Energy-Saving Train Operation Synergy Based on Multi-Agent Deep Reinforcement Learning on Spark Cloud
IEEE Transactions on Vehicular Technology(2023)
摘要
With the development of urban rail transit, energy conservation has been highly concerned by the worldwide railway industry. The existing energy-saving control methods are hard to meet the requirements of large-scale multi-train intelligent cooperation. Furthermore, in order to optimize the overall energy consumption and make effective utilization of regenerative braking energy (RBE), the micro train driving strategies must be taken into account in the macro train schedule regulation. This article aims to establish a systematic optimization model to describe the train traffic environment and design a deep reinforcement learning (DRL) approach using multi-agent cooperative actor-critic (MACAC) to reschedule multiple trains for energy saving. Due to the high dimensional characteristic of control actions, the action representation technique is applied for generalization. The multiple agents are deployed on the Spark cloud platform to effectively improve the learning efficiency through multi-node parallel computing. The proposed MACAC approach on Spark cloud is capable of dealing with the intelligent learning and computing efficiency of large-scale train operation synergy, and saving energy to the maximum extent on the premise of satisfying various time constraints. The evaluation was carried out through the field data of Beijing Yizhuang subway line. The results show that the proposed MACAC approach can effectively learn to improve the energy efficiency, and the parallel computing method on Spark cloud is suitable for practical applications.
更多查看译文
关键词
Energy saving,multi-agent deep reinforcement learning,regenerative braking,schedule regulation
AI 理解论文
溯源树
样例
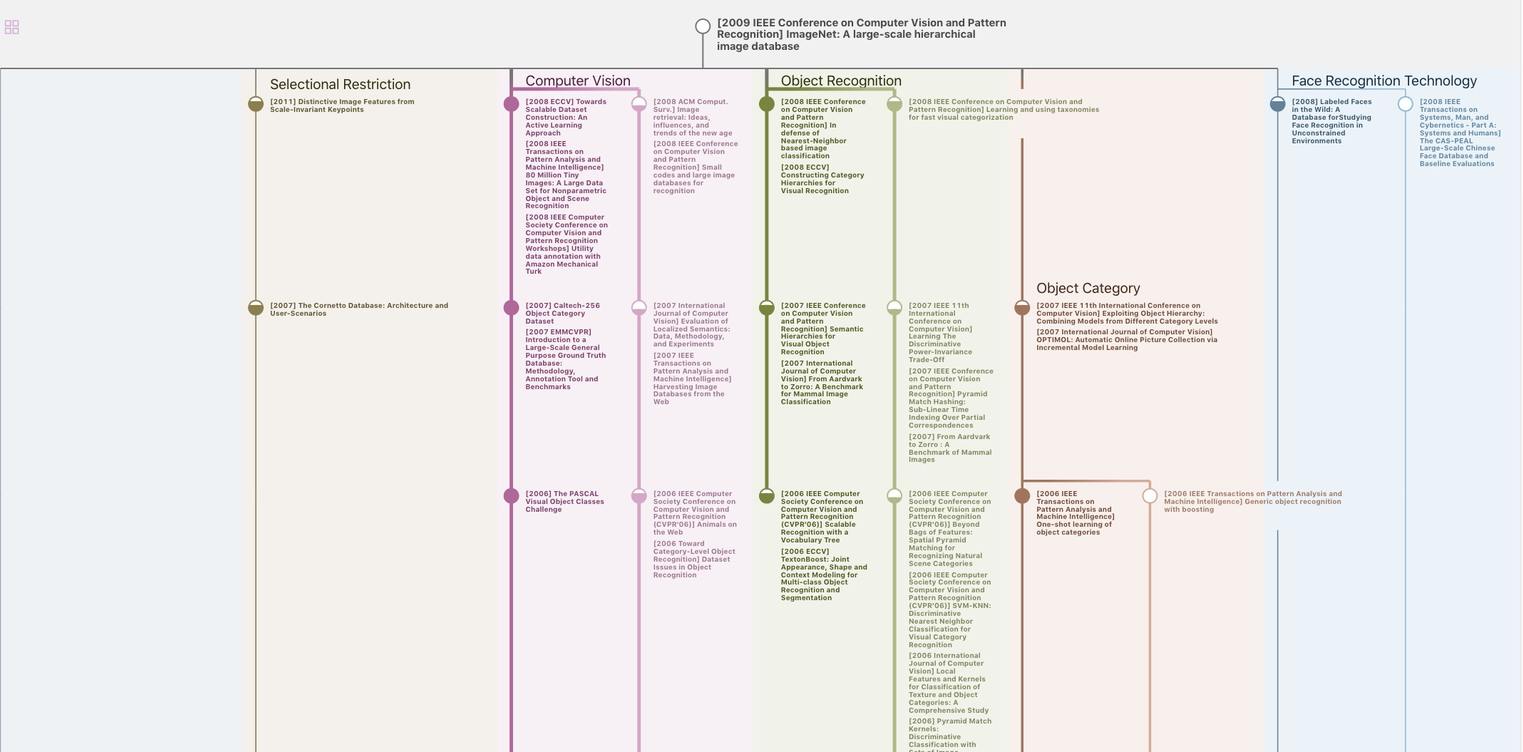
生成溯源树,研究论文发展脉络
Chat Paper
正在生成论文摘要