Deconfounded Dimension Reduction via Partial Embeddings
biorxiv(2023)
摘要
Dimension reduction tools preserving similarity and graph structure such as t -SNE and UMAP can capture complex biological patterns in high-dimensional data. However, these tools typically are not designed to separate effects of interest from unwanted effects due to confounders. We introduce the partial embedding (PARE) framework, which enables removal of confounders from any distance-based dimension reduction method. We then develop partial t -SNE and partial UMAP and apply these methods to genomic and neuroimaging data. Our results show that the PARE framework can remove batch effects in single-cell sequencing data as well as separate clinical and technical variability in neuroimaging measures. We demonstrate that the PARE framework extends dimension reduction methods to highlight biological patterns of interest while effectively removing confounding effects.
### Competing Interest Statement
RB has received consulting fees from Bristol-Myers Squibb and EMD Serono and research support from Bristol-Myers Squibb, EMD Serono, and Novartis. DSR has received research funding from Abata Therapeutics, Sanofi-Genzyme, and Vertex Pharmaceuticals, all unrelated to the current study. RTS receives consulting income from Octave Bioscience and compensation for scientific reviewing from the American Medical Association.
更多查看译文
关键词
partial embeddings,dimension reduction
AI 理解论文
溯源树
样例
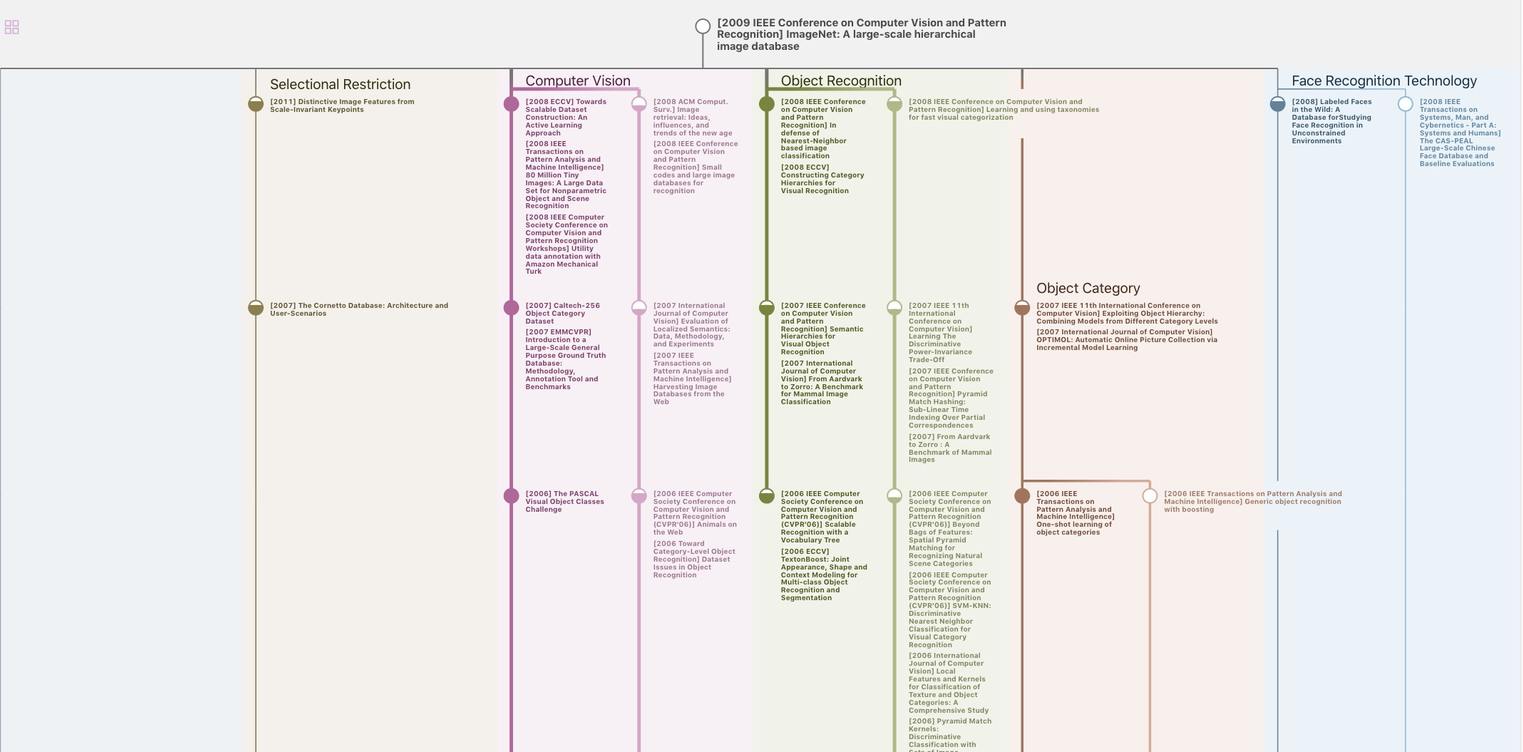
生成溯源树,研究论文发展脉络
Chat Paper
正在生成论文摘要