CRC-Aided Learned Ensembles of Belief-Propagation Polar Decoders
CoRR(2023)
摘要
Polar codes have promising error-correction capabilities. Yet, decoding polar codes is often challenging, particularly with large blocks, with recently proposed decoders based on list-decoding or neural-decoding. The former applies multiple decoders or the same decoder multiple times with some redundancy, while the latter family utilizes emerging deep learning schemes to learn to decode from data. In this work we introduce a novel polar decoder that combines the list-decoding with neural-decoding, by forming an ensemble of multiple weighted belief-propagation (WBP) decoders, each trained to decode different data. We employ the cyclic-redundancy check (CRC) code as a proxy for combining the ensemble decoders and selecting the most-likely decoded word after inference, while facilitating real-time decoding. We evaluate our scheme over a wide range of polar codes lengths, empirically showing that gains of around 0.25dB in frame-error rate could be achieved. Moreover, we provide complexity and latency analysis, showing that the number of operations required approaches that of a single BP decoder at high signal-to-noise ratios.
更多查看译文
关键词
crc-aided,belief-propagation
AI 理解论文
溯源树
样例
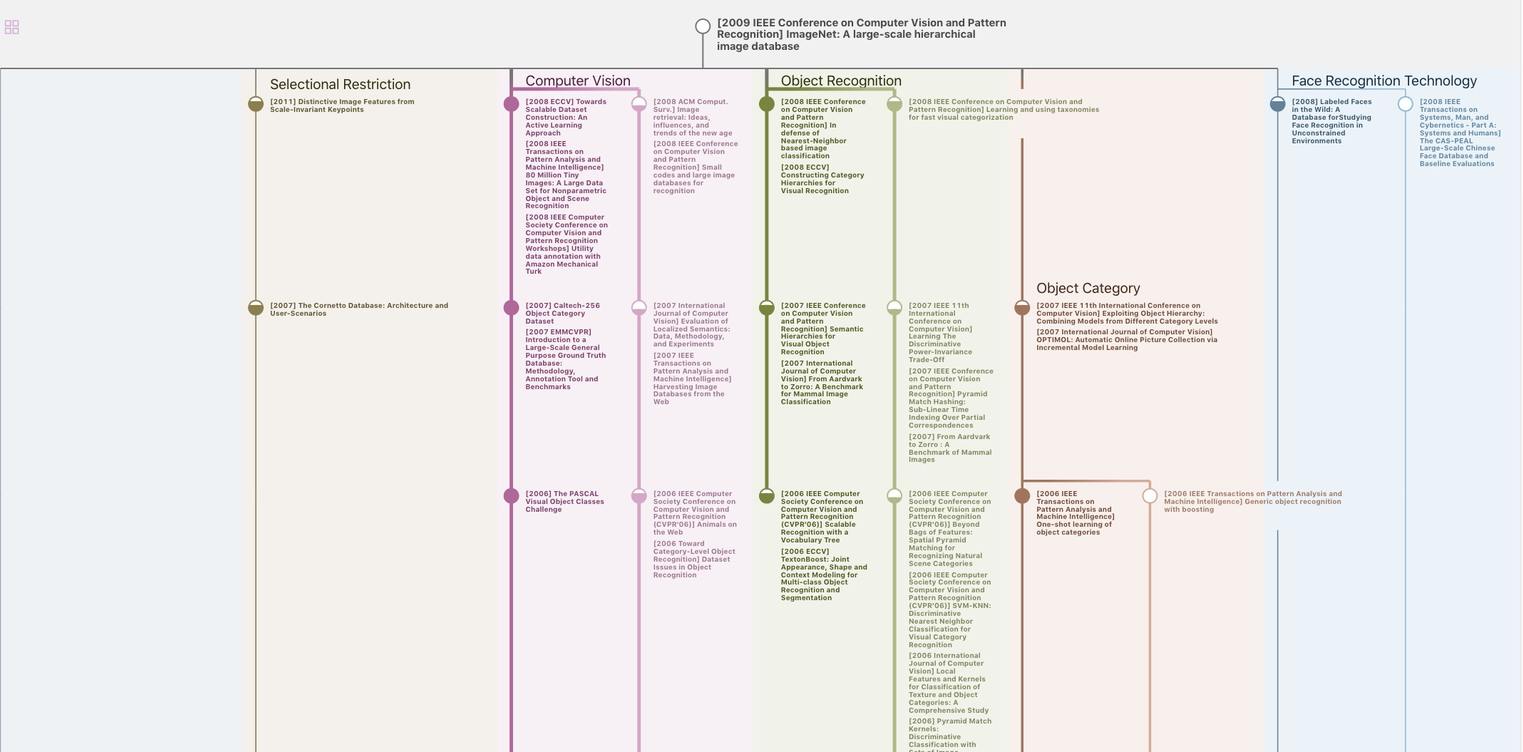
生成溯源树,研究论文发展脉络
Chat Paper
正在生成论文摘要