Adaptive neighbourhood recovery method for machine learning based 3D point cloud classification
International Journal of Remote Sensing(2023)
摘要
3D scene analysis and classification by automatically assigning semantic labels to 3D points has become a major concern in remote sensing, photogrammetry and computer vision. Due to the high redundancy of point clouds and unbalanced sampling density, it is difficult to recover appropriately sized neighbourhoods to extract local features from 3D point clouds, which will decrease the classification performance. Existing point cloud neighborhood recovery methods usually use fixed-size neighbourhoods, where the shape structure of the point cloud and the distribution of the input points are usually disregarded. To avoid the above shortcomings, an adaptive 3D point cloud neighbourhood recovery method is proposed, which fully considers the correlation between point clouds and the surface variation of local neighbourhoods in the neighbourhood recovery process, and recovers dynamically sized optimal neighbourhoods for point clouds. The performance of our proposed method is evaluated on the Oakland dataset and the Vaihingen dataset with various modern classifiers. Experiments demonstrate that our proposed method achieves a superior classification result.
更多查看译文
关键词
Point cloud classification,neighbourhood recovery,feature extraction,machine learning
AI 理解论文
溯源树
样例
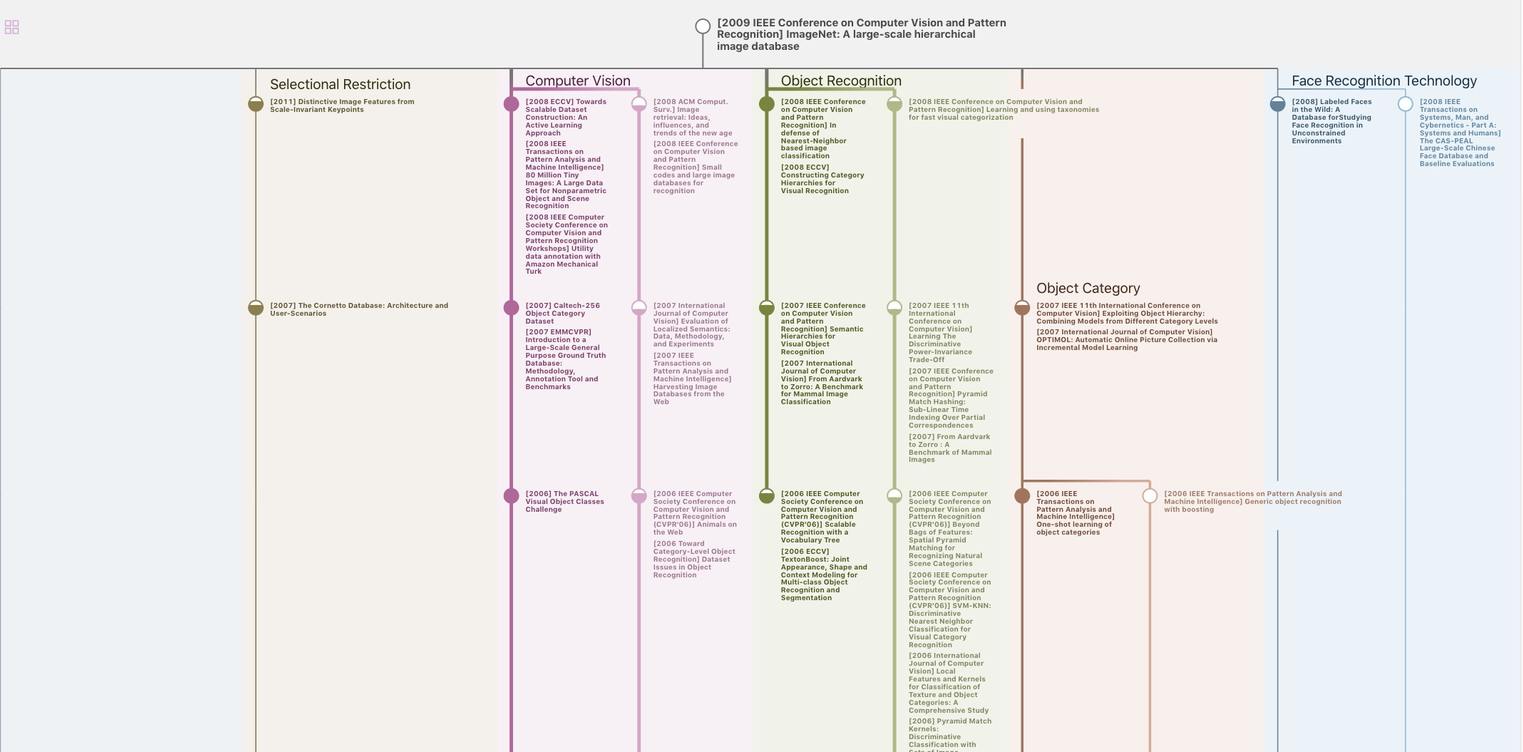
生成溯源树,研究论文发展脉络
Chat Paper
正在生成论文摘要