Rethinking precision of pseudo label: Test-time adaptation via complementary learning
PATTERN RECOGNITION LETTERS(2024)
摘要
Test-time adaptation (TTA) has been proven that is capable of transferring the source model to a different data distribution. The main challenge of TTA is how to learn the target-domain representation without the help of ground truth annotation. Previous studies have employed unsupervised objectives, such as the entropy of model predictions, as optimization targets to effectively learn features for test-time samples. However, the performance of the model is easily compromised by the quality of pseudo labels. In this work, we propose a novel way to leverage complementary learning to facilitate target feature learning, along with a complementary-labeling scenario and dynamical refining of the decision boundary. The use of complementary label learning effectively prevents the model from collapsing to high-confidence instances with incorrect pseudo labels. The complementary labels are less informative than ordinary labels and thus a suitable risk estimator is derived to better learn from them. We highlight that this learning of complementary labels agrees with Vanilla learning with ordinary data distribution. Comprehensive experiments show that the proposed learning algorithm achieves state-of-the-art performance on different datasets and experiment settings.
更多查看译文
关键词
Test-time adaptation,Complementary label,Unsupervised learning
AI 理解论文
溯源树
样例
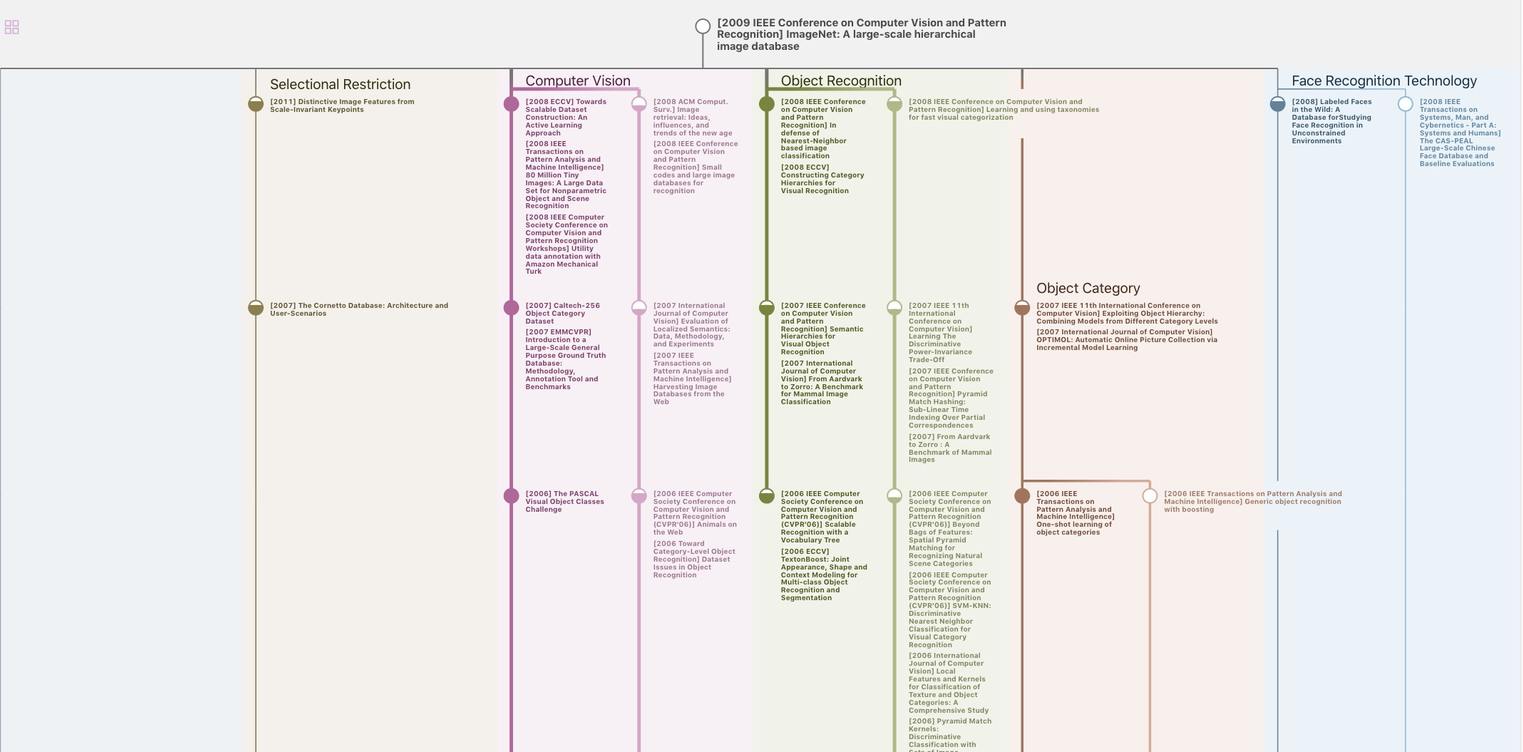
生成溯源树,研究论文发展脉络
Chat Paper
正在生成论文摘要