Transformer-Based Unsupervised Cross-Sensor Domain Adaptation for Electromechanical Actuator Fault Diagnosis
Machines(2023)
摘要
There have been some successful attempts to develop data-driven fault diagnostic methods in recent years. A common assumption in most studies is that the data of the source and target domains are obtained from the same sensor. Nevertheless, because electromechanical actuators may have complex motion trajectories and mechanical structures, it may not always be possible to acquire the data from a particular sensor position. When the sensor locations of electromechanical actuators are changed, the fault diagnosis problem becomes further complicated because the feature space is significantly distorted. The literature on this subject is relatively underdeveloped despite its critical importance. This paper introduces a Transformer-based end-to-end cross-sensor domain fault diagnosis method for electromechanical actuators to overcome these obstacles. An enhanced Transformer model is developed to obtain domain-stable features at various sensor locations. A convolutional embedding method is also proposed to improve the model's ability to integrate local contextual information. Further, the joint distribution discrepancy between two sensor domains is minimized by using Joint Maximum Mean Discrepancy. Finally, the proposed method is validated using an electromechanical actuator dataset. Twenty-four transfer tasks are designed to validate cross-sensor domain adaptation fault diagnosis problems, covering all combinations of three sensor locations under different operating conditions. According to the results, the proposed method significantly outperforms the comparative method in terms of varying sensor locations.
更多查看译文
关键词
electro-mechanical actuators,Transformer,cross-sensor,fault diagnosis
AI 理解论文
溯源树
样例
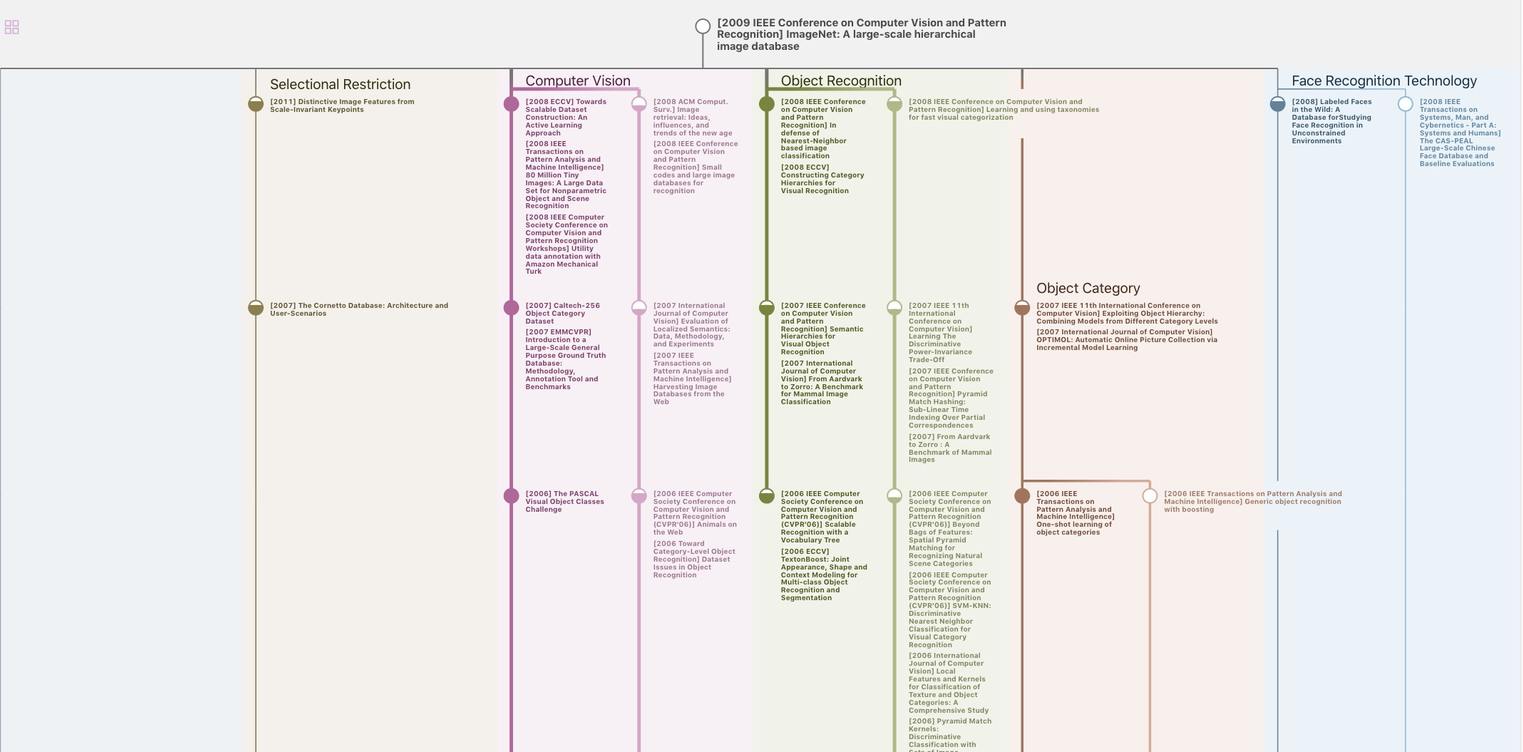
生成溯源树,研究论文发展脉络
Chat Paper
正在生成论文摘要