A grey wolf optimizer-based chaotic gravitational search algorithm for global optimization
The Journal of Supercomputing(2022)
Abstract
Gravitational search algorithm (GSA) is widely accepted as one of the effective optimization algorithms providing promising results. However, it suffers with local optima and premature convergence. To deal with those problems, chaotic gravitational constants for the gravitational search algorithm (CGSA) are proposed to enhance the efficiency of exploration via embedding chaotic maps, but the enhancement of exploration throughout iterations could somehow degrade the exploitation ability of GSA. Accordingly, this paper introduces a location update strategy inspired by the hunting mechanism of grey wolf optimizer (GWO) for improving the exploitation process of CGSA. In addition, a negative entropy function is designed to effectively control the implementation of the proposed strategy. In order to show the superior performance of the grey wolf chaotic gravitational search algorithm (GWCGSA), we carry out a comparative study of 30 benchmark functions (CEC 2014) with multiple GSAs and state-of-the-art algorithms. The experimental results show that the proposed strategy achieves better performance in most functions (greater than 20/30). Also, the experiments on seven engineering optimization problems indicate that the practicality of the proposed algorithm can be ensured.
MoreTranslated text
Key words
Gravitational search algorithm,Exploration and exploitation,Grey wolf optimizer,Chaotic map,Numerical optimization
AI Read Science
Must-Reading Tree
Example
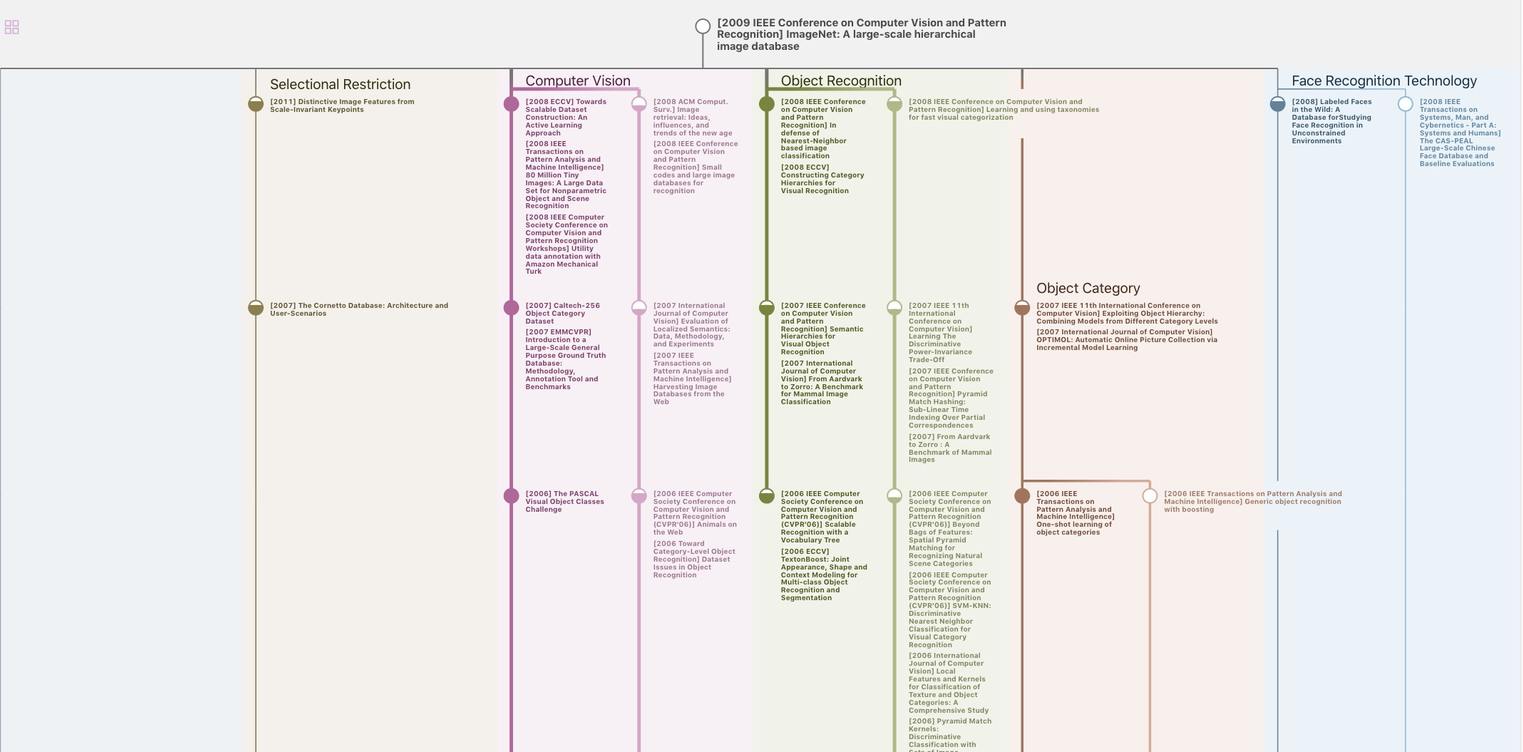
Generate MRT to find the research sequence of this paper
Chat Paper
Summary is being generated by the instructions you defined