ResInformer: Residual Transformer-Based Artificial Time-Series Forecasting Model for PM2.5 Concentration in Three Major Chinese Cities
Mathematics(2023)
摘要
Many Chinese cities have severe air pollution due to the rapid development of the Chinese economy, urbanization, and industrialization. Particulate matter (PM2.5) is a significant component of air pollutants. It is related to cardiopulmonary and other systemic diseases because of its ability to penetrate the human respiratory system. Forecasting air PM2.5 is a critical task that helps governments and local authorities to make necessary plans and actions. Thus, in the current study, we develop a new deep learning approach to forecast the concentration of PM2.5 in three major cities in China, Beijing, Shijiazhuang, and Wuhan. The developed model is based on the Informer architecture, where the attention distillation block is improved with a residual block-inspired structure from efficient networks, and we named the model ResInformer. We use air quality index datasets that cover 98 months collected from 1 January 2014 to 17 February 2022 to train and test the model. We also test the proposed model for 20 months. The evaluation outcomes show that the ResInformer and ResInformerStack perform better than the original model and yield better forecasting results. This study’s methodology is easily adapted for similar efforts of fast computational modeling.
更多查看译文
关键词
air pollution,PM2,5,deep learning,time series,forecasting
AI 理解论文
溯源树
样例
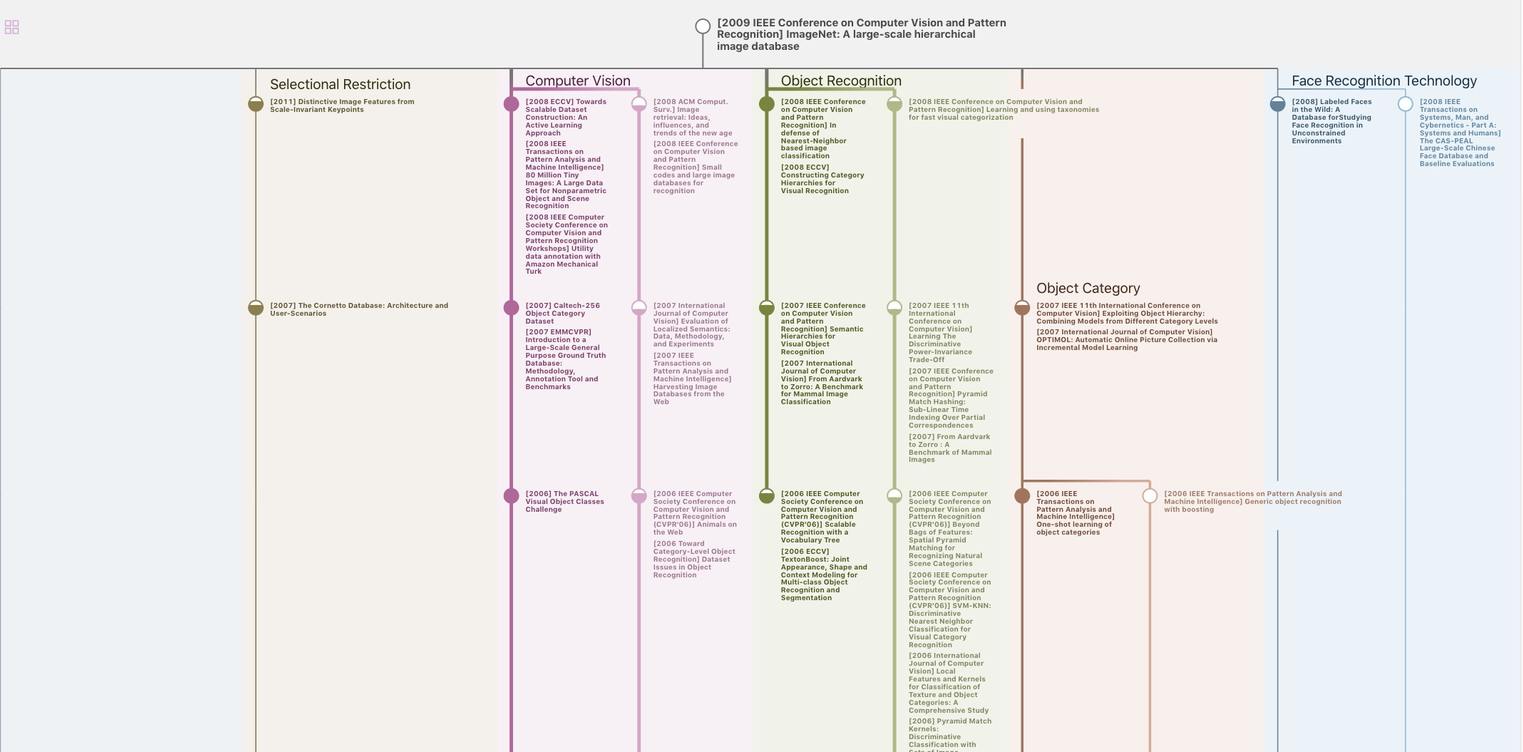
生成溯源树,研究论文发展脉络
Chat Paper
正在生成论文摘要