Conversion of the Mayo LDCT Data to Synthetic Equivalent through the Diffusion Model for Training Denoising Networks with a Theoretically Perfect Privacy
arxiv(2023)
摘要
Deep learning techniques are widely used in the medical imaging field; for example, low-dose CT denoising. However, all these methods usually require a large number of data samples, which are at risk of privacy leaking, expensive, and time-consuming. Because privacy and other concerns create challenges to data sharing, publicly available CT datasets are up to only a few thousand cases. Generating synthetic data provides a promising alternative to complement or replace training datasets without patient-specific information. Recently, diffusion models have gained popularity in the computer vision community with a solid theoretical foundation. In this paper, we employ latent diffusion models to generate synthetic images from a publicly available CT dataset-the Mayo Low-dose CT Challenge dataset. Then, an equivalent synthetic dataset was created. Furthermore, we use both the original Mayo CT dataset and the synthetic dataset to train the RED-CNN model respectively. The results show that the RED-CNN model achieved similar performance in the two cases, which suggests the feasibility of using synthetic data to conduct the low-dose CT research. Additionally, we use the latent diffusion model to augment the Mayo dataset. The results on the augmented dataset demonstrate an improved denoising performance.
更多查看译文
关键词
training denoising networks,privacy,diffusion model,mayo
AI 理解论文
溯源树
样例
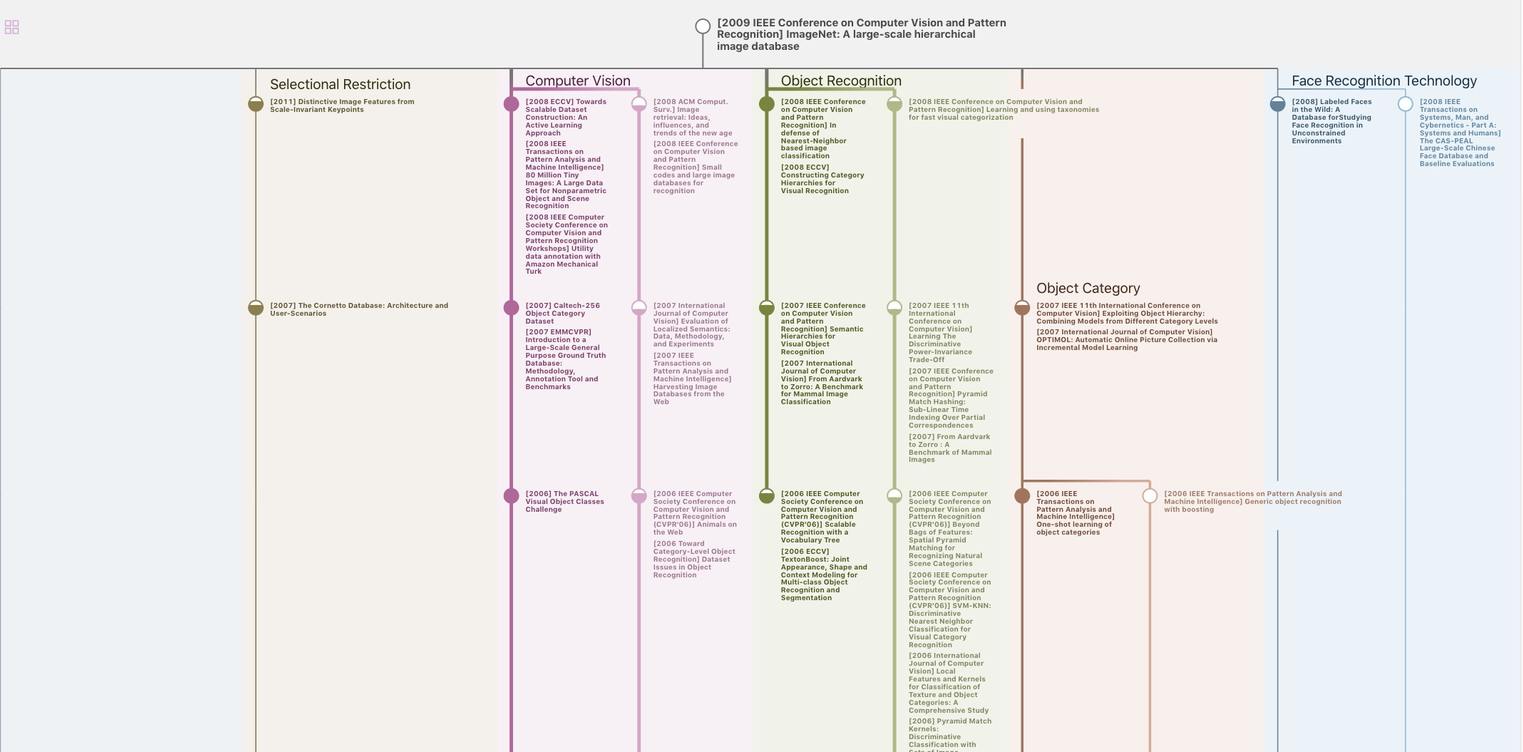
生成溯源树,研究论文发展脉络
Chat Paper
正在生成论文摘要