Perceptually Motivated Generative Model for Magnetic Resonance Image Denoising
JOURNAL OF DIGITAL IMAGING(2023)
摘要
Image denoising is an important preprocessing step in low-level vision problems involving biomedical images. Noise removal techniques can greatly benefit raw corrupted magnetic resonance images (MRI). It has been discovered that the MR data is corrupted by a mixture of Gaussian-impulse noise caused by detector flaws and transmission errors. This paper proposes a deep generative model (GenMRIDenoiser) for dealing with this mixed noise scenario. This work makes four contributions. To begin, Wasserstein generative adversarial network (WGAN) is used in model training to mitigate the problem of vanishing gradient, mode collapse, and convergence issues encountered while training a vanilla GAN. Second, a perceptually motivated loss function is used to guide the training process in order to preserve the low-level details in the form of high-frequency components in the image. Third, batch renormalization is used between the convolutional and activation layers to prevent performance degradation under the assumption of non-independent and identically distributed (non-iid) data. Fourth, global feature attention module (GFAM) is appended at the beginning and end of the parallel ensemble blocks to capture the long-range dependencies that are often lost due to the small receptive field of convolutional filters. The experimental results over synthetic data and MRI stack obtained from real MR scanners indicate the potential utility of the proposed technique across a wide range of degradation scenarios.
更多查看译文
关键词
Magnetic resonance imaging,Image denoising,Gaussian-impulse noise,Perceptually motivated loss,Adversarial training,Attention block
AI 理解论文
溯源树
样例
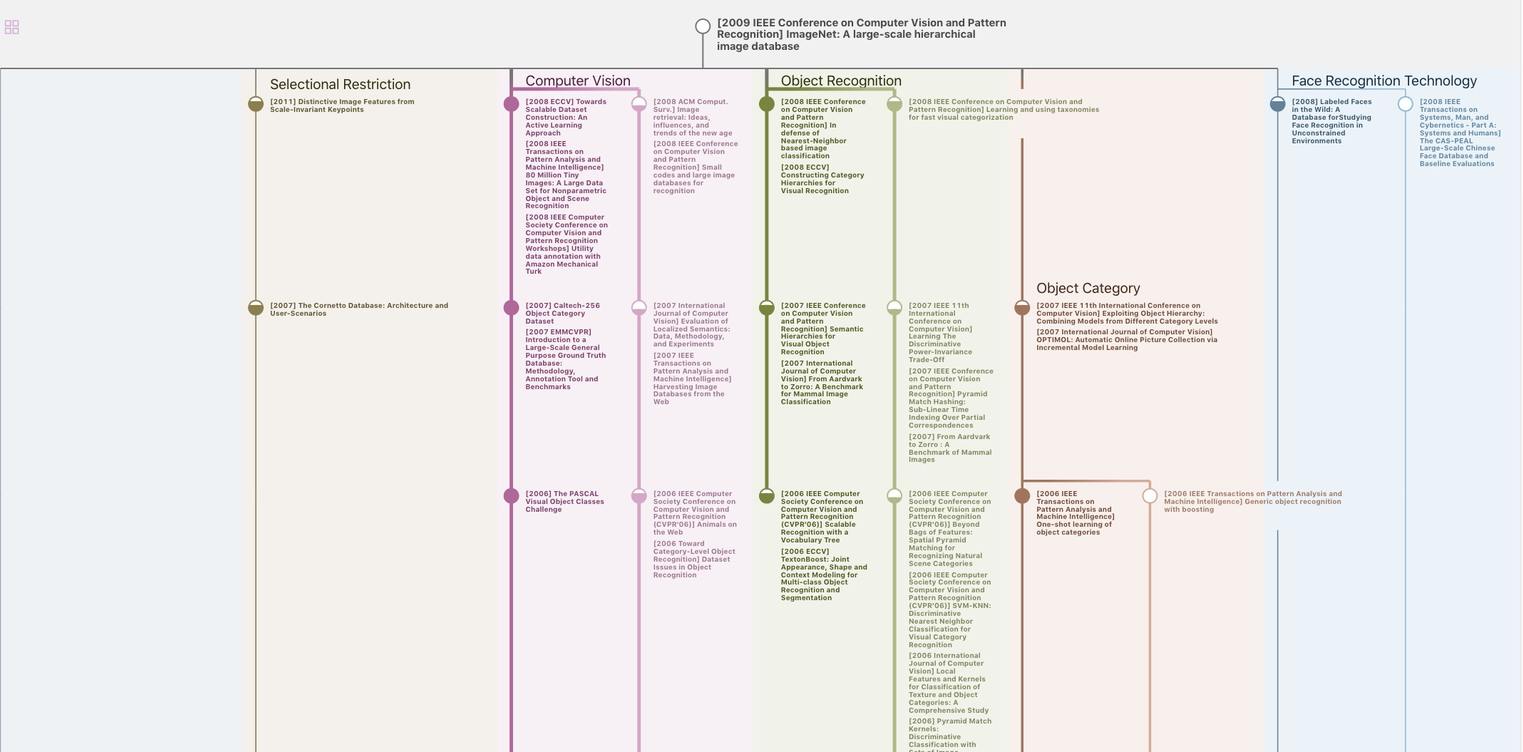
生成溯源树,研究论文发展脉络
Chat Paper
正在生成论文摘要