Deep Learning Enables Reduced Gadolinium Dose for Contrast-Enhanced Blood-Brain Barrier Opening
arxiv(2023)
摘要
Focused ultrasound (FUS) can be used to open the blood-brain barrier (BBB), and MRI with contrast agents can detect that opening. However, repeated use of gadolinium-based contrast agents (GBCAs) presents safety concerns to patients. This study is the first to propose the idea of modeling a volume transfer constant (Ktrans) through deep learning to reduce the dosage of contrast agents. The goal of the study is not only to reconstruct artificial intelligence (AI) derived Ktrans images but to also enhance the intensity with low dosage contrast agent T1 weighted MRI scans. We successfully validated this idea through a previous state-of-the-art temporal network algorithm, which focused on extracting time domain features at the voxel level. Then we used a Spatiotemporal Network (ST-Net), composed of a spatiotemporal convolutional neural network (CNN)-based deep learning architecture with the addition of a three-dimensional CNN encoder, to improve the model performance. We tested the ST-Net model on ten datasets of FUS-induced BBB-openings aquired from different sides of the mouse brain. ST-Net successfully detected and enhanced BBB-opening signals without sacrificing spatial domain information. ST-Net was shown to be a promising method of reducing the need of contrast agents for modeling BBB-opening K-trans maps from time-series Dynamic Contrast-Enhanced Magnetic Resonance Imaging (DCE-MRI) scans.
更多查看译文
关键词
reduced gadolinium dose,contrast-enhanced,blood-brain
AI 理解论文
溯源树
样例
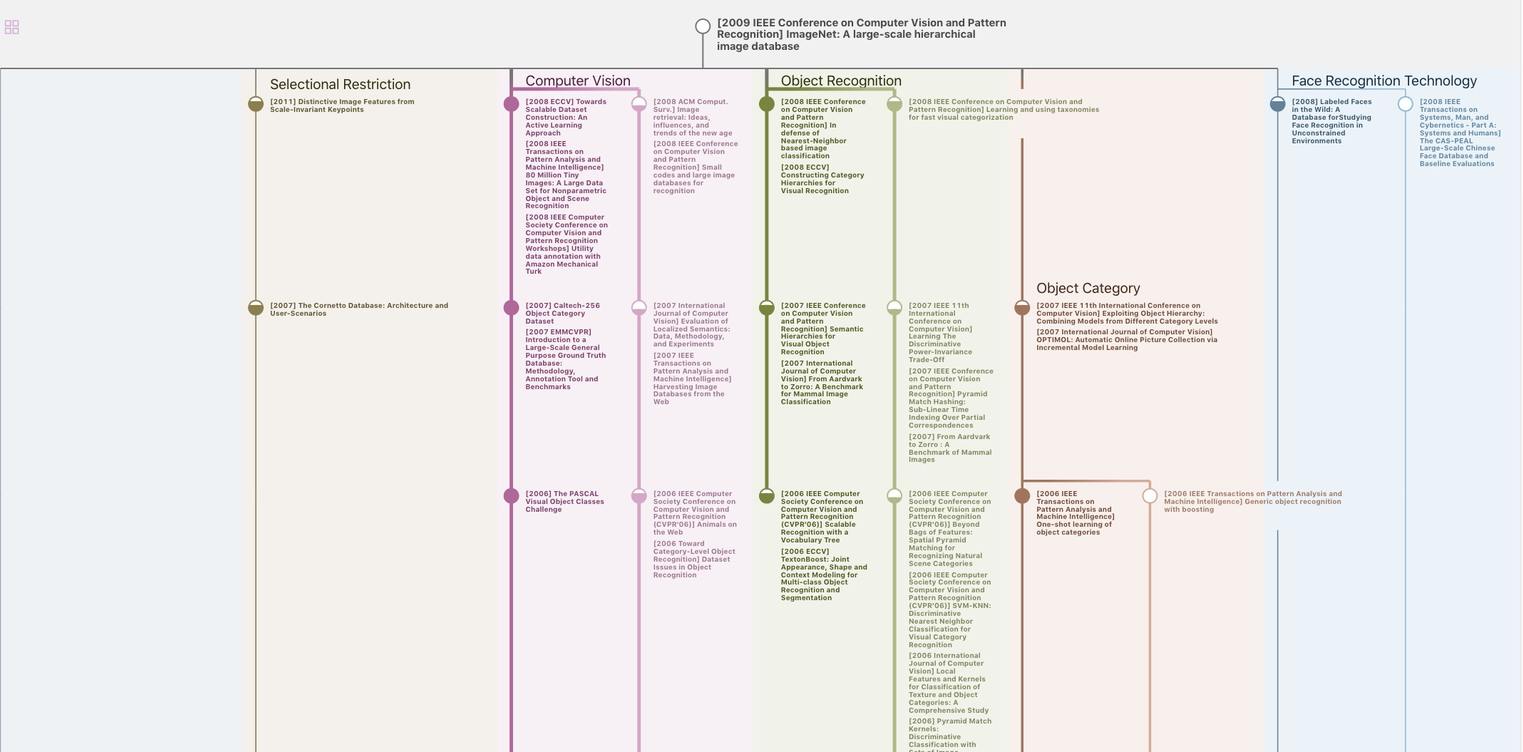
生成溯源树,研究论文发展脉络
Chat Paper
正在生成论文摘要