Generalizing p -Laplacian: spectral hypergraph theory and a partitioning algorithm
MACHINE LEARNING(2022)
Abstract
For hypergraph clustering, various methods have been proposed to define hypergraph p -Laplacians in the literature. This work proposes a general framework for an abstract class of hypergraph p -Laplacians from a differential-geometric view. This class includes previously proposed hypergraph p -Laplacians and also includes previously unstudied novel generalizations. For this abstract class, we extend current spectral theory by providing an extension of nodal domain theory for the eigenvectors of our hypergraph p -Laplacian. We use this nodal domain theory to provide bounds on the eigenvalues via a higher-order Cheeger inequality. Following our extension of spectral theory, we propose a novel hypergraph partitioning algorithm for our generalized p -Laplacian. Our empirical study shows that our algorithm outperforms spectral methods based on existing p -Laplacians.
MoreTranslated text
Key words
Spectral clustering,Hypergraph learning,Hypergraph p -Laplacian,Cheeger inequality
AI Read Science
Must-Reading Tree
Example
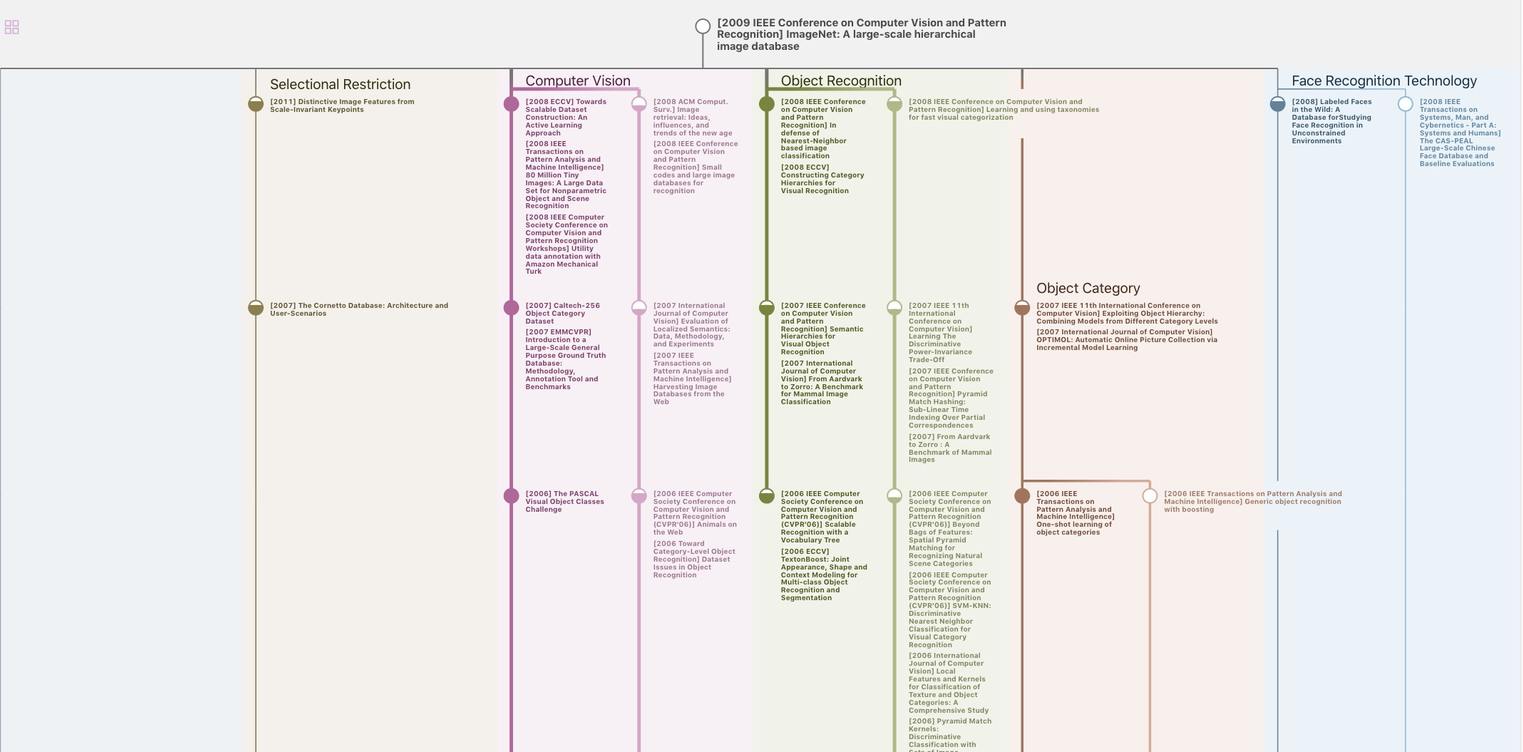
Generate MRT to find the research sequence of this paper
Chat Paper
Summary is being generated by the instructions you defined