An Interactive Dashboard for Predicting Bank Customer Attrition
2022 International Conference on Emerging Trends in Computing and Engineering Applications (ETCEA)
摘要
Customer attrition rate is a significant concern for commercial banks. In this era of increased competition, banks have to compete fiercely to retain existing customers, particularly high-grade customers. Commercial banks have a clear motivation to predict customer attrition and by taking appropriate actions beforehand, they can not only significantly increase profits, but also, enhance their core competitiveness. This paper presents six machine learning algorithms, Logistic Regression, Support Vector Machine, Decision Tree, Random Forest, Gradient Boosting Classifier and K-Nearest Neighbor, which are trained using two different publicly available datasets to predict bank customer attrition rates. The results of the algorithms are measured using four metrics: Accuracy, Precision, Recall, and F-Measure. In addition, a dashboard is designed that can provide exploratory analysis of current customers and also provides their loyalty status prediction using the mentioned machine learning algorithms. Results show that Gradient Boosting Classifier and Random Forest algorithms performed the best, reaching an average accuracy of about 87% and 97%, respectively for both datasets.
更多查看译文
关键词
Machine Learning,Business Intelligence,Customer Churn,Prediction
AI 理解论文
溯源树
样例
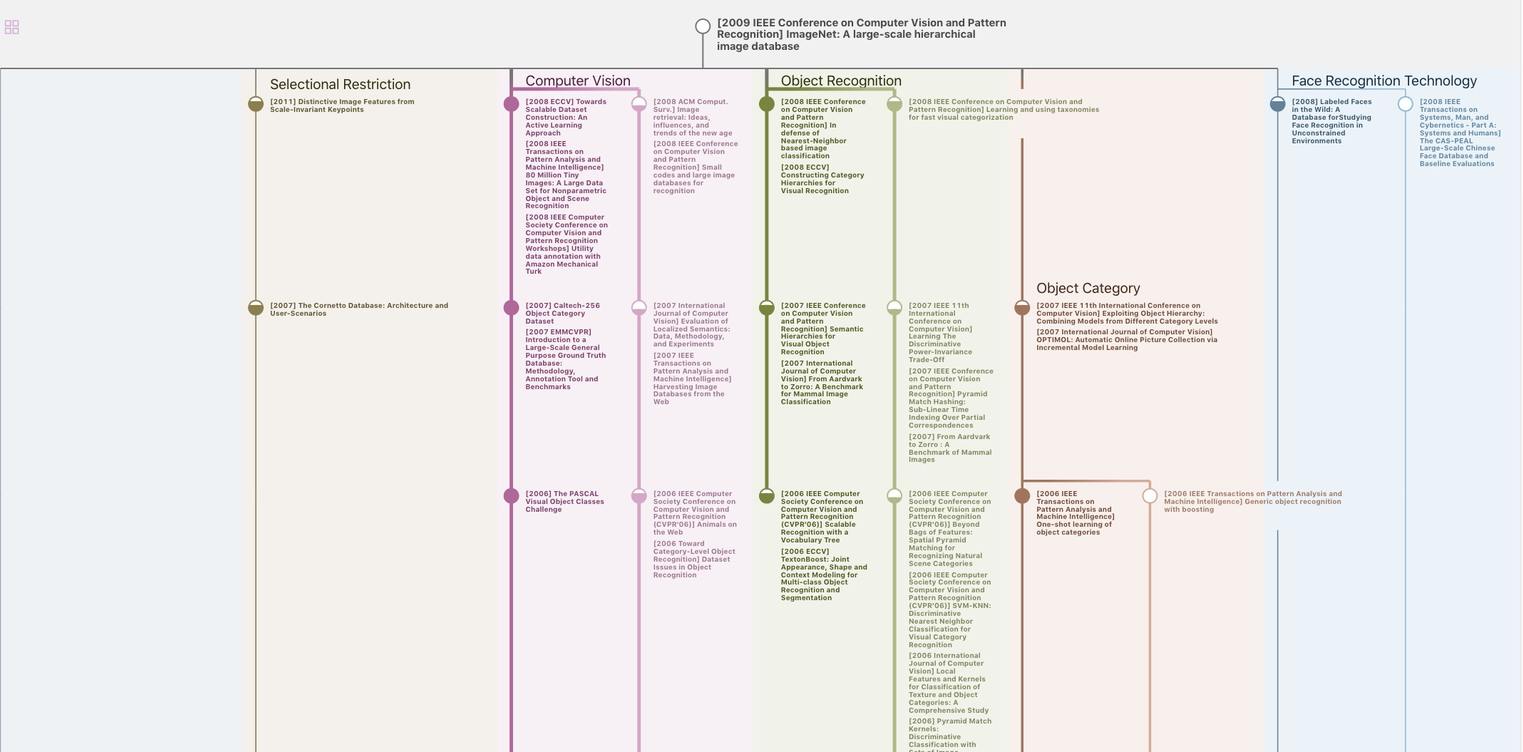
生成溯源树,研究论文发展脉络
Chat Paper
正在生成论文摘要