Bridging Chemical Knowledge and Machine Learning for Performance Prediction of Organic Synthesis
CHEMISTRY-A EUROPEAN JOURNAL(2023)
摘要
Recent years have witnessed a boom of machine learning (ML) applications in chemistry, which reveals the potential of data-driven prediction of synthesis performance. Digitalization and ML modelling are the key strategies to fully exploit the unique potential within the synergistic interplay between experimental data and the robust prediction of performance and selectivity. A series of exciting studies have demonstrated the importance of chemical knowledge implementation in ML, which improves the model's capability for making predictions that are challenging and often go beyond the abilities of human beings. This Minireview summarizes the cutting-edge embedding techniques and model designs in synthetic performance prediction, elaborating how chemical knowledge can be incorporated into machine learning until June 2022. By merging organic synthesis tactics and chemical informatics, we hope this Review can provide a guide map and intrigue chemists to revisit the digitalization and computerization of organic chemistry principles.
更多查看译文
关键词
machine learning,molecular embedding,organic synthesis,performance prediction,synthetic dataset
AI 理解论文
溯源树
样例
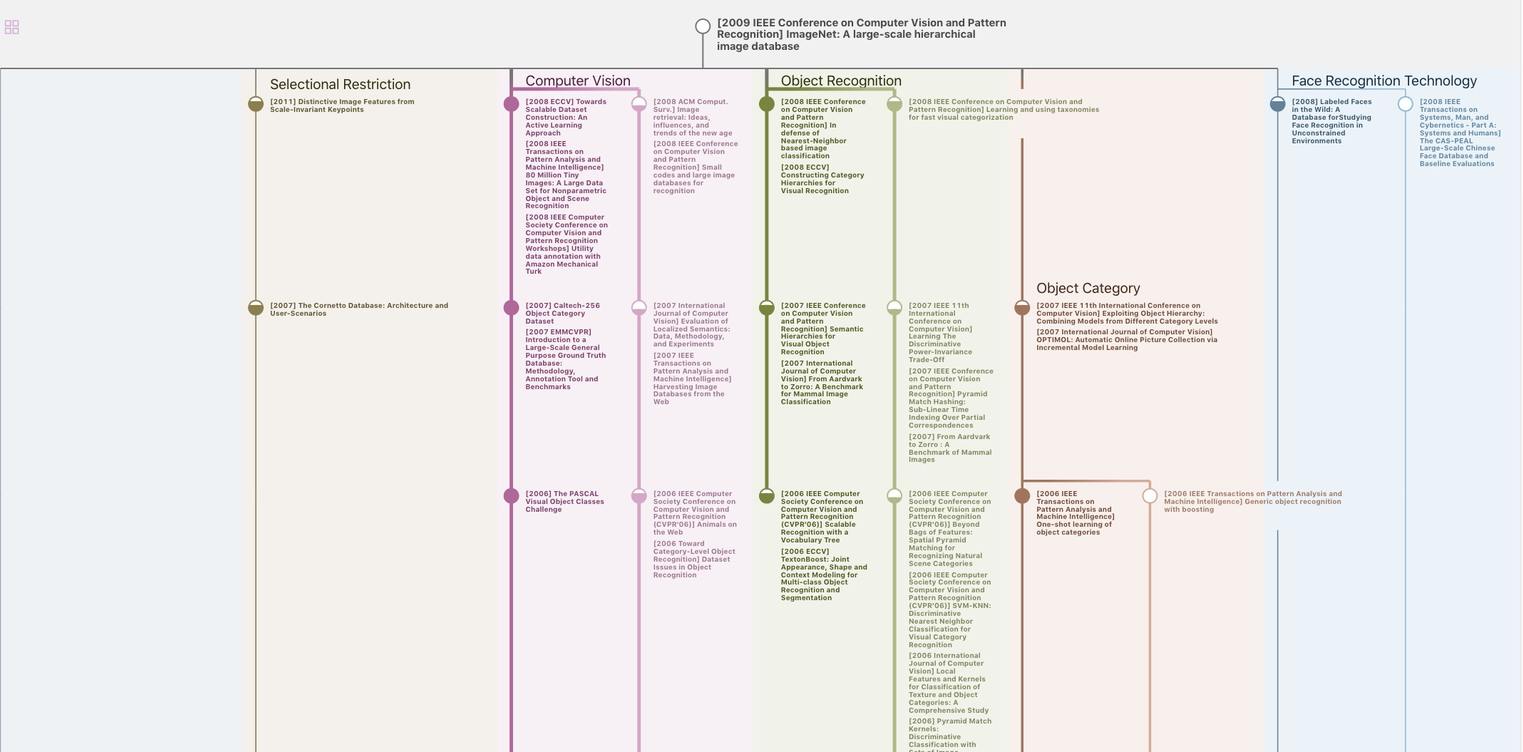
生成溯源树,研究论文发展脉络
Chat Paper
正在生成论文摘要