Stability Guaranteed Model Predictive Control With Adaptive Lyapunov Constraint
IEEE TRANSACTIONS ON AUTOMATION SCIENCE AND ENGINEERING(2024)
摘要
This paper aims to design a stabilized Adaptive Model Predictive Control (MPC) for nonlinear continuous systems with unknown dynamics at the start time. The proposed method is based on the Lyapunov MPC (LMPC) where the derivative of the Lyapunov function of the closed-loop system is limited to be less than that under an auxiliary Lyapunov controller. Different from the existing LMPC methods, this paper provides an online constraint updating strategy, which gradually reduces the conservation of the constraint. Specifically, the piece-wise linear (PWL) model updated by the Set-Membership (SM) identification method is adopted in the constraint and the auxiliary controller design, which can result in a non-increasing boundary of the model error. Then, a sufficient condition that guarantees the stabilization of the closed-loop system is deduced, which takes the effect of sample-and-hold implementation and the error boundary of the PWL model into account. By this condition, an optimization problem to update the Lyapunov controller for relaxing the constraints of MPC is proposed. We prove that, by the proposed method, the states will eventually converge to a small region around the equilibrium, both this small region and the conservatism of MPC decrease with the increasing of the accuracy of the PWL model. The application of the proposed method to a chemical process demonstrates the effectiveness of the proposed method.
更多查看译文
关键词
Adaptation models,Predictive models,Stability analysis,Uncertainty,Closed loop systems,Optimization,Predictive control,Model predictive control,Lyapunov technique,nonlinear system,adaptive control
AI 理解论文
溯源树
样例
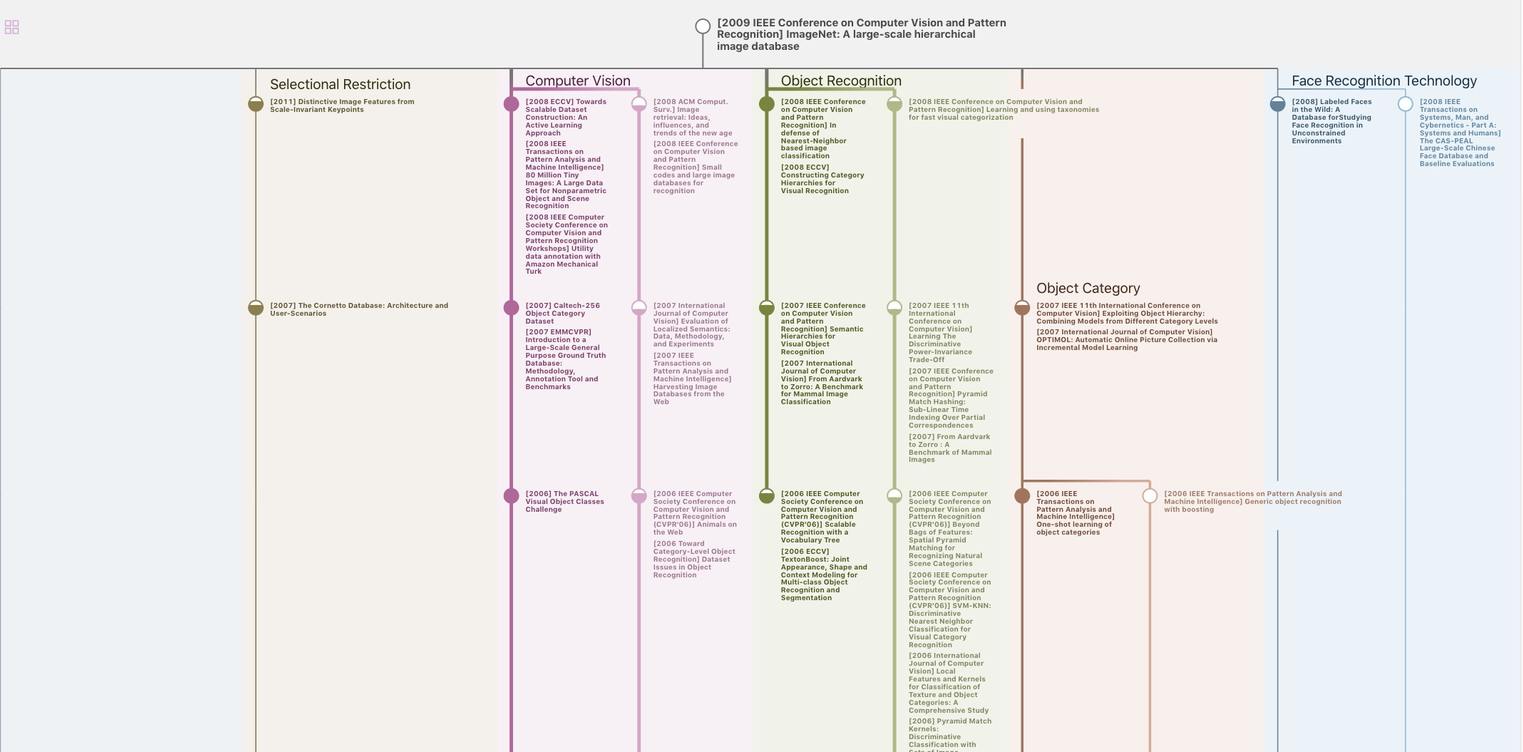
生成溯源树,研究论文发展脉络
Chat Paper
正在生成论文摘要