An Extreme Learning Machine Model for Venue Presence Detection
Proceedings of ELM 2021 Proceedings in Adaptation, Learning and Optimization(2023)
摘要
Value-added services allocation or denial in a particular venue for a given user is of high significance. It will get more prominent as we move to 5G and 6G networks’ roll out, as we will get other means to have better aids. In this paper, Extreme Learning Machines (ELM) model performance is compared with Support Vector Machines (SVM), K-Nearest Neighbors (KNN), Logistic Regression (LR), and Random Forest (RF) models for venue presence detection. The input data is collected from the number of UEs (User Equipment) simultaneously placed inside and outside a venue and kept for longer duration. UEs logs essential data such as received signal reference power for serving cells and neighbor candidate cells, along with other network information. Our findings show that ELM model performs above 95% accuracy for a count of zero, one, and two neighbors. The results get better as we consider the collected data from more neighbors’ cells in our ELM computation.
更多查看译文
关键词
extreme learning machine model,extreme learning machine,presence
AI 理解论文
溯源树
样例
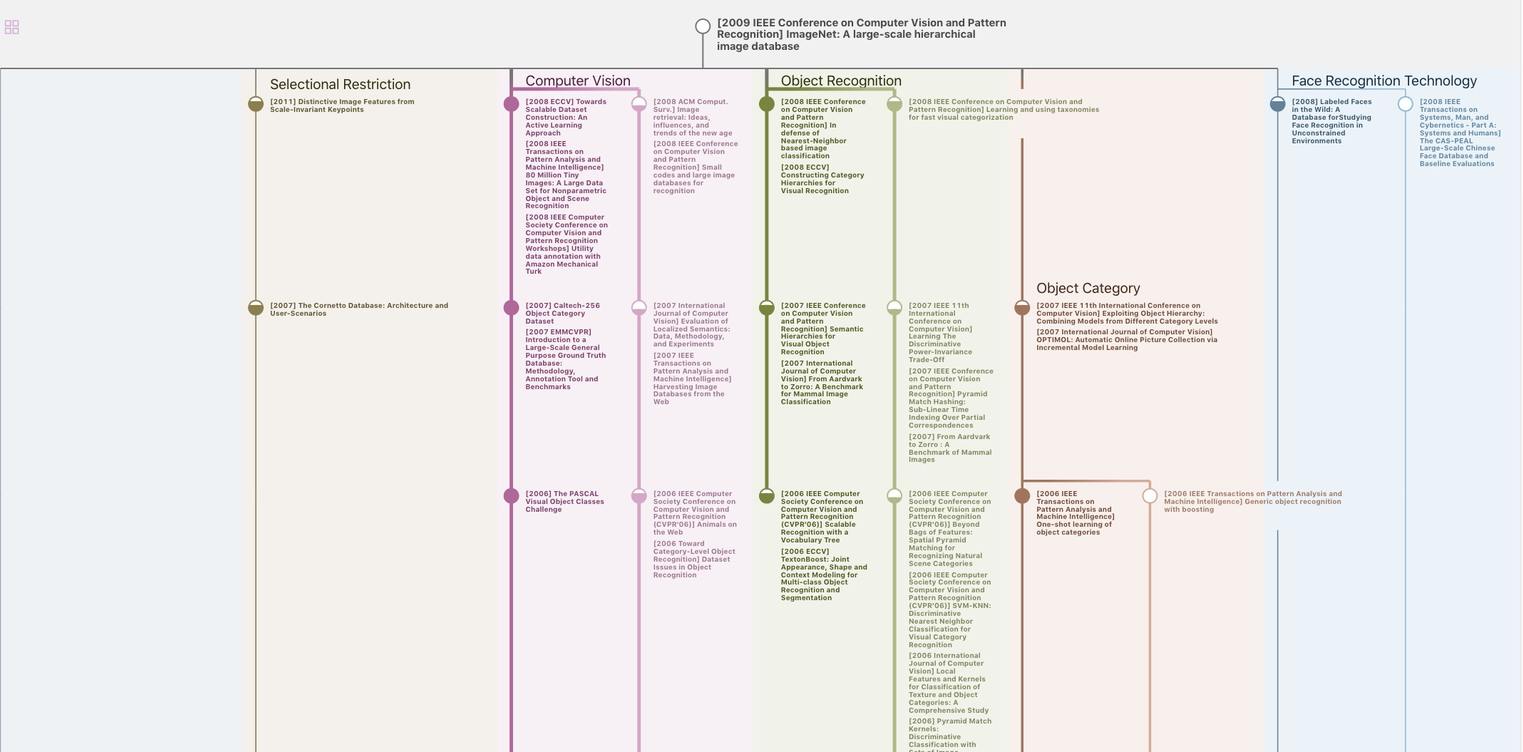
生成溯源树,研究论文发展脉络
Chat Paper
正在生成论文摘要