Optimization of Stamping Process Parameters Based on Improved GA-BP Neural Network Model
ENGINEERING OPTIMIZATION(2024)
关键词
Genetic algorithm,BP neural network,Stamping process parameters,Optimization
AI 理解论文
溯源树
样例
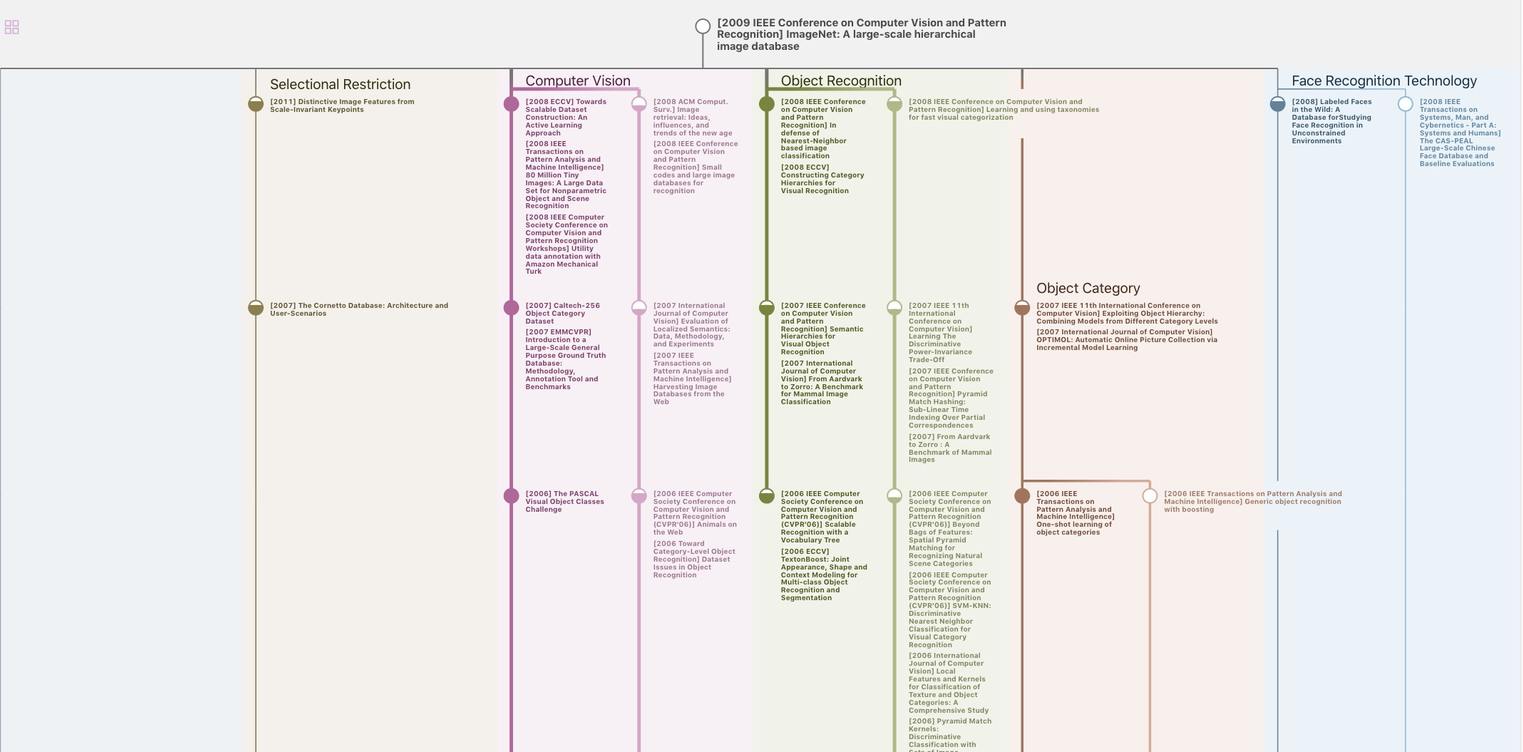
生成溯源树,研究论文发展脉络
Chat Paper
正在生成论文摘要