A local region proposals approach to instance segmentation for intestinal polyp detection
INTERNATIONAL JOURNAL OF MACHINE LEARNING AND CYBERNETICS(2022)
Abstract
This article designs a cascaded neural network to diagnose colonoscopic images automatically. With the limited number of labeled polyps masked in binary, the proposed detection network uses a hetero-encoder to map a colonoscopic image to an aggregated set of exemplified images as data argumentation to force the successive autoencoder to learn important features acting as a denoising autoencoder. In other words, the autoencoder denoises the transient images generated in the precedent hetero-encoder training process by auto-associating the ground truth and its variants. A hard attention model classifies the segmented image and applies a local region proposal network (RPN) to the generation and aggression of bounding boxes only on the segmented images to allow a more precise detection such that computations on bounding boxes with less information are avoided. The proposed system can outperform current complex state-of-art methods like faster-R-CNN from the experiments on endoscopic images.
MoreTranslated text
Key words
Image segmentation,Convolution neural network,Region proposal network,Polyps detection
AI Read Science
Must-Reading Tree
Example
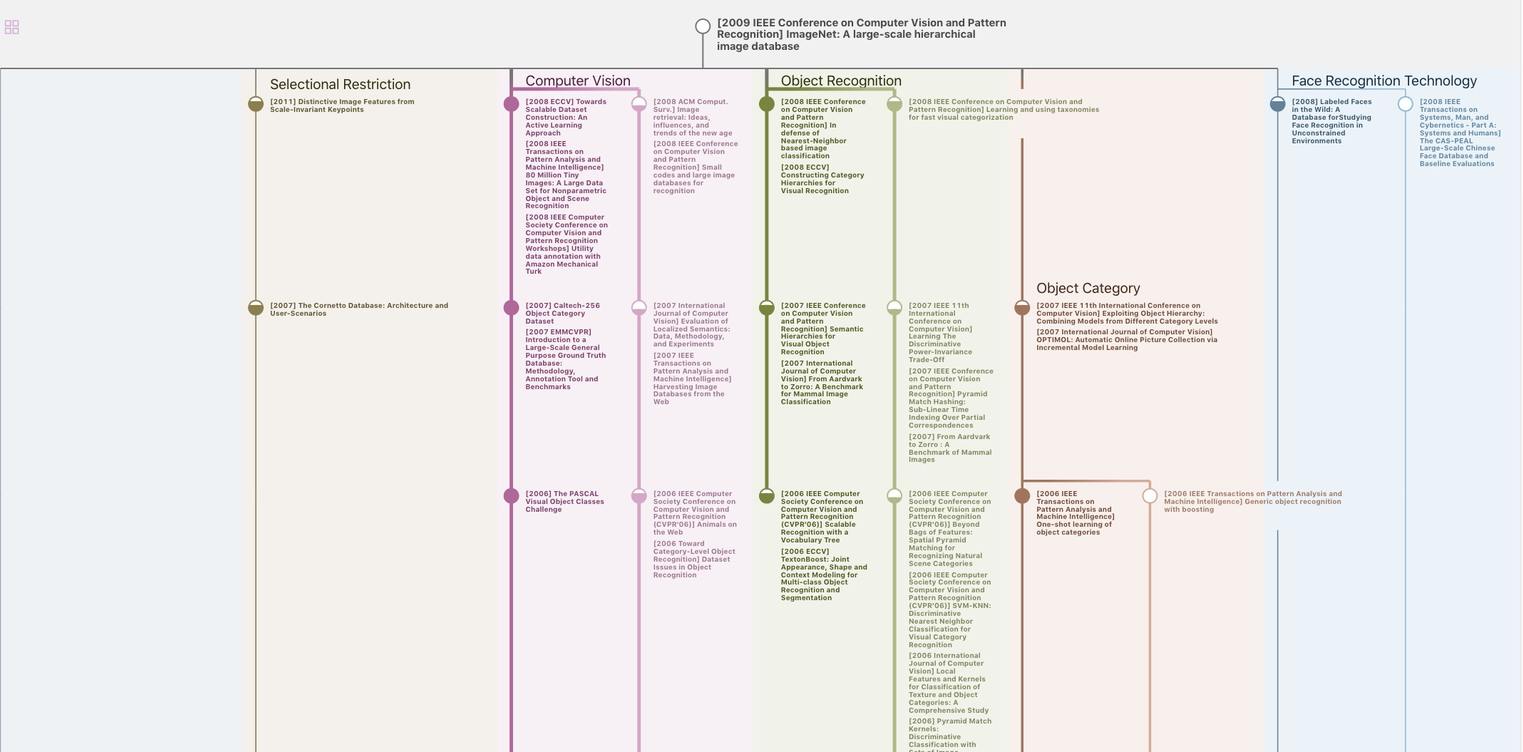
Generate MRT to find the research sequence of this paper
Chat Paper
Summary is being generated by the instructions you defined