Siam-GCAN: A Siamese Graph Convolutional Attention Network for EEG Emotion Recognition
IEEE TRANSACTIONS ON INSTRUMENTATION AND MEASUREMENT(2022)
摘要
The graph convolutional network (GCN) shows effective performance in electroencephalogram (EEG) emotion recognition owing to the ability to capture brain connectivity. However, the depth information cannot be extracted only through the GCN structure, and the learning process of the GCN model ignores the intraclass and the interclass information. Regarding the above problems, we propose a Siamese graph convolutional attention network, named Siam-GCAN, which mainly considers the following two aspects: on the one hand, we use a deep attention layer implemented by a multihead attention mechanism to abstract deeper and valuable features rather than stacking graph convolution layers. On the other hand, we employ the Siamese network to cluster the outputs of GCNs based on Euclidean distance to ensure the learned information has a certain class separability. Experimental results on two public emotional datasets, the Shanghai Jiao Tong University (SJTU) emotion EEG dataset and the SJTU emotion EEG dataset-IV, demonstrate that Siam-GCAN outperforms the state-of-the-art baselines in EEG emotion recognition.
更多查看译文
关键词
Attention mechanism, electroencephalogram (EEG), emotion recognition, graph convolutional network (GCN), Siamese network
AI 理解论文
溯源树
样例
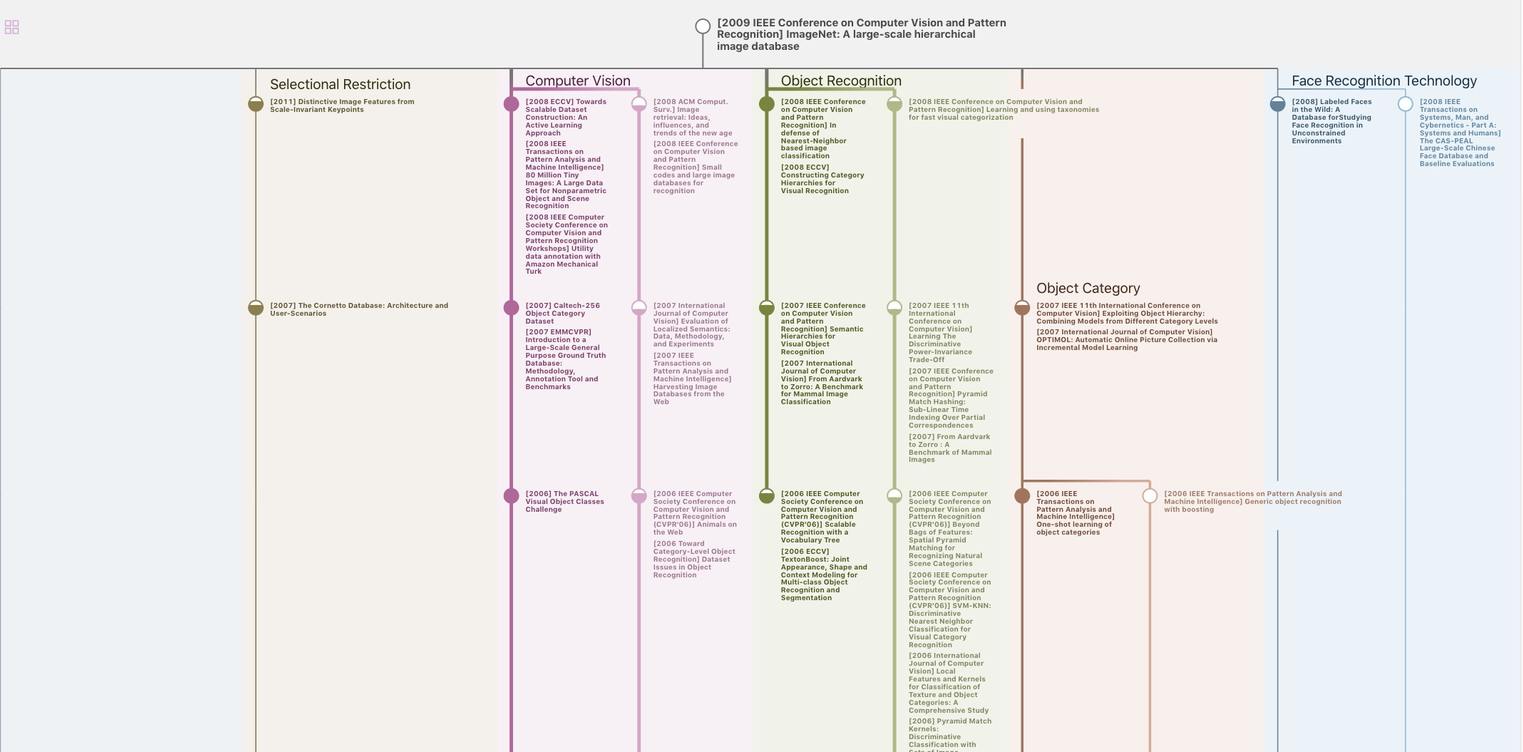
生成溯源树,研究论文发展脉络
Chat Paper
正在生成论文摘要