Server load and network-aware adaptive deep learning inference offloading for edge platforms
Internet of Things(2023)
摘要
This work presents DIAMOND, a deep neural network computation offloading scheme consisting of a lightweight client-to-server latency profiling component combined with a server inference time estimation module to accurately assess the expected latency of a deep learning model inference. Latency predictions for both the network and server are comprehensively used to make dynamic (partial) model offloading decisions at the client in run-time. Compared to previous work, DIAMOND targets to minimize network latency estimation overhead and considers the concurrent processing nature of state-of-the-art deep learning inference server designs. Our extensive evaluations with an NVIDIA Jetson Nano client connected to an NVIDIA Triton server shows that DIAMOND completes inference operations with noticeably reduced computational/energy overhead and latency compared to previously proposed model offloading approaches. Furthermore, our results show that DIAMOND well-adapts to practical server load and network dynamics.
更多查看译文
关键词
Computation offloading,Deep neural networks,Distributed systems,Mobile/embedded computing
AI 理解论文
溯源树
样例
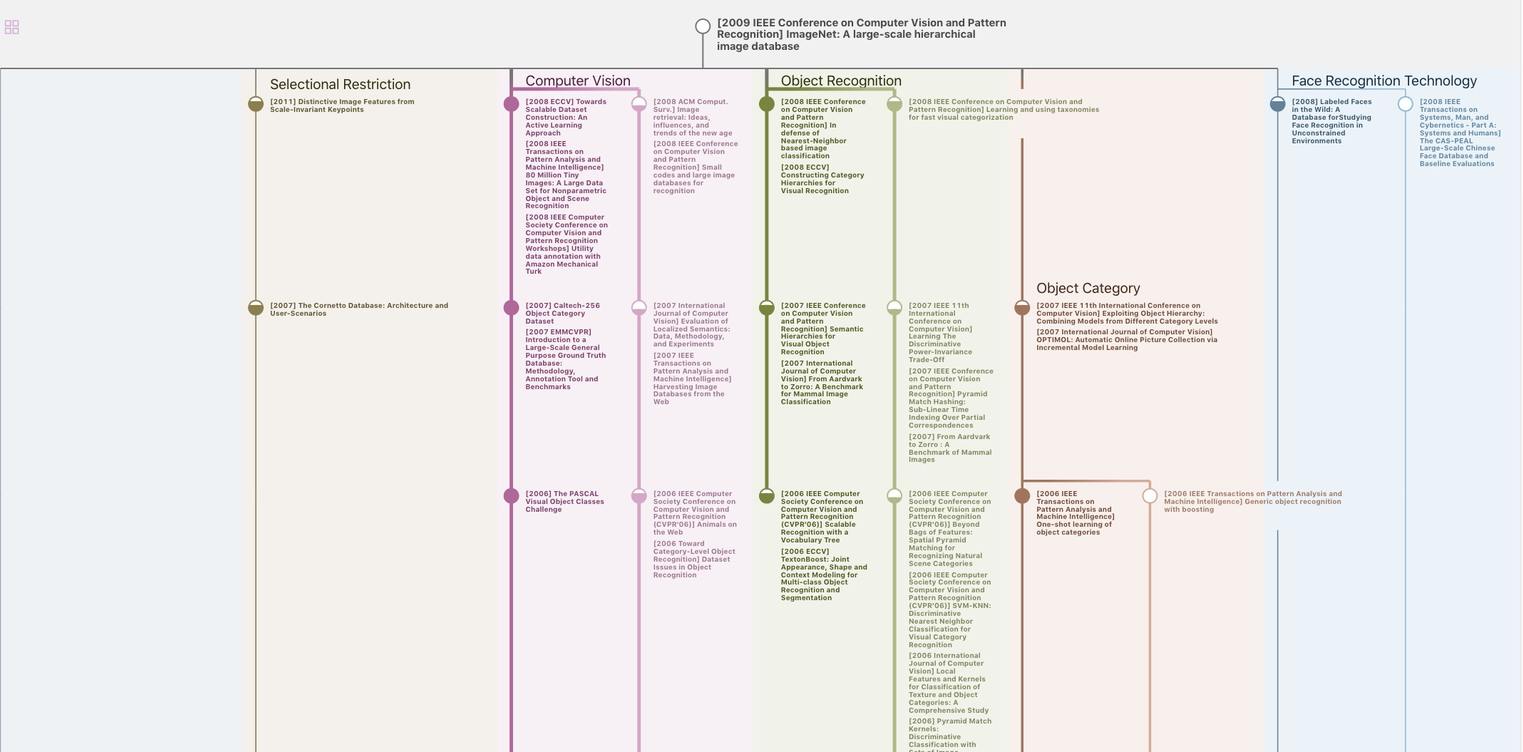
生成溯源树,研究论文发展脉络
Chat Paper
正在生成论文摘要