Graph neural networks for conditional de novo drug design
WIREs Computational Molecular Science(2023)
摘要
Drug design is costly in terms of resources and time. Generative deep learning techniques are using increasing amounts of biochemical data and computing power to pave the way for a new generation of tools and methods for drug discovery and optimization. Although early methods used SMILES strings, more recent approaches use molecular graphs to naturally represent chemical entities. Graph neural networks (GNNs) are learning models that can natively process graphs. The use of GNNs in drug discovery is growing exponentially. GNNs for drug design are often coupled with conditioning techniques to steer the generation process towards desired chemical and biological properties. These conditioned graph-based generative models and frameworks hold promise for the routine application of GNNs in drug discovery.This article is categorized under:Data Science > Artificial Intelligence/Machine LearningData Science > ChemoinformaticsData Science > Computer Algorithms and Programming
更多查看译文
关键词
deep learning,drug discovery,generative models,graph neural networks
AI 理解论文
溯源树
样例
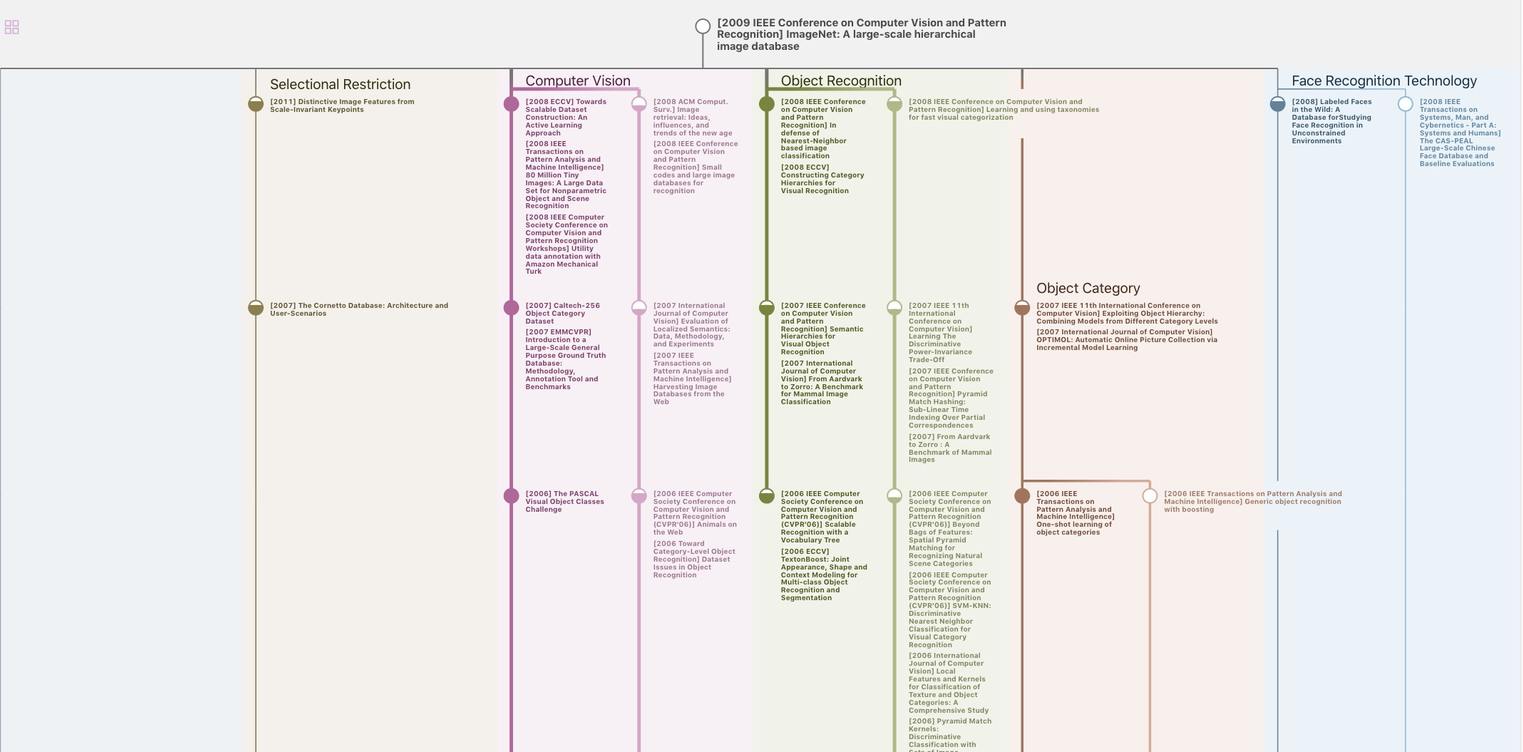
生成溯源树,研究论文发展脉络
Chat Paper
正在生成论文摘要