Autonomous trajectory planning method for hypersonic vehicles in glide phase based on DDPG algorithm
PROCEEDINGS OF THE INSTITUTION OF MECHANICAL ENGINEERS PART G-JOURNAL OF AEROSPACE ENGINEERING(2022)
摘要
An autonomous optimal trajectory planning method based on the deep deterministic policy gradient (DDPG) algorithm of reinforcement learning (RL) for hypersonic vehicles (HV) is proposed in this paper. First, the trajectory planning problem is converted into a Markov Decision Process (MDP), and the amplitude of the bank angle is designated as the control input. The reward function of the MDP is set to minimize the trajectory terminal position errors with satisfying hard constraints. The deep neural network (DNN) is used to approximate the policy function and action-value function in the DDPG framework. The Actor network then computes the control input directly according to flight states. Using a limited exploration strategy, the optimal policy network would be considered fully trained when the reward value reached maximum convergence. Simulation results show that the policy network trained using a DDPG algorithm accomplishes 3-dimensional (3D) trajectory planning during the HV glide phase with high terminal precision and stable convergence. Additionally, the single step calculation time of the policy network occurs in near real time, which suggests great potential as an autonomous online trajectory planner. Monte Carlo experiments prove the strong robustness of the implementation of an autonomous trajectory planner under aerodynamic disturbances.
更多查看译文
关键词
deep reinforcement learning,DDPG algorithm,hypersonic vehicle,3D trajectory planning,glide phase
AI 理解论文
溯源树
样例
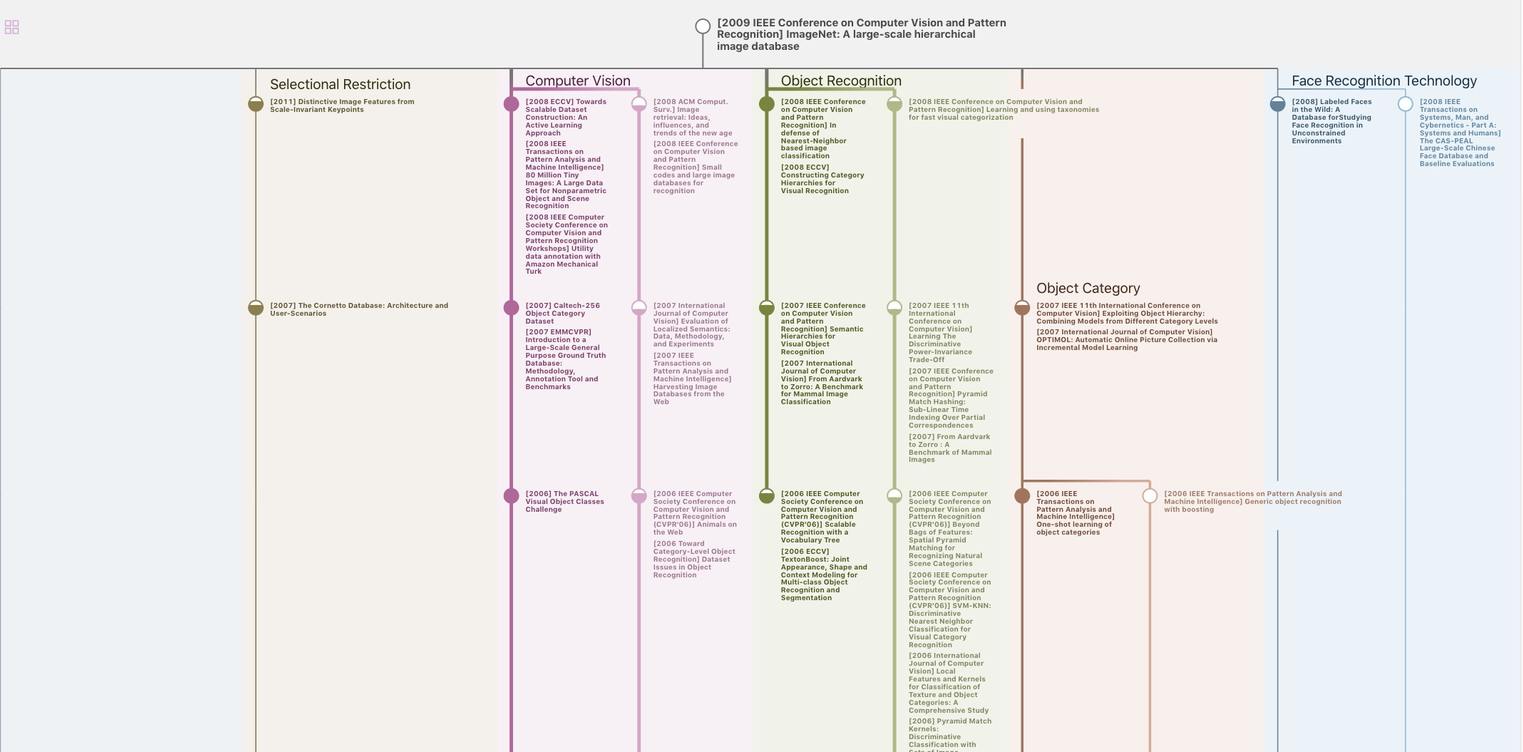
生成溯源树,研究论文发展脉络
Chat Paper
正在生成论文摘要