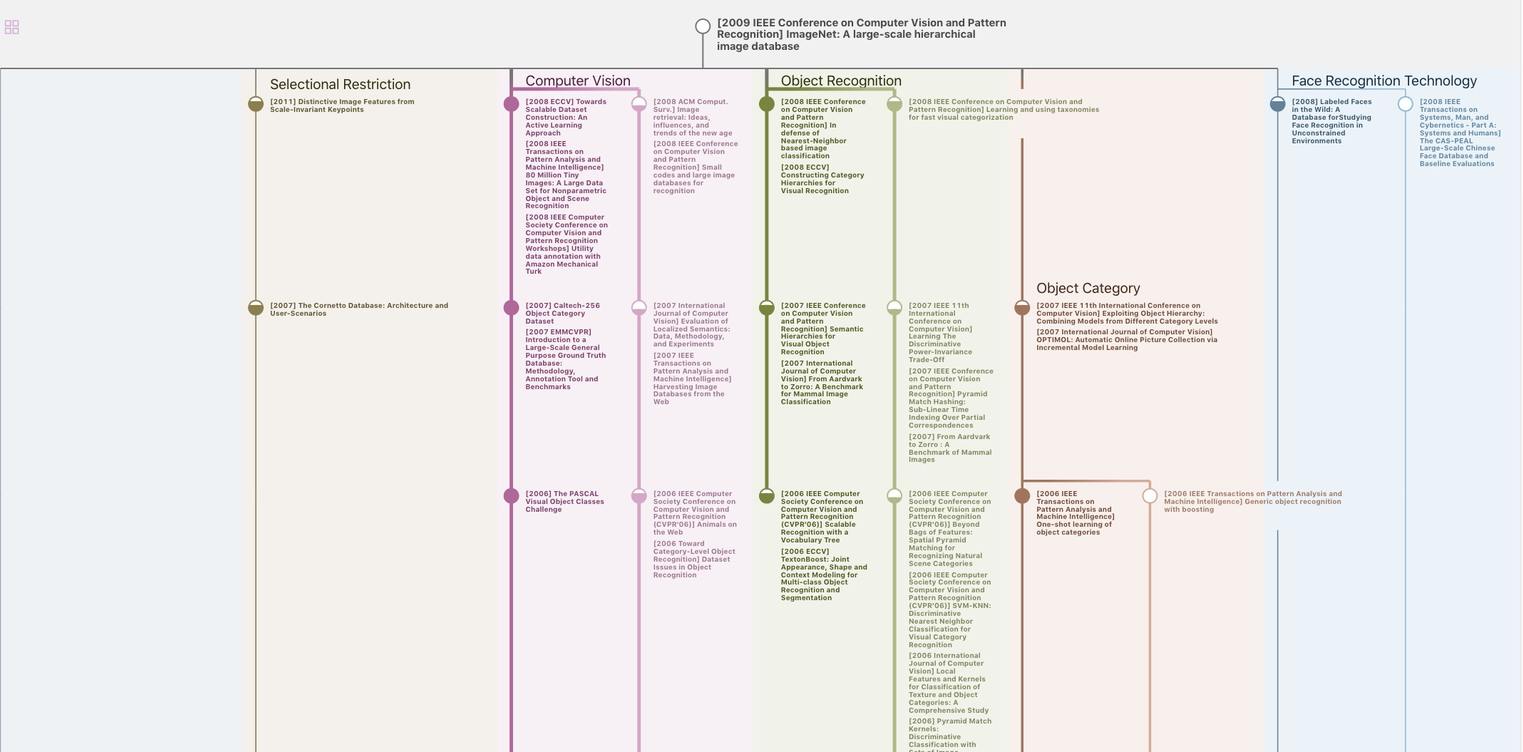
生成溯源树,研究论文发展脉络
Chat Paper
正在生成论文摘要