Structural optimization on the heating shell of a quartz crystal microbalance space atmospheric density sensor based on the self-directed online machine learning optimization approach
International Communications in Heat and Mass Transfer(2023)
摘要
In this study, a numerical model of quartz crystal microbalance (QCM) of a space atmospheric density sensor is developed. To reduce the heating time of desorption, the two-section linear and nonlinear radiation surfaces are proposed to optimize the heat transfer characteristics of the heating shell based on the self-directed online machine learning optimization (SOLO) approach. SOLO approach integrates deep neural network (DNN) with the finite elements method (FEM), which substitutes the objective as a function of design variables. New training data is generated dynamically based on the DNN's prediction of the shortest heating time of QCM space atmospheric density sensor. For the optimal two-section radiation surface, H1, L1 (height and horizontal span of first section radiation surface) are selected as the variables to be optimized. The optimal design values are 4.32 mm and 2.53 mm, respectively, the corresponding heating time is about 99.8 s, which approximately reduces 15% compared with the original structure. For the nonlinear radiation surface, the generatrix of the first section follows an exponential function a(ebx-1), the second section follows a logarithmic function cln(dx + 1), the distance between the top of heating shell and the boundary point of the two section is denoted as Hn. a, b, c, d and Hn are selected as the variables to be optimized, the optimal design values are 1.84, 0.79, 1.72, 45.99 and 0.5 mm, respectively, the corresponding heating time is about 97.7 s, which approximately reduces 18.6% compared with the original structure.
更多查看译文
关键词
QCM sensor,Heating shell,Self-directed online learning,Optimization
AI 理解论文
溯源树
样例
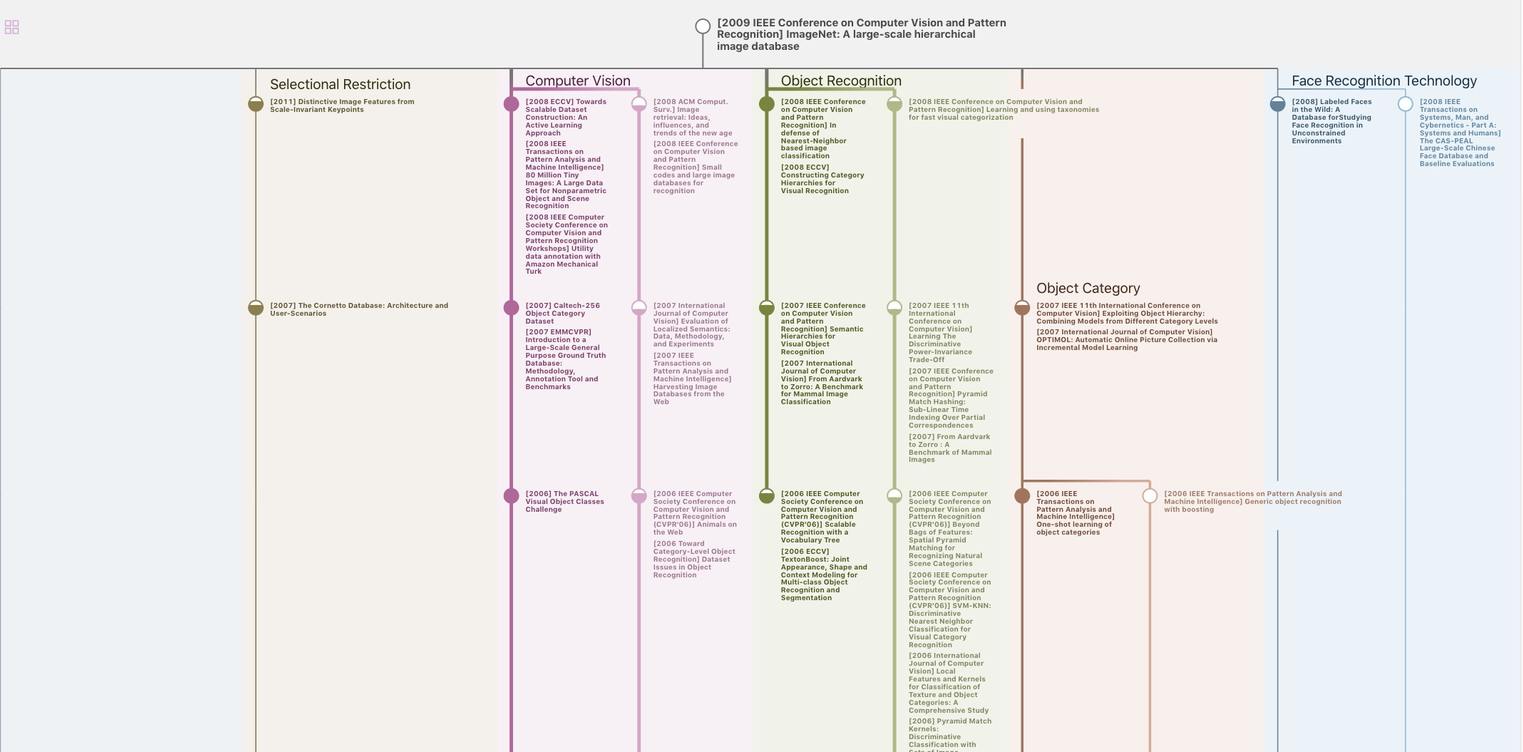
生成溯源树,研究论文发展脉络
Chat Paper
正在生成论文摘要