Efficient Activation Function Optimization through Surrogate Modeling
CoRR(2023)
摘要
Carefully designed activation functions can improve the performance of neural networks in many machine learning tasks. However, it is difficult for humans to construct optimal activation functions, and current activation function search algorithms are prohibitively expensive. This paper aims to improve the state of the art through three steps: First, the benchmark datasets Act-Bench-CNN, Act-Bench-ResNet, and Act-Bench-ViT were created by training convolutional, residual, and vision transformer architectures from scratch with 2,913 systematically generated activation functions. Second, a characterization of the benchmark space was developed, leading to a new surrogate-based method for optimization. More specifically, the spectrum of the Fisher information matrix associated with the model's predictive distribution at initialization and the activation function's output distribution were found to be highly predictive of performance. Third, the surrogate was used to discover improved activation functions in CIFAR-100 and ImageNet tasks. Each of these steps is a contribution in its own right; together they serve as a practical and theoretical foundation for further research on activation function optimization. Code is available at https://github.com/cognizant-ai-labs/aquasurf, and the benchmark datasets are at https://github.com/cognizant-ai-labs/act-bench.
更多查看译文
关键词
efficient activation function optimization
AI 理解论文
溯源树
样例
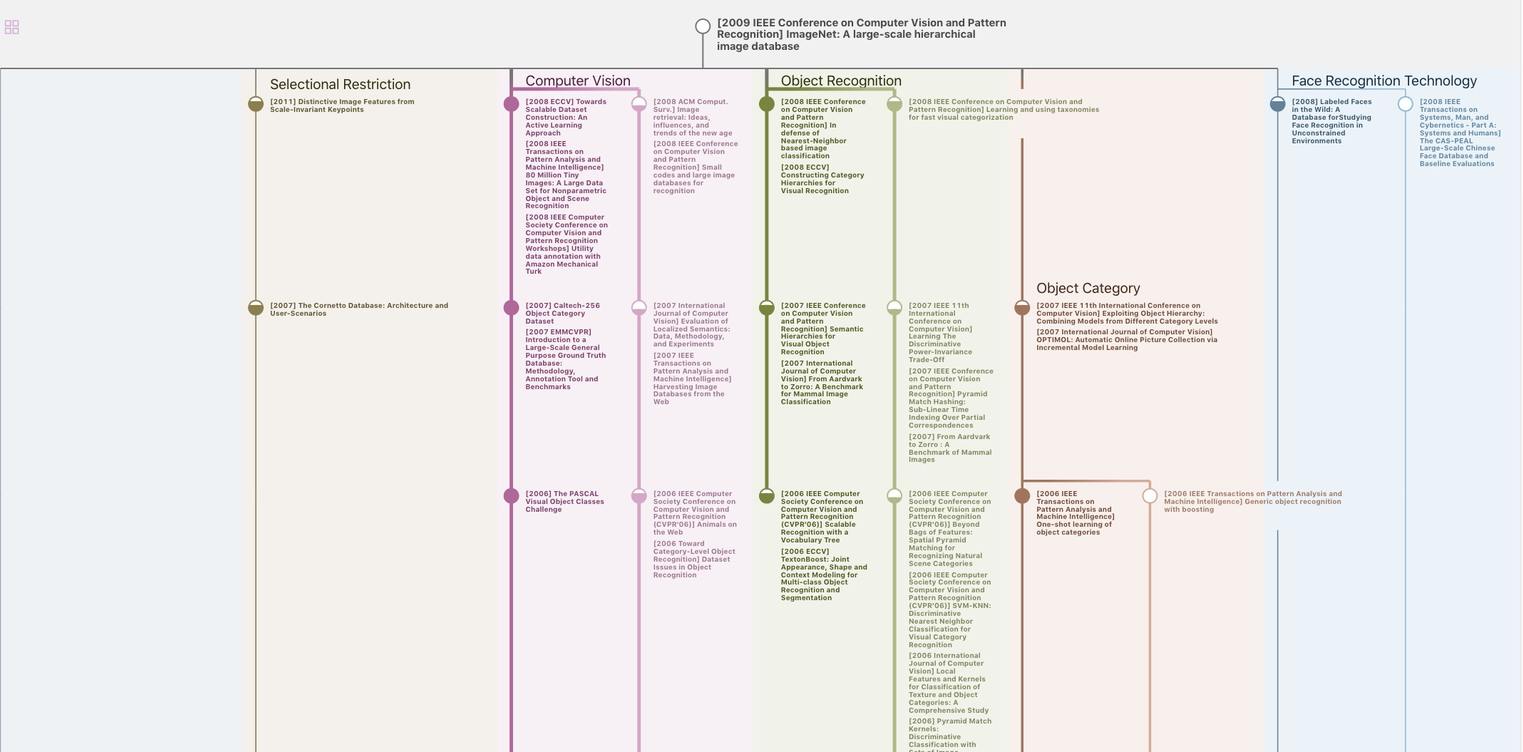
生成溯源树,研究论文发展脉络
Chat Paper
正在生成论文摘要