Improvement of Computational Performance of Evolutionary AutoML in a Heterogeneous Environment
2023 IEEE Congress on Evolutionary Computation (CEC)(2023)
摘要
Resource-intensive computations are a major factor that limits the effectiveness of automated machine learning solutions. In the paper, we propose a modular approach that can be used to increase the quality of evolutionary optimization for modelling pipelines with a graph-based structure. It consists of several stages - parallelization, caching and evaluation. Heterogeneous and remote resources can be involved in the evaluation stage. The conducted experiments confirm the correctness and effectiveness of the proposed approach. The implemented algorithms are available as a part of the open-source framework FEDOT.
更多查看译文
关键词
AutoML,heterogeneous infrastructure,evolutionary optimization,caching
AI 理解论文
溯源树
样例
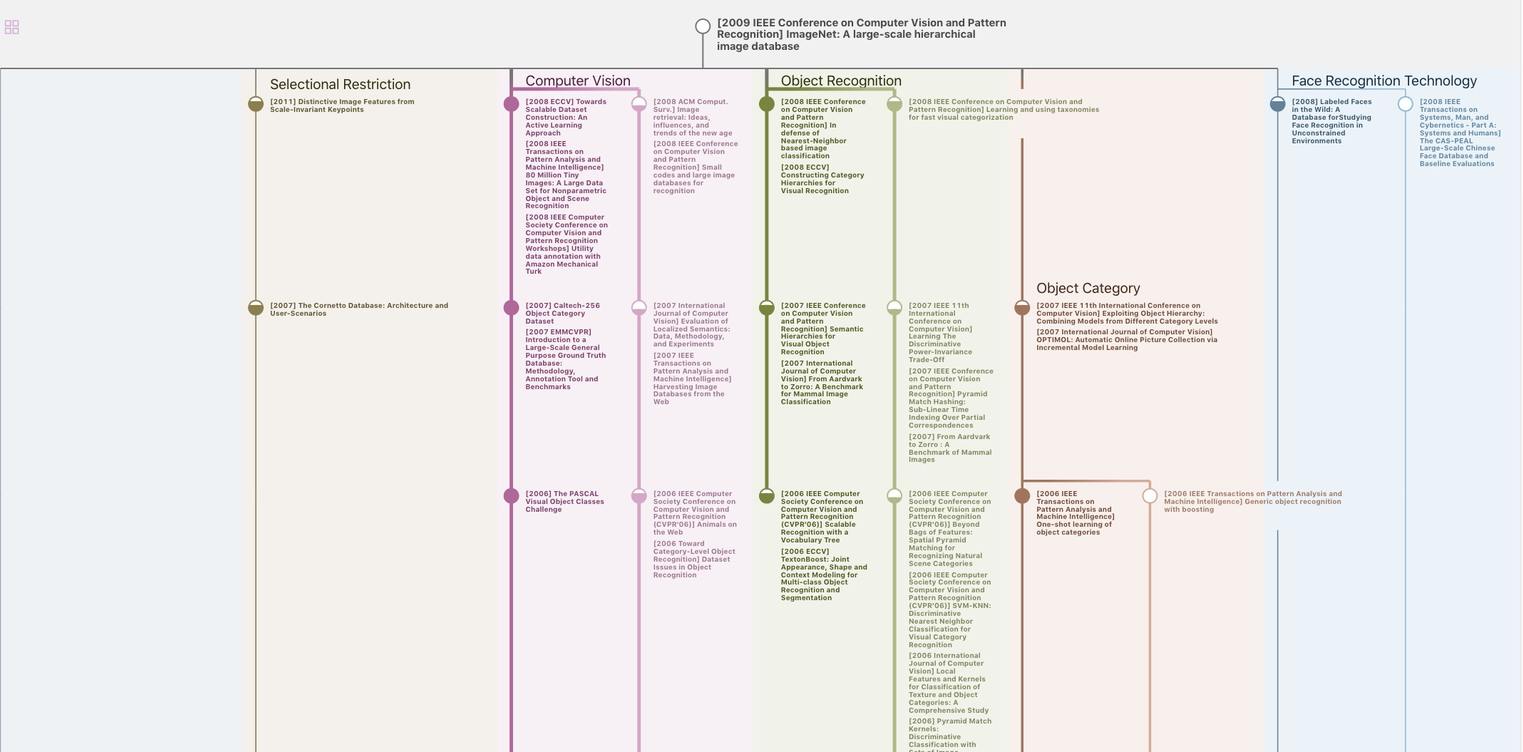
生成溯源树,研究论文发展脉络
Chat Paper
正在生成论文摘要