Object Detection and Image Segmentation for Autonomous Vehicles
2021 IEEE 9TH INTERNATIONAL CONFERENCE ON INFORMATION, COMMUNICATION AND NETWORKS (ICICN 2021)(2021)
Abstract
With the rapid development of edge computing and the demand for green, safe and efficient transportation system, edge intelligence has been widely used in various traffic scenarios. By collecting images and videos, vehicles can obtain basic data and traffic flow information, which can be used to predict future movement trends. In addition, different traffic participants and their surroundings can be distinguished by image segmentation technology. In this paper, considering the resource limitation and latency constraint on edge vehicles, we proposed an improved vehicle detection algorithm based on tailored YOLOv4(You Only Look Once). To further increase the detection accuracy and speed, we introduce the Efficient Channel Attention (ECA) mechanism and High-Resolution Network (HRNet) into improved YOLOv4. After that, based on collected and detected objects, we proposed an image segmentation algorithm based on the DeepLabv3+ network, in which the MobileNetv2 is taken as the backbone network and the Softpool pooling algorithm is adopted as the pooling method. Experimental results show that compared with other classic methods, our proposed model has a higher mean Average Precision (mAP) for object detection and can improve the accuracy of original YOLOv4 from 83.34% to 87.64%. For image segmentation, our model also outperform other models with the Mean Intersection over Union (mIOU) improved from 72.18% to 74.99%.
MoreTranslated text
Key words
object detection, image segmentation, edge intelligence, power constrain network, attention mechanism
AI Read Science
Must-Reading Tree
Example
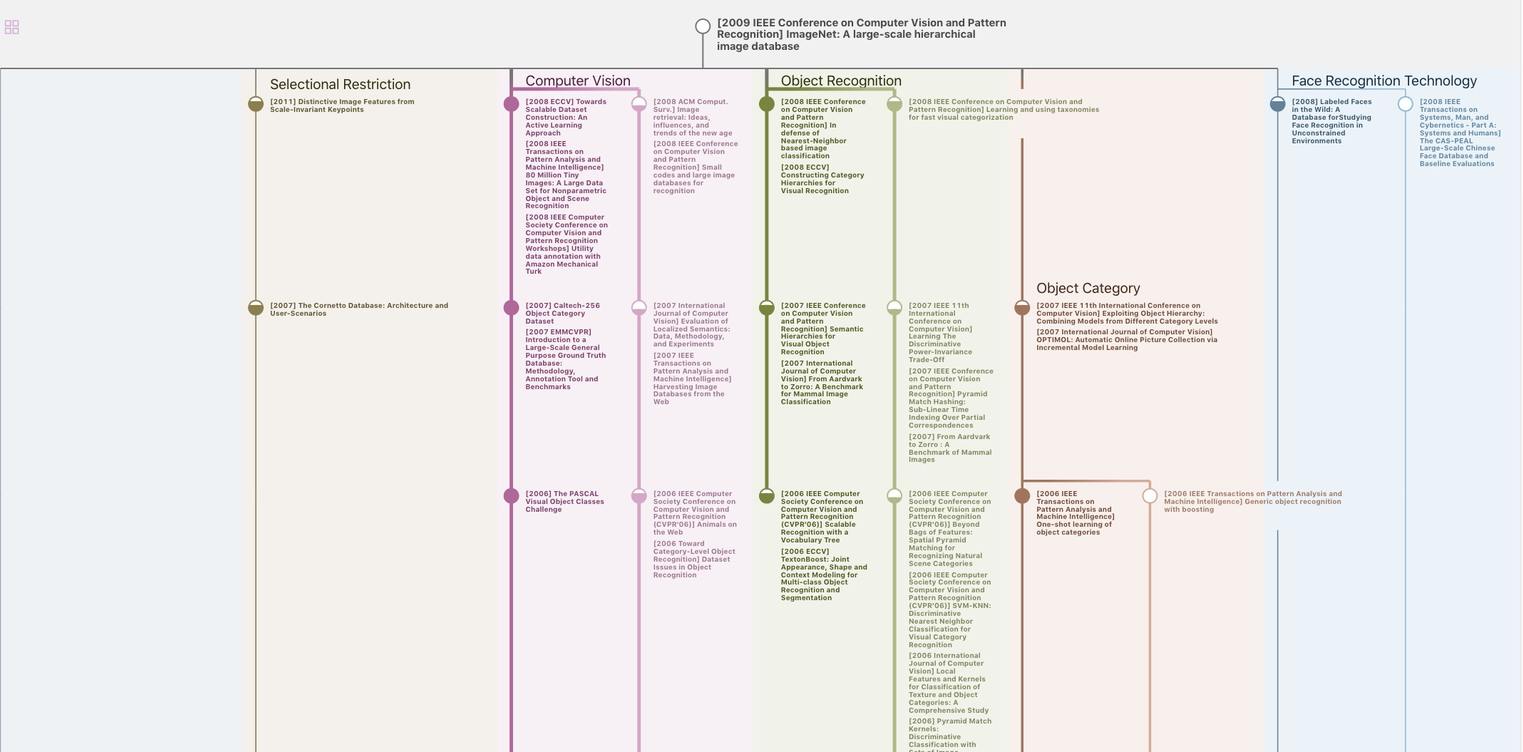
Generate MRT to find the research sequence of this paper
Chat Paper
Summary is being generated by the instructions you defined