A domain-decomposed vae method for bayesian inverse problems
INTERNATIONAL JOURNAL FOR UNCERTAINTY QUANTIFICATION(2024)
摘要
Bayesian inverse problems are often computationally challenging when the forward model is governed by complex partial differential equations (PDEs). This is typically caused by expensive forward model evaluations and highdimensional parameterization of priors. This paper proposes a domain -decomposed variational autoencoder Markov chain Monte Carlo (DD-VAE-MCMC) method to tackle these challenges simultaneously. Through partitioning the global physical domain into small subdomains, the proposed method first constructs local deterministic generative models based on local historical data, which provide efficient local prior representations. Gaussian process models with active learning address the domain decomposition interface conditions. Then inversions are conducted on each subdomain independently in parallel and in low -dimensional latent parameter spaces. The local inference solutions are postprocessed through the Poisson image blending procedure to result in an efficient global inference result. Numerical examples are provided to demonstrate the performance of the proposed method.
更多查看译文
关键词
Bayesian inference,Markov chain Monte Carlo,domain decomposition,deep generative models
AI 理解论文
溯源树
样例
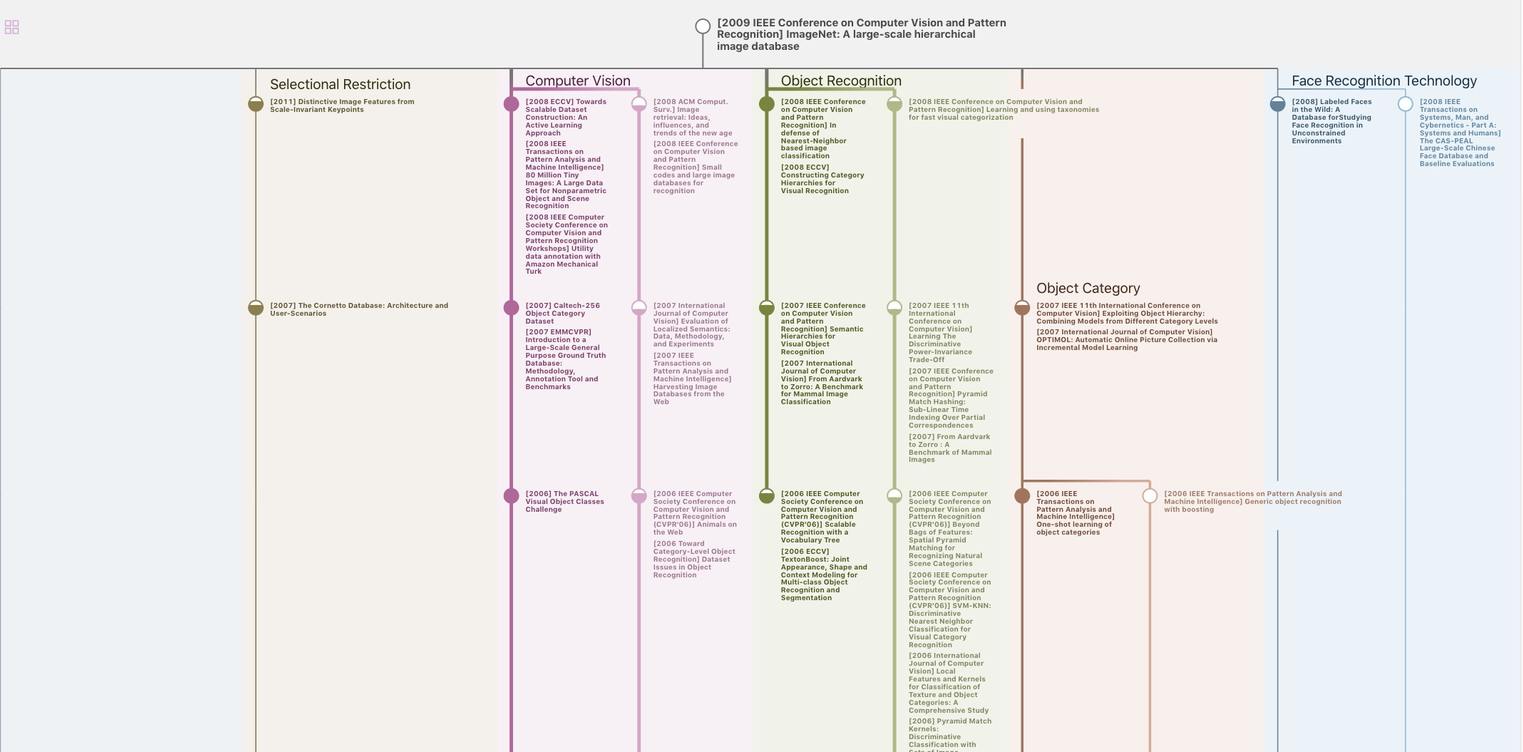
生成溯源树,研究论文发展脉络
Chat Paper
正在生成论文摘要