LR-ProtoNet: Meta-Learning for Low-Resolution Few-Shot Recognition and Classification
2022 IEEE International Conference on Networking, Sensing and Control (ICNSC)(2022)
摘要
For the few-shot classification problem of low-resolution(LR) images, we propose a meta-learning method based on prototypical networks called LR-ProtoNet. The metric-based meta-learning algorithm mainly extracts the features of the support and query samples through the feature encoder and obtains the prediction categories from a metric module. Our core idea is to add feature-affine layers in the feature encoder to increase the feature distribution of LR images, and use Brownian Distance Covariance(BDC) in the metric module to capture the joint distribution and nonlinear relationship between different affine transformations. We down-sample standard few-shot image datasets to simulate LR images and conduct extensive ablation experiments and comparative studies of other meta methods in general image recognition and fine-grained classification. Experimental results demonstrate that our proposed model can effectively utilize low-resolution image information, achieving state-of-the-art performance compared to baseline works.
更多查看译文
关键词
low-resolution image recognition,meta learning,prototypical networks,few-shot classification
AI 理解论文
溯源树
样例
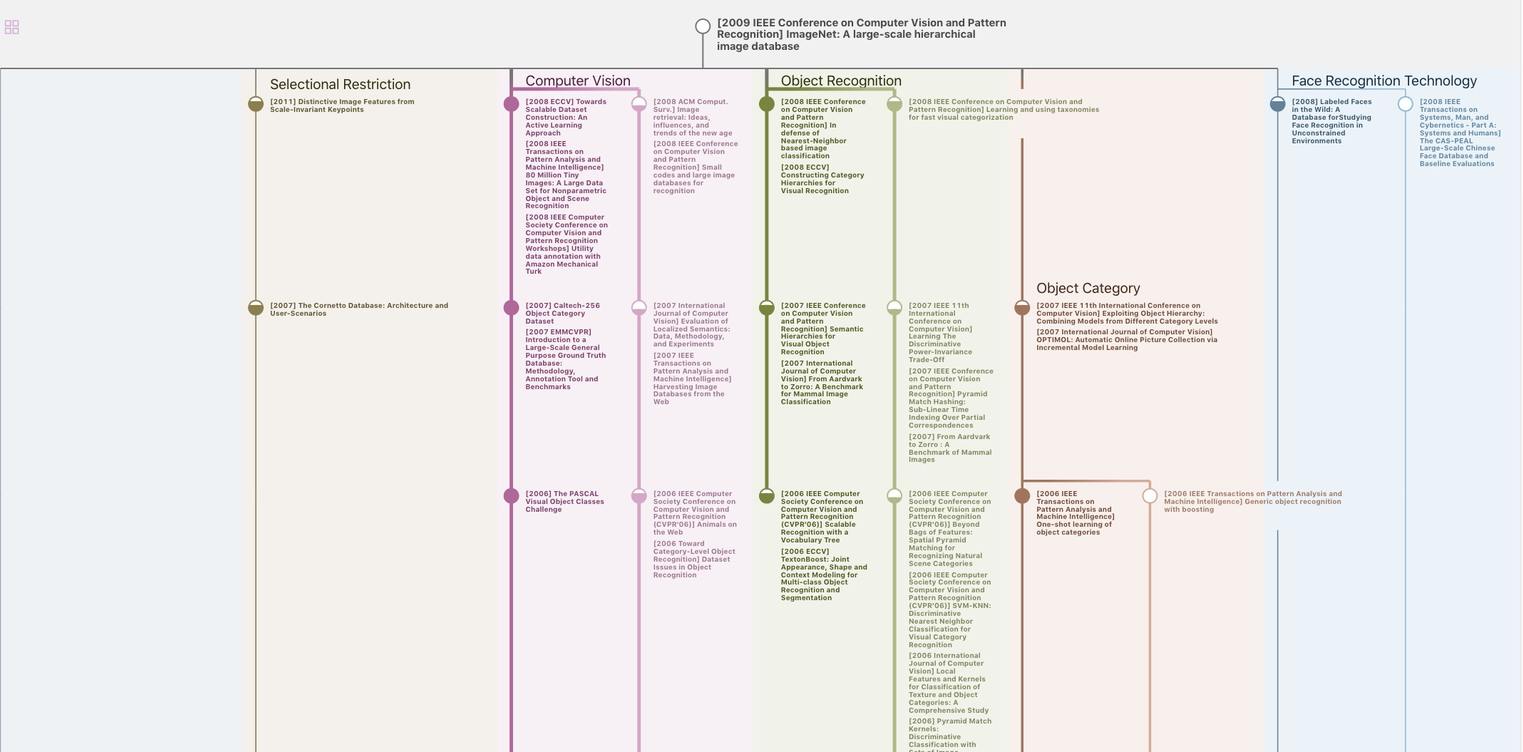
生成溯源树,研究论文发展脉络
Chat Paper
正在生成论文摘要