Re-planning of Quadrotors Under Disturbance Based on Meta Reinforcement Learning
J. Intell. Robotic Syst.(2023)
摘要
In searching for the stable motion re-planning as required by autonomous quadrotors, deep reinforcement learning ( DRL ) techniques play a vital role in balancing the reference trajectory tracking and flight safety. In this research, our intention amounts to improving the traditional DRL strategies in terms of the following two essential factors: 1). searching the best optimization without losing efficiency under the conditions of unstructured disturbances, e.g. , continuously changing wind perturbation or slight collisions; 2). balancing the safety and flight aggressiveness according to the intensity of the wind disturbance and the complexity of the environment. To be specific, in caring about the prompt convergence, in the present study, the reference trajectory with a re-timing publication mechanism was well-adopted for providing reasonable initial parameters for the proposed DRL model, hence improving the optimization performance of DRL strategies and meeting the requirements of smooth flight actions. Furthermore, the problem of motion re-planning in different environments was formulated into a series of partially observable Markov decision problems ( POMDPs ). Correspondingly, a learned objective function was introduced into the model agnostic meta-learning ( MAML ) framework and a MAML algorithm with mixed objective function ( OMAML ) was proposed for solving raised POMDPs while simultaneously enhancing the present method with the ability to balance flight aggressiveness and safety. Finally, sufficient simulation experiments were conducted in comparison with the state-of-the-art methods both in trajectory tracking and collision avoidance tasks, so as to demonstrate the effectiveness of the proposed method.
更多查看译文
关键词
Motion and path planning,Reinforcement learning,Wind disturbance,Collision avoidance
AI 理解论文
溯源树
样例
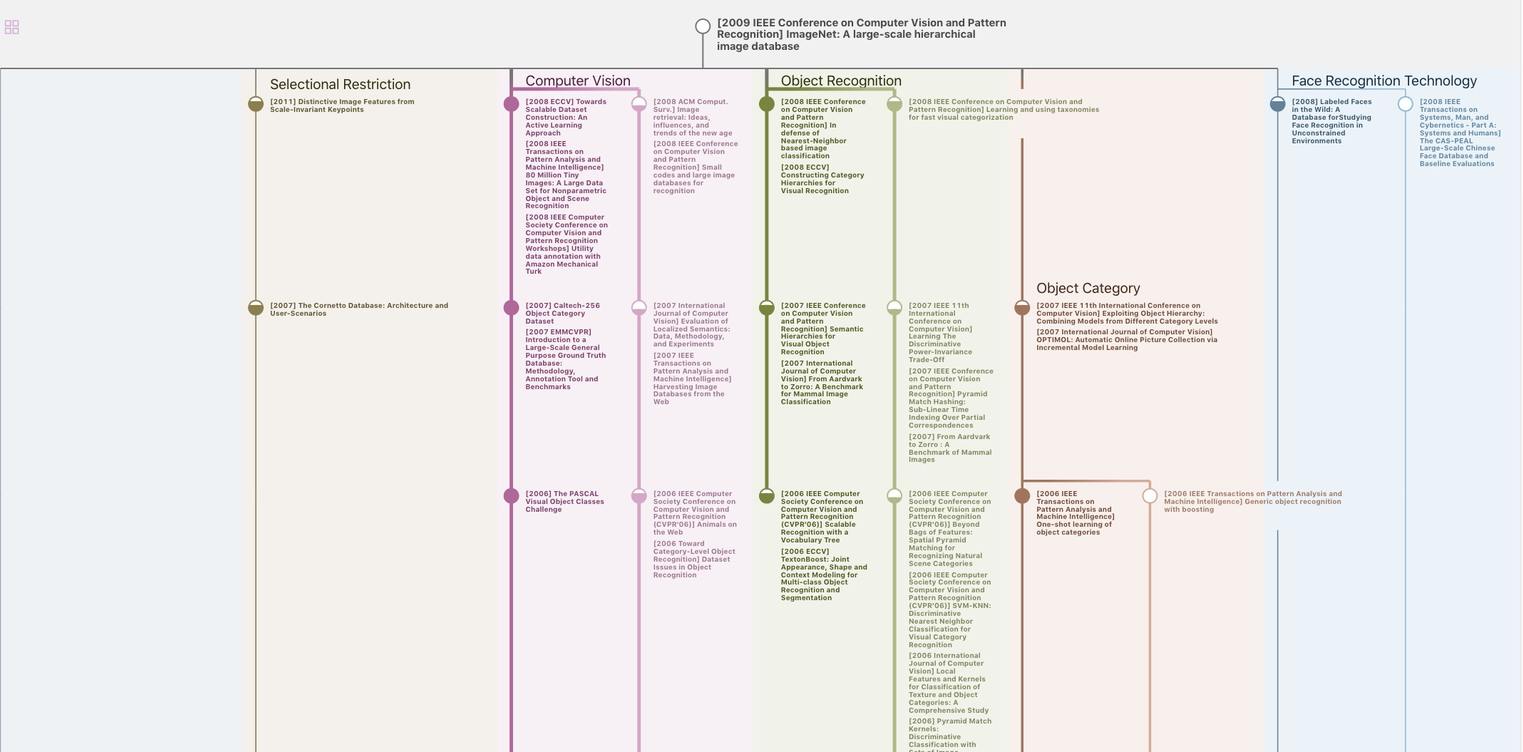
生成溯源树,研究论文发展脉络
Chat Paper
正在生成论文摘要