A satellite-driven model to estimate long-term particulate sulfate levels and attributable mortality burden in China
Environment international(2023)
摘要
Ambient fine particulate matter (PM2.5) pollution is a major environmental and public health challenge in China. In the recent decade, the PM2.5 level has decreased mainly driven by reductions in particulate sulfate as a result of large-scale desulfurization efforts in coal-fired power plants and industrial facilities. Emerging evidence also points to the differential toxicity of particulate sulfate affecting human health. However, estimating the long-term spatiotemporal trend of sulfate is difficult because a ground monitoring network of PM2.5 constituents has not been established in China. Spaceborne sensors such as the Multi-angle Imaging SpectroRadiometer (MISR) instrument can provide complementary information on aerosol size and type. With the help of state-of-the-art machine learning techniques, we developed a sulfate prediction model under support from available ground measurements, MISR-retrieved aerosol microphysical properties, and atmospheric reanalysis data at a spatial resolution of 0.1 degrees. Our sulfate model performed well with an out-of-bag cross-validation R-2 of 0.68 at the daily level and 0.93 at the monthly level. We found that the national mean population-weighted sulfate con-centration was relatively stable before the Air Pollution Prevention and Control Action Plan was enforced in 2013, ranging from 10.4 to 11.5 mu g m(-3). But the sulfate level dramatically decreased to 7.7 mu g m(-3) in 2018, with a change rate of-28.7 % from 2013 to 2018. Correspondingly, the annual mean total non-accidental and car-diopulmonary deaths attributed to sulfate decreased by 40.7 % and 42.3 %, respectively. The long-term, full -coverage sulfate level estimates will support future studies on evaluating air quality policies and understanding the adverse health effect of particulate sulfate.
更多查看译文
关键词
Air pollution,Particulate sulfate,Atmospheric big data,Machine learning,Spatiotemporal distribution,Health impact assessment
AI 理解论文
溯源树
样例
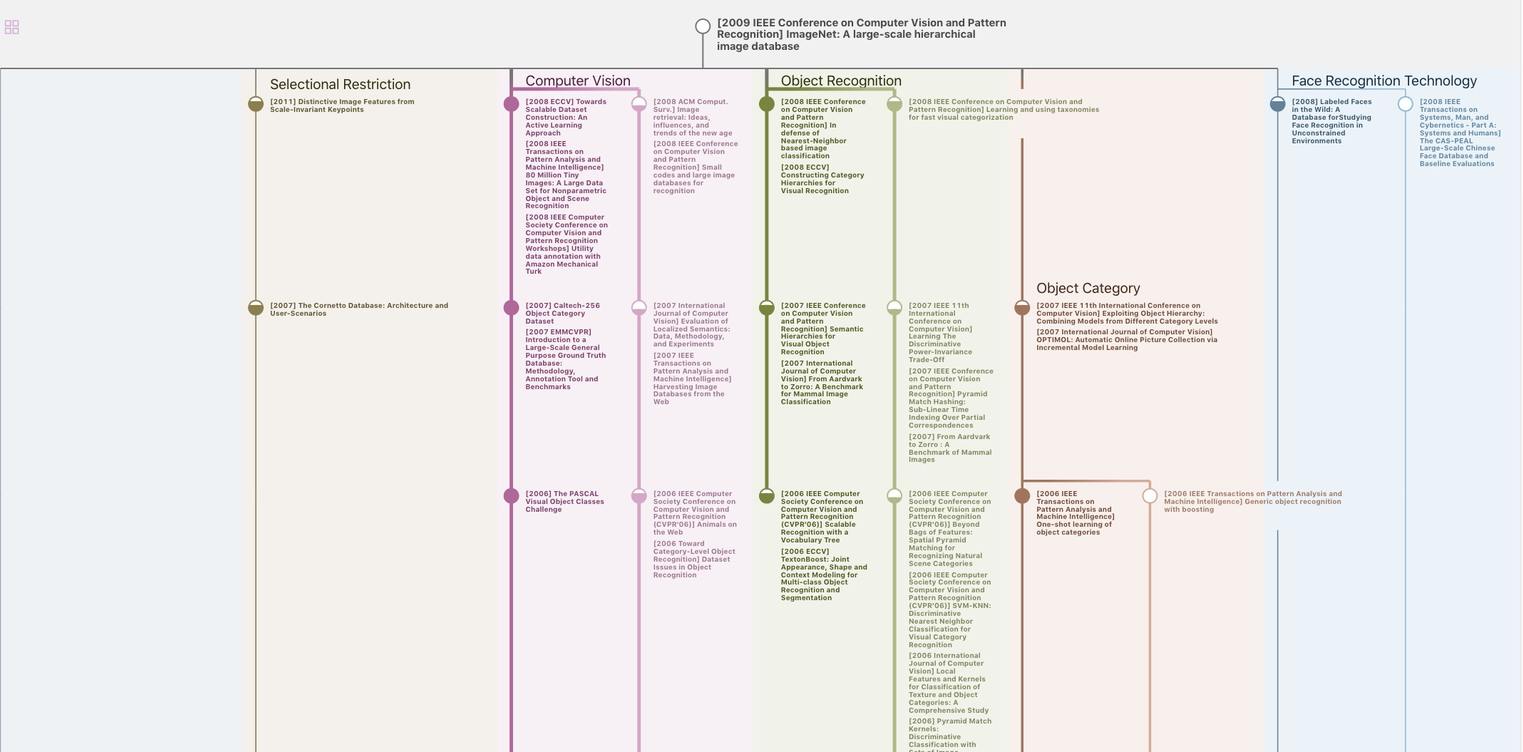
生成溯源树,研究论文发展脉络
Chat Paper
正在生成论文摘要