Negative flux aggregation to estimate feature attributions
CoRR(2023)
摘要
There are increasing demands for understanding deep neural networks' (DNNs) behavior spurred by growing security and/or transparency concerns. Due to multi-layer nonlinearity of the deep neural network architectures, explaining DNN predictions still remains as an open problem, preventing us from gaining a deeper understanding of the mechanisms. To enhance the explainability of DNNs, we estimate the input feature's attributions to the prediction task using divergence and flux. Inspired by the divergence theorem in vector analysis, we develop a novel Negative Flux Aggregation (Ne-FLAG) formulation and an efficient approximation algorithm to estimate attribution map. Unlike the previous techniques, ours doesn't rely on fitting a surrogate model nor need any path integration of gradients. Both qualitative and quantitative experiments demonstrate a superior performance of Ne-FLAG in generating more faithful attribution maps than the competing methods. Our code is available at https://github.com/xinli0928/NeFLAG.
更多查看译文
关键词
negative flux aggregation,feature
AI 理解论文
溯源树
样例
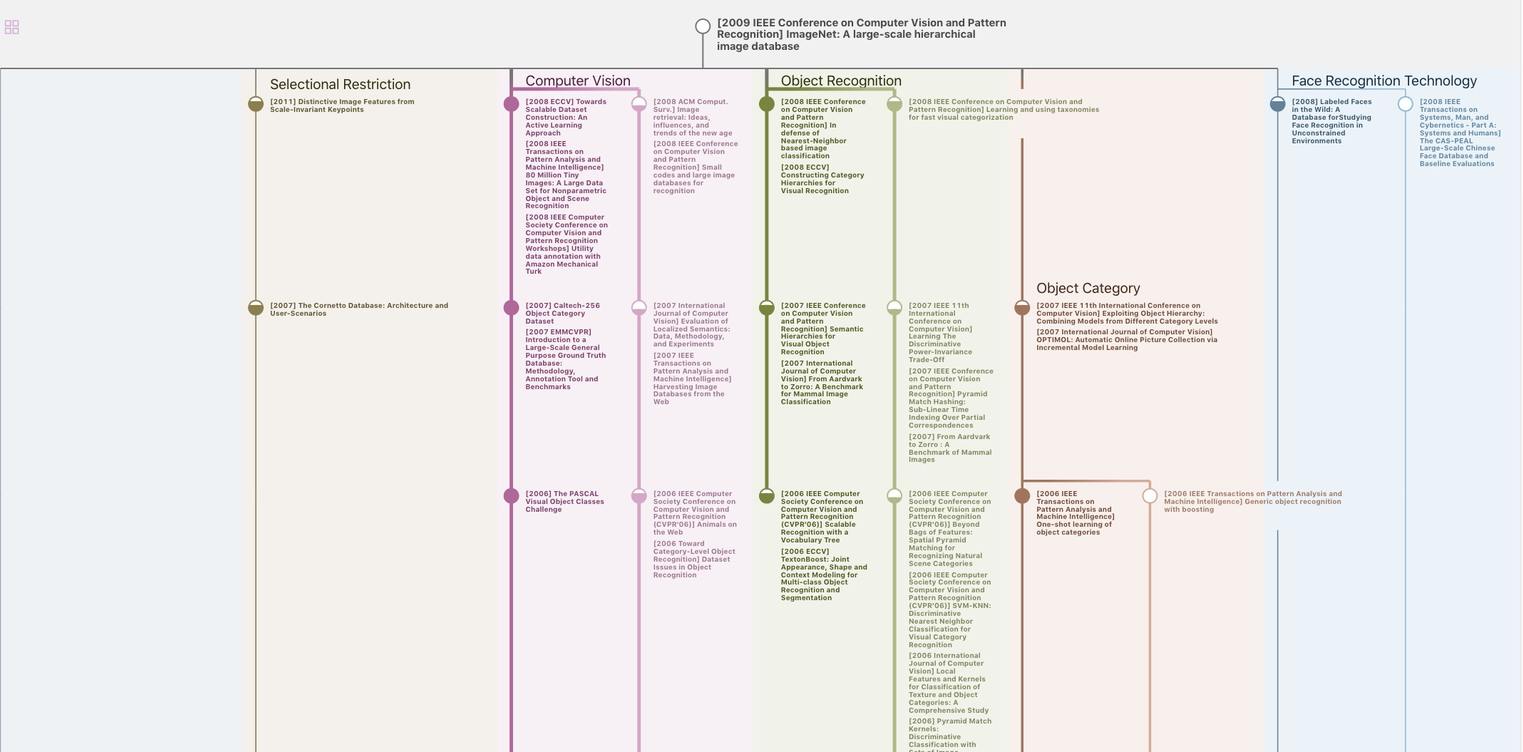
生成溯源树,研究论文发展脉络
Chat Paper
正在生成论文摘要