Multiple imputation for propensity score analysis with covariates missing at random: some clarity on within and across methods
arxiv(2023)
摘要
In epidemiology and social sciences, propensity score methods are popular for estimating treatment effects using observational data, and multiple imputation is popular for handling covariate missingness. However, how to appropriately use multiple imputation for propensity score analysis is not completely clear. This paper aims to bring clarity on the consistency (or lack thereof) of methods that have been proposed, focusing on the within approach (where the effect is estimated separately in each imputed dataset and then the multiple estimates are combined) and the across approach (where typically propensity scores are averaged across imputed datasets before being used for effect estimation). We show that the within method is valid and can be used with any causal effect estimator that is consistent in the full-data setting. Existing across methods are inconsistent, but a different across method that averages the inverse probability weights across imputed datasets is consistent for propensity score weighting. We also comment on methods that rely on imputing a function of the missing covariate rather than the covariate itself, including imputation of the propensity score and of the probability weight. Based on consistency results and practical flexibility, we recommend generally using the standard within method. Throughout, we provide intuition to make the results meaningful to the broad audience of applied researchers.
更多查看译文
关键词
propensity score analysis,multiple imputation,covariates
AI 理解论文
溯源树
样例
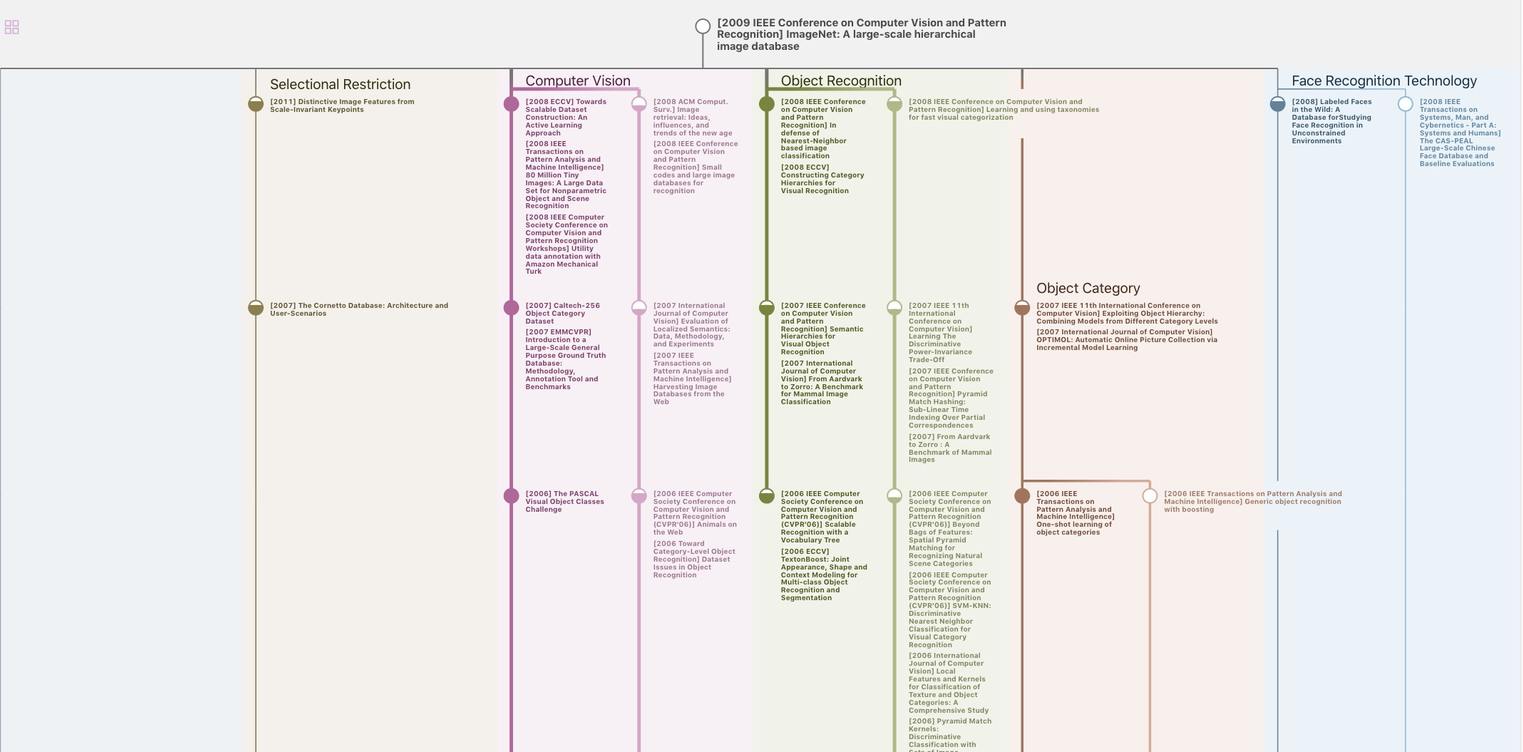
生成溯源树,研究论文发展脉络
Chat Paper
正在生成论文摘要