Anomalies, Representations, and Self-Supervision
arXiv (Cornell University)(2023)
摘要
We develop a self-supervised method for density-based anomaly detection using contrastive learning, and test it using event-level anomaly data from CMS ADC2021. The AnomalyCLR technique is data-driven and uses augmentations of the background data to mimic non-Standard-Model events in a model-agnostic way. It uses a permutation-invariant Transformer Encoder architecture to map the objects measured in a collider event to the representation space, where the data augmentations define a representation space which is sensitive to potential anomalous features. An AutoEncoder trained on background representations then computes anomaly scores for a variety of signals in the representation space. With AnomalyCLR we find significant improvements on performance metrics for all signals when compared to the raw data baseline.
更多查看译文
关键词
anomalies,representations,self-supervision
AI 理解论文
溯源树
样例
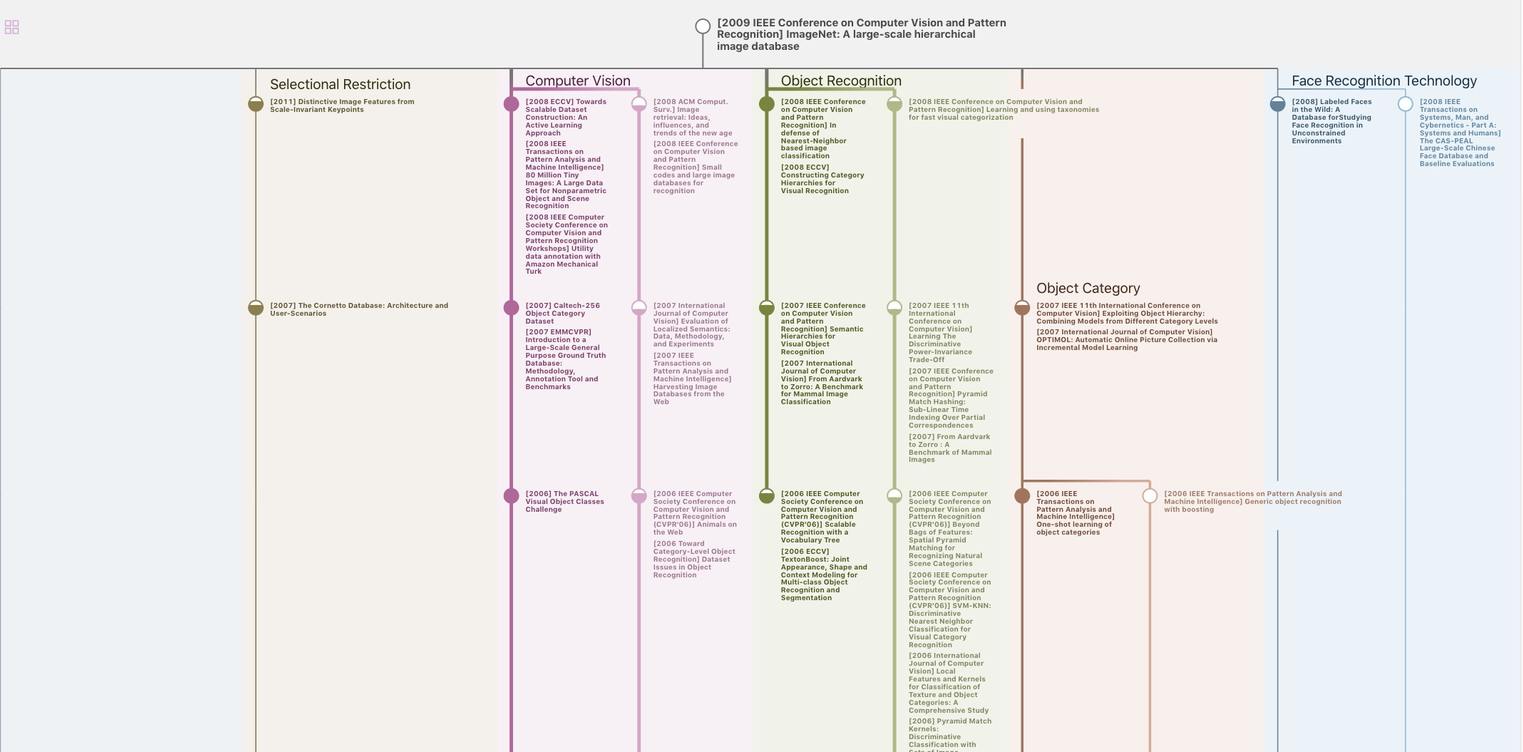
生成溯源树,研究论文发展脉络
Chat Paper
正在生成论文摘要