Ranking with submodular functions on the fly
arxiv(2023)
摘要
Maximizing submodular functions have been studied extensively for a wide range of subset-selection problems. However, much less attention has been given to the role of submodularity in sequence-selection and ranking problems. A recently-introduced framework, named \emph{maximum submodular ranking} (MSR), tackles a family of ranking problems that arise naturally when resources are shared among multiple demands with different budgets. For example, the MSR framework can be used to rank web pages for multiple user intents. In this paper, we extend the MSR framework in the streaming setting. In particular, we consider two different streaming models and we propose practical approximation algorithms. In the first streaming model, called \emph{function arriving}, we assume that submodular functions (demands) arrive continuously in a stream, while in the second model, called \emph{item arriving}, we assume that items (resources) arrive continuously. Furthermore, we study the MSR problem with additional constraints on the output sequence, such as a matroid constraint that can ensure fair exposure among items from different groups. These extensions significantly broaden the range of problems that can be captured by the MSR framework. On the practical side, we develop several novel applications based on the MSR formulation, and empirically evaluate the performance of the proposed~methods.
更多查看译文
关键词
submodular functions
AI 理解论文
溯源树
样例
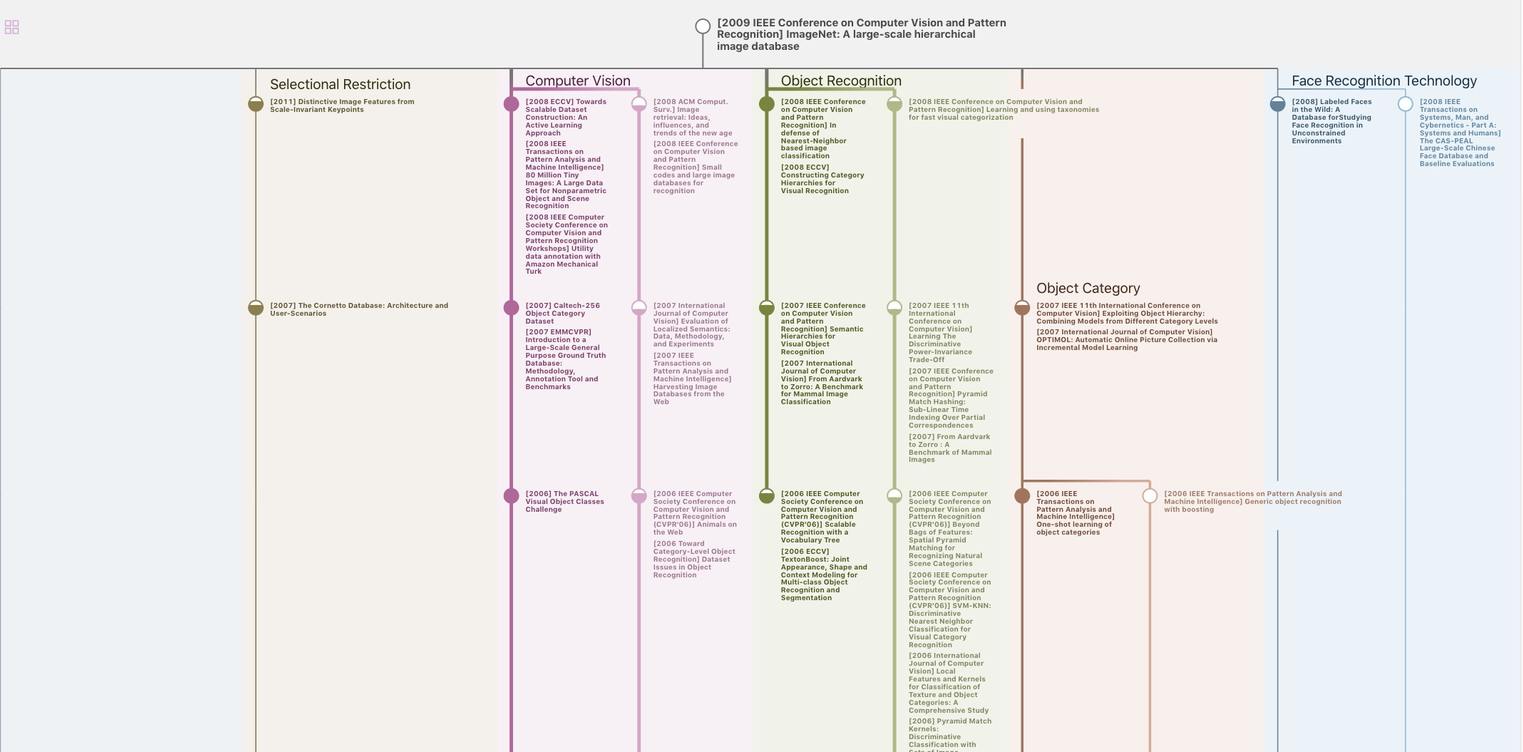
生成溯源树,研究论文发展脉络
Chat Paper
正在生成论文摘要