Hot-NetVLAD: Learning Discriminatory Key Points for Visual Place Recognition
IEEE Robotics and Automation Letters(2023)
摘要
Hot-NetVLAD implements a hot-spot detector on a learned local key-patch descriptor algorithm for Visual Place Recognition (VPR), thereby greatly cutting down the size of features extracted. The hot-spots pinpoint which regions are crucial for comparison when performing VPR. As hot-spots land on only a small portion of the feature space, the number of local descriptors extracted is greatly reduced. A novel method to extract ground truths of hot-spots in the context of VPR is proposed so that the hot-spot detector in Hot-NetVLAD can be trained for VPR purposes. Hot-NetVLAD is evaluated on the Pittsburgh250k and Tokyo24/7 datasets. While results show that Hot-NetVLAD trades some accuracy loss for storage efficiency, the recall remains competitive when compared to state-of-the-art methods. Furthermore, identified hot-spots bring new insights to key regions required for VPR, as they tend to fall on distinguishable static objects in the scene. This can potentially be applied to increase the robustness of mobile robot localization by increasing resilience to dynamic environments, whilst still being able to perform static obstacle matching effectively.
更多查看译文
关键词
Localization,vision-based navigation,intelligent transportation systems
AI 理解论文
溯源树
样例
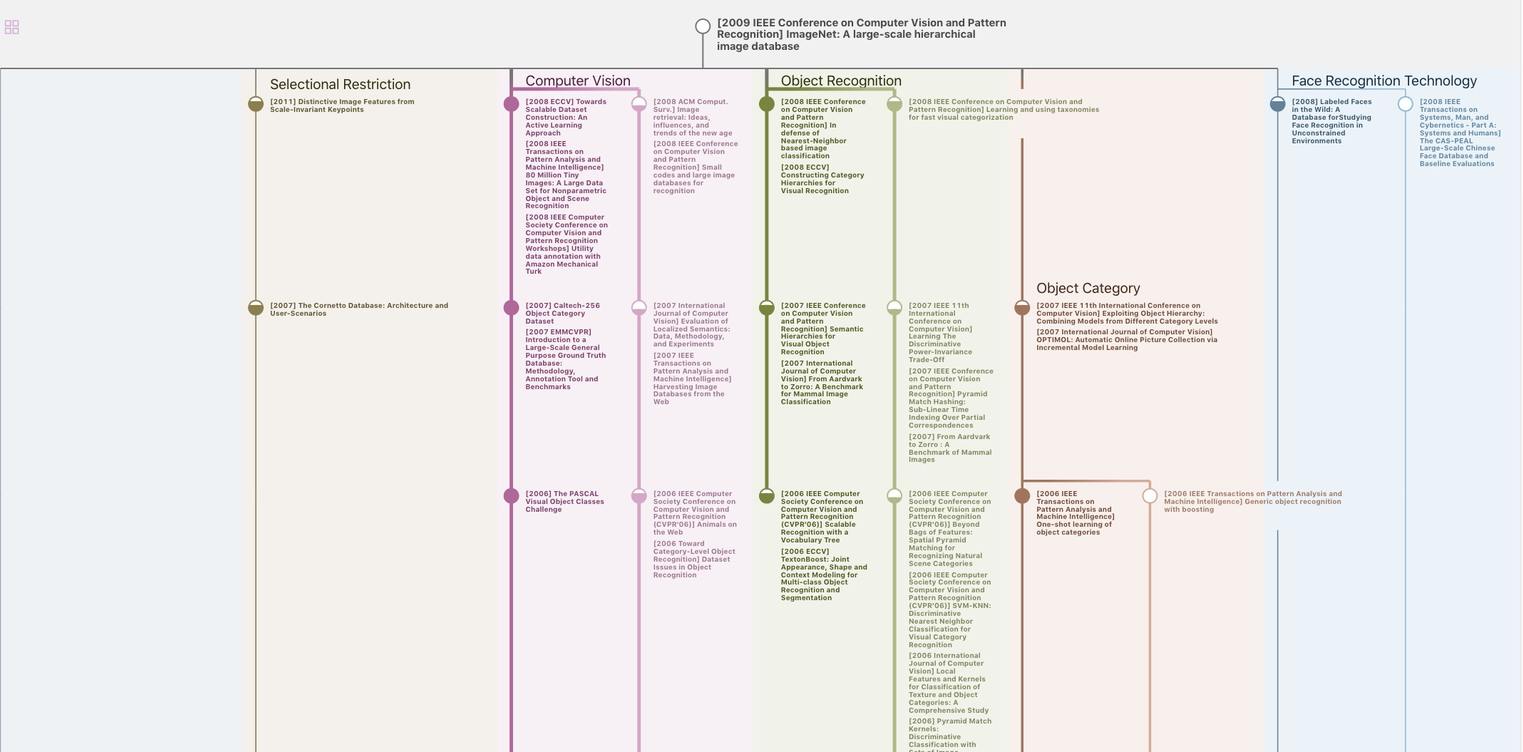
生成溯源树,研究论文发展脉络
Chat Paper
正在生成论文摘要