Surgical Aggregation: Federated Class-Heterogeneous Learning
arxiv(2023)
摘要
The release of numerous chest x-ray datasets has spearheaded the development
of deep learning models with expert-level performance. However, they have
limited interoperability due to class-heterogeneity – a result of inconsistent
labeling schemes and partial annotations. Therefore, it is challenging to
leverage these datasets in aggregate to train models with a complete
representation of abnormalities that may occur within the thorax. In this work,
we propose surgical aggregation, a federated learning framework for aggregating
knowledge from class-heterogeneous datasets and learn a model that can
simultaneously predict the presence of all disease labels present across the
datasets. We evaluate our method using simulated and real-world
class-heterogeneous datasets across both independent and identically
distributed (iid) and non-iid settings. Our results show that surgical
aggregation outperforms current methods, has better generalizability, and is a
crucial first step towards tackling class-heterogeneity in federated learning
to facilitate the development of clinically-useful models using previously
non-interoperable chest x-ray datasets.
更多查看译文
AI 理解论文
溯源树
样例
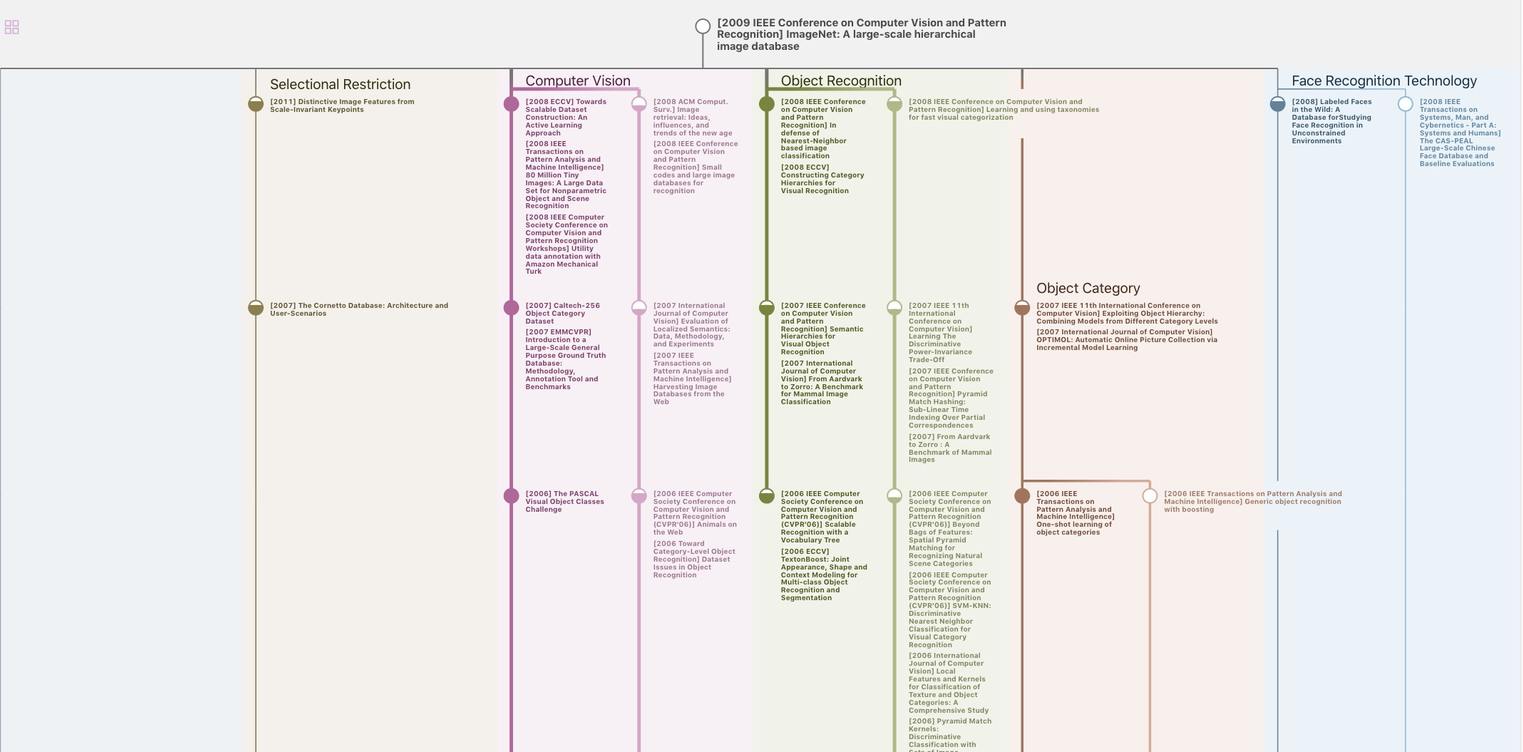
生成溯源树,研究论文发展脉络
Chat Paper
正在生成论文摘要