Uncertainty quantification of multi-scale resilience in networked systems with nonlinear dynamics using arbitrary polynomial chaos
Scientific reports(2023)
摘要
Complex systems derive sophisticated behavioral dynamics by connecting individual component dynamics via a complex network. The resilience of complex systems is a critical ability to regain desirable behavior after perturbations. In the past years, our understanding of large-scale networked resilience is largely confined to proprietary agent-based simulations or topological analysis of graphs. However, we know the dynamics and topology both matter and the impact of model uncertainty of the system remains unsolved, especially on individual nodes. In order to quantify the effect of uncertainty on resilience across the network resolutions (from macro-scale network statistics to individual node dynamics), we employ an arbitrary polynomial chaos (aPC) expansion method to identify the probability of a node in losing its resilience and how the different model parameters contribute to this risk on a single node. We test this using both a generic networked bi-stable system and also established ecological and work force commuter network dynamics to demonstrate applicability. This framework will aid practitioners to both understand macro-scale behavior and make micro-scale interventions.
更多查看译文
关键词
resilience,chaos,networked systems,nonlinear dynamics,uncertainty,multi-scale
AI 理解论文
溯源树
样例
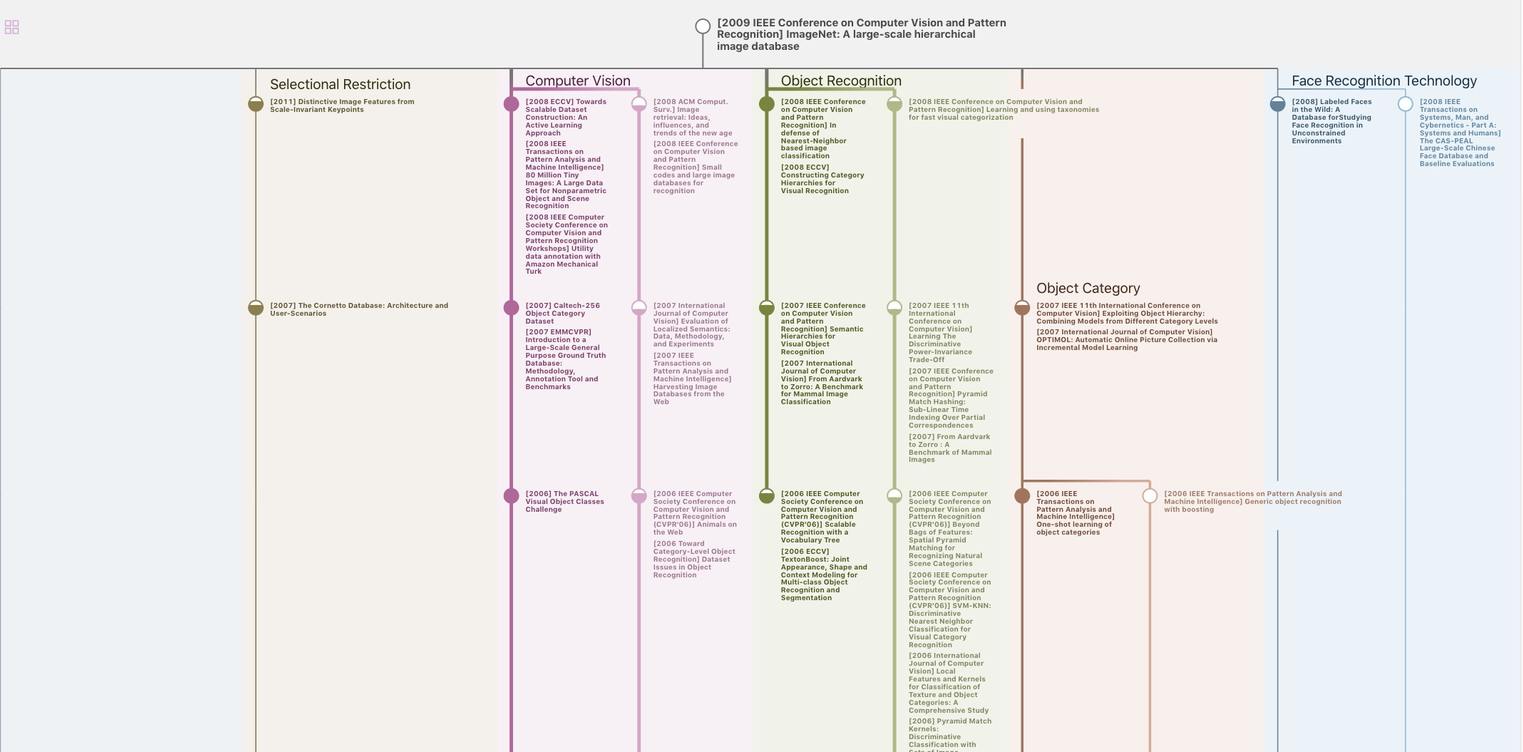
生成溯源树,研究论文发展脉络
Chat Paper
正在生成论文摘要