Fault Diagnosis Method for Bearing Based on Digital Twin
MATHEMATICAL PROBLEMS IN ENGINEERING(2022)
Abstract
The bearing is an essential component of rotating machinery, as its reliability and running state have a direct impact on the machinery's performance. Considering that deep learning-based fault diagnosis methods for bearing require a large amount of labelled sample data, a novel fault diagnosis framework based on digital twin is proposed. In the case of fault data available, self-organizing maps with minimum quantization error and support vector machine are employed to analyze the data. Where fault data is unavailable, a bearing digital twin model is first constructed to simulate the data, and the convolutional neural network combined with transfer learning is utilized to diagnose the bearing faults. Then, the law of bearing performance degradation is investigated. The effectiveness of the proposed method is verified using bearing vibration data.
MoreTranslated text
AI Read Science
Must-Reading Tree
Example
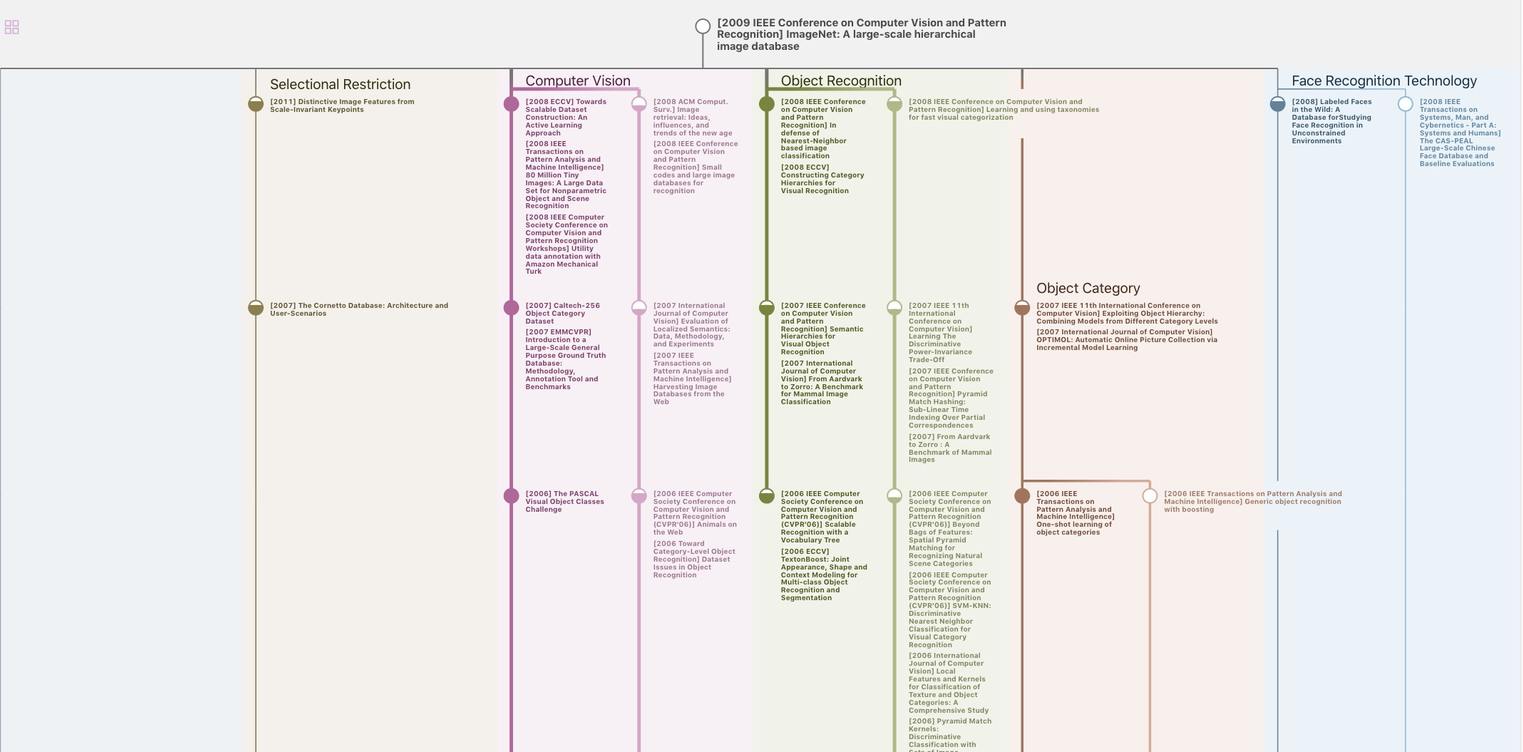
Generate MRT to find the research sequence of this paper
Chat Paper
Summary is being generated by the instructions you defined