Two-channel deep recursive multi-scale network based on multi-attention for no-reference image quality assessment
International Journal of Machine Learning and Cybernetics(2023)
摘要
With the development of convolutional neural network (CNN) technology, No-reference Image Quality Assessment (NR-IQA) based on CNN has attracted the attention of many scholars. However, most of the previous methods improved the evaluation performance by increasing the network depth and various feature extraction mechanisms. This maybe causes some problems such as insufficient feature extraction, detail loss and gradient disappearance due to the limited samples with labels in the existing database. To learn feature representation more effectively, this paper proposes a Two-channel Deep Recursive Multi-Scale Network Based on Multi-Attention (ATDRMN), which can accurately evaluate image quality without relying on reference images. The network is a two-channel convolution networks with original image and gradient image as inputs. In two sub-branch networks, the Multi-scale Feature Extraction Block based on Attention (AMFEB) and the Improved Atrous Space Pyramid Pooling Network (IASPP-Net) are proposed to extend the attention-required feature information and obtain different levels of hierarchical feature information. Specifically, each AMFEB makes full use of image features in convolution kernels of different sizes to expand feature information, and further inputs these features into the attention mechanism to learn their corresponding weights. The output of each AMFEB is extended by the cavity convolution algorithm in IASPP-Net to obtain more context information and learn its hierarchical features. Finally, multiple AMFEBs and IASPP-Nets are respectively deeply and recursively fused to further obtain the most effective feature information, and then the output features are inputted to the regression network for final quality evaluation. The experimental results on seven databases showed that the proposed method has good robustness and is superior to the most advanced NR-IQA methods.
更多查看译文
关键词
Multi-scale,No reference image quality assessment,Deep recursive,Attention mechanism
AI 理解论文
溯源树
样例
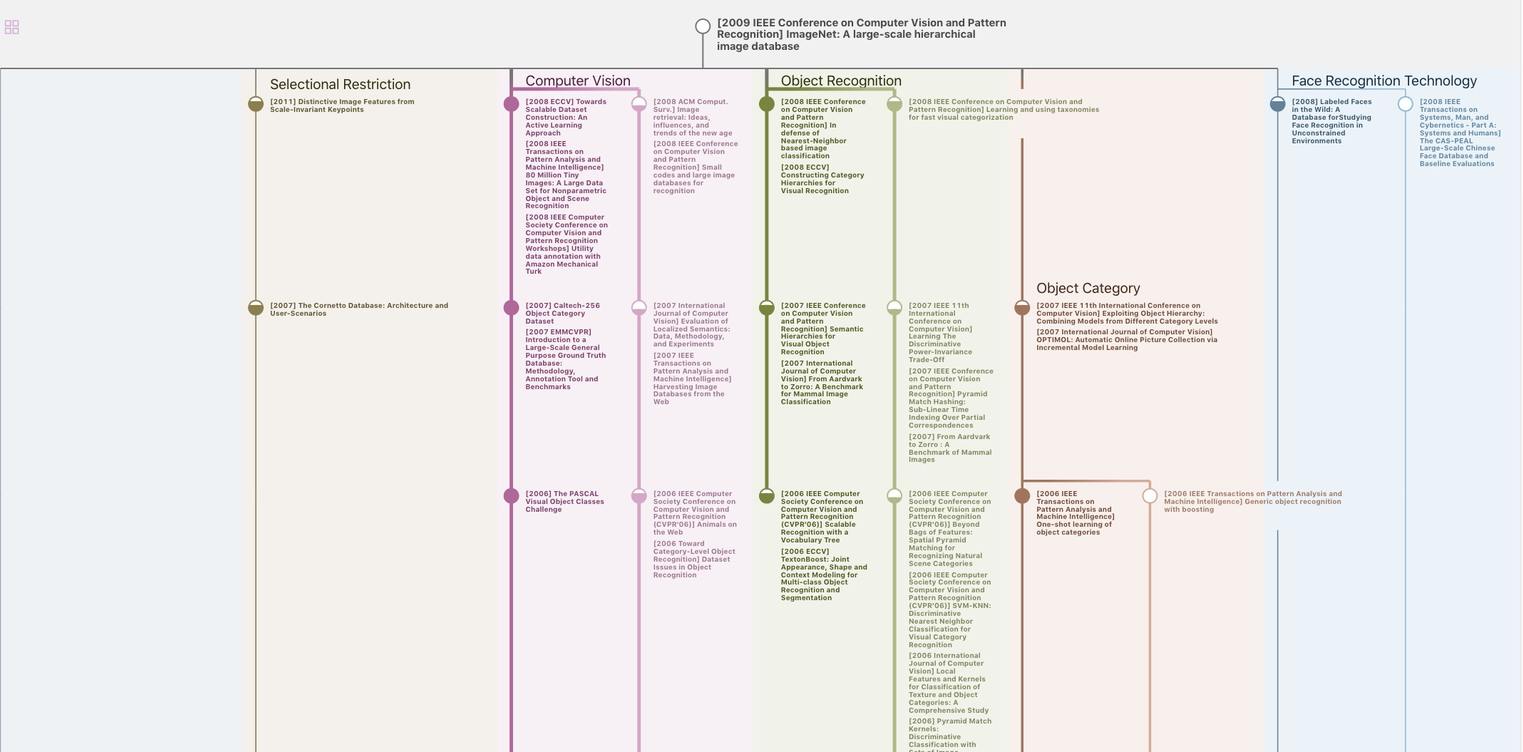
生成溯源树,研究论文发展脉络
Chat Paper
正在生成论文摘要